Automatic Table Recognition and Extraction from Heterogeneous Documents ()
Received 17 November 2015; accepted 27 December 2015; published 30 December 2015

1. Introduction
A table is made up of series of rows and columns. It is a cell that houses information on a table, the information can be alphabetic, numeric or alphanumeric; a table can also house images depending on what the user wants to use the table for. In sciences, tables are used to summarize a topic or a statement, also, in accounts; tables are used to know the money spent, the money at hand and the money to be spent. So, a table can be referred to as a set of facts or figures arranged in lines or columns.
A table as a list of facts or numbers arranged in a special order, usually in rows and columns and as an arrangement of numbers, words or items of any kind, in a definite and compact form, so as to exhibit some set of facts or relations in a distinct and comprehensive way, for convenience of study, reference, or calculation.
According to [1] , tables are visual oriented arrangements of information widely used in many different domains as a way to present and communicate complex information to human readers. Tables are used in all facets of lifelike science, engineering and agriculture among others. Tables appear in print media, handwritten notes, computer software, architectural ornamentation, traffic signs and many other places and have been described as databases designed for human eyes [2] . Tables appear in the earliest writing on clay tablets, and in the most modern Web pages. Some make use of line-art, others rely on whitespace only. The precise conventions and terminology for describing tables varies depending on the context. Further, tables differ significantly in variety, structure, flexibility, notation, representation and use. However, tables have many different layouts and are mostly contained in semi-structured and unstructured documents having various internal encodings such as HTML, PDF and flat text. Table recognition is concerned about detection of all lines, both vertical and horizontal which make up a table, along with their intersection, which will aid not only to detect a table which consequently can be extracted out of a whole document but also to describe both the physical and logical structure, thus, inferring a table recognition process [3] . While it is easy for human beings to recognise and understand tables, it is difficult for computers, because tables do not have any identifying characteristic in common (because tables do not have the same identifying characteristic) [4] .
Table Extraction (TE) is the task of detecting and decomposing table information in a document [5] . Automatically extracting information contained in tables and storing them in structured machine-readable (usable) form is of paramount importance in many application fields. Table recognition and extraction is a very challenging problem that poses many issues to researchers and practitioners in defining effective approaches.
Among many inspirational works is the work of [6] who designed an automatic table metadata extraction algorithm which was tested on PDF and that of [7] that extracted metadata from heterogeneous references using hidden Markov model and performed smoothing for transition probabilities.
2. Related Works
Many researchers have used HMM to extract one thing or the other from documents. Ojokoh et al. [7] used a full second-order HMM to extract metadata from heterogeneous references. Ana Costa e Silva [8] experimented with different ways of combining the components of an HMM for document analysis applications, in particular for finding tables in text. Pinto et al. (2003) in their own work presented the use of conditional random fields (CRFs) for table extraction, and compared them with hidden Markov models (HMMs). One of the pioneering works on table detection and recognition was done by [9] . Kieninger et al. [9] developed a table spotting and structure extraction system called T-Recs. The system relies on word bounding boxes as input. These word boxes are clustered with a bottom-up approach into regions by building a “segmentation graph”. These regions are then designated as candidate table regions if they satisfy certain criterion. The key limitation of the approach is that, based only on word boxes, multi-column layouts cannot be handled very accurately, so, it works well for only single column pages.
Oro et al. [1] automatically extracted information contained in tables and stored them in structured machine- readable form. Dalvi et al. [10] described an open-domain information extraction method for extracting concept-instance pairs from an HTML corpus. The method used relies on a novel approach for clustering terms- found in HTML tables, and then assigning concept names to these clusters using Hearst patterns. The method was efficiently applied to a large corpus, and experimental results on several datasets showed that the method can accurately extract large numbers of concept-instance pairs, the problem of automatically harvesting concept-instance pairs from a large corpus of HTML tables was considered.
Tengli et al. [11] extracted table automatically from web pages of semi-structured HTML tables, learns lexical variants from various examples but uses vector space model to deal with non-exact matches and labels. They defined their own evaluation methodology because they did not encounter any experimental evaluation of table extraction that can be used as reference of comparison with their work. Sale et al. [12] focused on extracting tables from large-scale HTML texts, analysed the structural aspects of a web table within which they devised the rules to process and extract attribute-value pairs from the table. Heuristic rules were employed to identify the tables. Also, a table interpretation algorithm was proposed which captures the attribute-value relationship among table cells. Yildiz et al. [4] developed several heuristics which together recognise and decompose tables in PDF files and store the extracted data in a structured data format (XML) for easier reuse. A prototype was also implemented which gives the user the ability of making adjustments on the extracted data. The work shows that purely heuristic-based approaches can achieve good results, especially for lucid tables. Wang and Hu [13] considered the problem of table detection in web documents and described a machine learning based approach to classify each given table entity as either genuine or non-genuine. Gatos et al. [3] proposed a novel technique for automatic table detection in document images, they did not use either training phase or domain-specific heuristics but used an approach which applied to a variety of document types, that is, used a particular parameterization that depends on the average character height of the document image. Their proposed approach builds upon several consequent stages such as image preprocessing; horizontal and vertical line detection (horizontal and vertical line estimation and (ii) line estimation improvement by using image/text areas removal.); and table detection (Detection of line intersections and (ii) table detection-reconstruction.). The efficiency of the proposed method is demonstrated by using a performance evaluation scheme which considers a great variety of documents such as forms, newspapers/magazines, scientific journals, tickets/bank cheques, certificates and handwritten documents.
HMM is one of the most popular models being used for sequential model, it is widely used because it is simple and easy enough that one can actually estimate the pre-eminence, it is also rich enough that it can handle real world applications. HMM is a doubly stochastic process with an underlying stochastic process that is not observable (it is hidden), but can only be observed through another set of stochastic processes that produce the sequence of observed symbols [14] .
Information can be extracted using standard approaches of hand-written regular expressions (perhaps stacked), using classifiers (like generative: naïve Bayes classifier, discriminative: maximum entropy models), sequence models (like Hidden Markov model, Conditional Markov model (CMM)/Maximum-entropy Markov model (MEMM), Conditional random fields (CRF) (are commonly used in conjunction with IE for tasks as varied as extracting information from research papers to extracting navigation instructions). Hybrid approaches can also be used for IE which is the combination of some of the standard approaches listed above but HMM was used in this research work.
This research work was therefore motivated by the need to recognize and extract tables from a large collection of documents of different types and structures and it was able to achieve its aim upon converting the different faces of the documents to similar faces using document converter.
It has been shown in this work that HMM is a fast learner even with small size data set and can also do very well with a large size data set since it is a machine learning model.
3. Hidden Markov Model
A Hidden Markov Model (HMM) is a finite state automation comprising of stochastic state transitions and symbol emissions. The automation models a probabilistic generative process whereby a sequence of symbols is produced by starting at a designated start state, transitioning to a new state, emitting one of a set of output symbols selected by that state, transitioning again, emitting another symbol, and so on, until a designated final state is reached. Associated with each of a set of states is a probability distribution over the symbols in the emission symbol and a probability distribution over its set of outgoing transitions [7] .
Expressing the model mathematically, we have the definitions in Equations (1) to (6)
The Hidden Markov Model is a five-tuple
,
(1)
S is the set of states,
(2)
is the probability of being from state i to state j (3)
where,
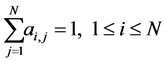
, indicating the probability of being at state Si at time t = 1 (4)
(5)
where M is the number of emission symbols in the discrete vocabulary, V.
, indicating the probability of observing symbol,
at state
, (6)
where,
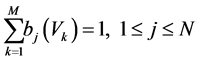
A table contains properties like td, tr, tbody, table, paragraph (that is table data, table row, table body, table, paragraph respectively). Each of these properties is a state generating sequence of observable/emission symbols during transition from one state to another. Given an HMM, each transition is performed by determining the sequence of states that is most likely to have generated the entire observable/emission symbol sequence. Viterbi algorithm is a common way of showing the most probable path of (for) the sequence [7] . After the Viterbi algorithm had shown the most probable path, the table extracted by the HMM was shown while its HTML version was generated with the help of an algorithm shown below:
1) For all the tables generated, pick a table (ti
. where n = number of tables) if they are not exhausted.
2) Display the fetched table (ti)
3) Generate the HTML (hi) for ti
4) For all the tags (
. where m = number of tags in tables
); fetch a tag.
5) Display the tag ![]()
6) Fetch the next token.
a) If it is not a tag but inner HTML, indent positively and display content.
b) If it is another tag indent positively (indent right) and display content.
c) If it is closing tag indent negatively and display content.
The tables extracted by the HMM were sometimes not the same as the original tables but may be different in either of the following:
1) the contents of two cells may merge into a single cell (that is, the content of cell B may be extracted into cell A leaving cell B empty and adding to the content of cell A and vice versa).
2) a cell may be missing,
3) a row may be missing as the case may be.
But in all, there is retention of about 90% to 98% of the original table.
4. System Architecture
The system architecture shown in Figure 1 describes the table recognition and extraction processes. The heterogeneous documents constitute the source document. These documents were pre-processed where necessary and parsed in order to extract tables that were embedded in them for further processing.
The design spans through all the tools used for pre-processing heterogeneous documents into HTML code, the generation of Transition Matrix, Initial Probability Matrix, Observable Symbol Matrix, Smoothing the Observable Symbol Matrix using Laplace Smoothing Method and Viterbi’s algorithm to determine the best path, and finally the extracted tables and contents.
4.1. The Training Phase
The data sets used in this research work were self-generated because there is no known standardised data set for table extraction. It contains five hundred and twenty six (526) tables in twenty-five (25) documents of Microsoft Word, Portable Document Format and Hypertext Markup Language. This set of documents is divided into two phases: The training phase and the testing phase. In document pre-processor, heterogeneous documents were converted to their HTML equivalence. The PDF is first converted to Word document using a PDF converter (PDFC) before being converted to its HTML equivalence.
![]()
Figure 1. Architecture for table recognition and extraction processes.
To build an HMM for table extraction in documents, one needs to first decide how many states the model will contain and what transitions between states should be allowed [7] . At the beginning of the training phase, the model was automatically learned from the training data. The model was first constructed to produce all the results for Transition Probability Matrix, Initial Probability Matrix and Observable/Emission Symbols/Words contained in the data sets, with the start state having a transition being represented by a unique path with one state per tag. Three hundred and twenty-one (321) tables were used. As the training data are coming from the training phase, they may be saved in the DBMS or may not be saved. This model consists of the result for Transition Probability Matrix, Initial Probability Matrix, Observable/Emission Symbol Matrix and the smoothened Observable/Emission Symbol Matrix to avoid a negative result at the end. Transition Probability Matrix like the name implies is a matrix of state transitions. It has the general mathematical representation as demonstrated.
Given:
where S is a matrix of states
where T is a matrix of state transitions
This is a system of n states.
For all
which is the probability of each state transition, the sum along all the rows is 1, and the transitions are non-negative. A transition probability that is zero (0) signifies an impossible transition from any state to that state. All probabilities cannot be greater than unity.
The case study has five states: TD, TH, TBody, P, Table while the algorithm for the transition probability is given below:
1) Get the object document from the CK Editor using get Data() API function
2) Determine which of the states of interest comes first
3) Find the probability of each of the states considering the number of documents used
4) Store this in a table.
5) Output the initial probability matrix.
Observable/emission symbols are the symbols extracted by the system.
In this research work, the following are the categories of observable symbols:
1) Numbers
2) Single words
3) Short statements
4) Long statements
5) Title case statements
6) Uppercase statements
The probability of the observable/emission symbols was computed and the algorithm computes the probability of the observable/emission symbols, stores its matrix and generates an output.
A description of the observable/emission symbols and samples from the data set is shown in Table 1.
4.2. Smoothing
Since the observable/emission symbol probability assigns zero probability to some unseen emissions, retaining these zeroes would affect the result negatively, and then there is a need for smoothing. There are so many methods used for smoothing, but in this research work, Laplace method was used. The maximum likelihood model was modified such that one (1) is placed as the numerator and m is added to the denominator [15] .
(7)
where Vk is the unseen symbol while transitioning from state i to j, m is the addition of the total number of sequence of states and the number of non-zero probability across row.
4.3. The Testing Phase
This phase contains two hundred and five (205) tables. This is used for the evaluation of the trained model.
4.3.1. The Viterbi Algorithm
After going through the processes of transitioning, transition probability, observable/emission probabilities, a trained Hidden Markov Model would have been formed. This model consisting of values for the transition and observable/emission probability matrices alongside with the sequence of symbols derived from the testing data sets will be passed to the Viterbi Algorithm which will produce a sequence of states (
) most likely to have produced the symbol sequence which represent the tokens in the table.
![]()
Table 1. Observable/emission symbols and examples.
The specific problem to be solved by Viterbi algorithm is to obtain the most possible state sequence,
for a given observation sequence,
. This can be obtained by computing the probabilities for all possible sequences and then obtaining the maximum probability as follows:
(8)
The Viterbi algorithm makes a number of assumptions. First, both the observed events and hidden events must be in a sequence. This sequence often corresponds to time. Second, these two sequence need to be aligned and an instance of an observed event needs to correspond to exactly one instance of a hidden event. Third, computing the most likely hidden sequence up to a certain point t must depend only on the observed event at point t, and the most likely sequence at point t-1. These assumptions listed above can be elaborated as follows. The Viterbi algorithm operates on a state machine assumption. That is, at any time the system being modeled is in some state. There are a finite number of states, however large, that can be listed. Each state is represented as a node. Multiple sequences of state (paths) can lead to a given state, but one is the most likely path to that state called the “survivor path.” This is a fundamental assumption of the algorithm because the algorithm will examine all possible paths leading to a state and only keep the one most likely. This way the algorithm does not have to keep track of all possible paths, only one per state. A second key assumption is that a transition from a previous state to a new state is marked by an incremental metric, usually a number. This transition is computed from the event. The third key assumption is that the events are cumulative over a path in some sense, usually additive. So the crux of the algorithm is to keep a number for each state. When an event occurs, the algorithm examines moving forward to a new set of states by combining the metric of a possible previous state with the incremental metric of the transition due to the event and chooses the best. The incremental metric associated with an event depends on the transition possibility from the old state to the new state.
The Viterbi algorithm is stated as follows in four major steps:
1) Initialization:
(9)
(10)
2) Recursion:
(11)
(12)
![]()
3) Termination:
(13)
(14)
4) Path (state sequence) backtracking
(15)
4.3.2. Reports (Extracted Tables)
The result of the best path given by the Viterbi’s algorithm is interpreted to its equivalent table in HTML code for proper evaluation.
4.3.3. Evaluation
The testing data set was evaluated to see how well the trained model performed the task of table extraction.
Evaluation was done measuring per token accuracy, precision, recall and f-measure for each extracted table in the tested documents. These evaluation measures are defined in Equations (16)-(21).
(16)
(17)
(18)
(19)
(20)
(21)
where A is number of correctly extracted cells (True Positives), B is number of cells existing but not extracted (False Negatives), C is number of cells extracted but associated with wrong labels (False Positives) and D is number of cells that did not exist and were not extracted (True Negatives).
4.3.4. Experimental Results and Discussion
Experiments were carried out using a self-generated data set. The training set was used to train the HMM and the testing set was used to evaluate the effect of extraction.
Table 2 shows the evaluation results of the hidden Markov model for automatic table extraction from heterogeneous documents from the dataset. Document 16 had the highest accuracy (93.0%), precision (98.2%), recall (94.3%) and f-measure (95.8%) values because it had the highest number of tables. Document 21 had higher values for accuracy (92.3%), precision (98.1%), recall (93.8%) and f-measure (95.6%) which had 21 tables than document 20 which had 23 tables with values for accuracy (85.0%), precision (94.5%), recall (88.2%) and f-measure (85.9%). The reason is because document 21though having 21 tables had some tables that are lengthy while document 20 does not have any lengthy table among the 23 tables it had. Documents 24 and 25 had the same number of tables and the least but document 25 had better values for accuracy (81.5%), precision (91.8%) and f-measure (87.4%) than document 24 while the two documents had the same recall value (85.2%). The table also showed that HMM is a fast learner. It had learned effectively for as low as 66 tables (with higher accuracy, precision, recall and f-measure than for one hundred and thirty-nine (139) tables and two hundred and five (205) tables). Increase in the number of tables seems not to be proportional to the effectiveness of the learning process of the model, as the overall and average accuracy, precision, recall and f-measure are higher for one hundred and thirty-nine (139) tables than for two hundred and five (205) tables.
Table 3 shows the comparison between Trigram HMM and Table Extraction from Heterogeneous Documents
![]()
Table 2. Accuracy, precision, recall and F-measure of HMM from self-generated data set from each document.
(Table Extractor) using HMM. It shows that the precision for Table Extractor HMM (the proposed system) is higher than that of Trigram HMM [7] with 3.3%. Recall and F-measure were better with Trigram HMM than that of Table Extractor HMM. The reason being that different experiments were performed by different authors with different datasets also, the number of data in the dataset used by each author differs.
Figure 2 shows that the proposed system has a good accuracy and recall, but better precision. Figure 3 shows that the proposed system has 93.5% average precision, 82.1% accuracy with a recall of 84.2%. Figure 4 shows comparison of Overall Precision, Accuracy, Recall and F-measure for different number of tables used for the experiment, that is, two hundred and five tables which is the total number of tables used for testing which is divided into two: sixty six and one hundred and thirty nine tables. Sixty six (66) tables have the highest accuracy, precision, recall and f-measure of 92.2%, 98%, 93.7% and 93.5% respectively.
Figure 5 shows comparison of Average Accuracy, Precision, Recall and F-measure for different number of tables used for the experiment, that is, two hundred and five tables which is the total number of tables used for testing which is divided into two: sixty-six and one hundred and thirty-nine tables. 66 tables have the highest accuracy, precision, recall and f-measure of 88.5%, 96.6%, 90.3% and 91.9% respectively.
![]()
Table 3. Comparison of precision, recall and f-measure of the trigram HMM and table extractor HMM.
![]()
Figure 2. Overall accuracy, precision, recall and f-measure in percentage.
![]()
Figure 3. Overall average for accuracy, precision, recall and f-measure in percentage.
![]()
Figure 4. Comparison of overall precision, accuracy, recall and F-measure for different number of tables.
![]()
Figure 5. Comparison of average precision, accuracy, recall and f-measure for different number of tables.
5. Conclusion and Recommendations
This research work presented how tables can be recognised and extracted automatically from heterogeneous documents using Hidden Markov Model (HMM). Smoothing was done for transition probability matrix and Viterbi’s algorithm was used to get the best path with the use of an algorithm which helped to display both the extracted table and its HTML equivalence; these were stored in a database and also displayed in web browsers. Automatic table recognition and extraction provide scalability and usability for digital libraries and their collections. Heterogeneous documents (except HTML documents) were initially pre-processed and converted to HTML codes after which an algorithm recognises the table. HMM was applied to extract the table portion from HTML code. The model was trained and tested with five hundred and twenty-six self-generated tables (three hundred and twenty-one (321) tables for training and two hundred and five (205) tables for testing). Viterbi algorithm was implemented for the testing. Evaluation was done to determine the accuracy, precision, recall and f-measure of the extraction.
In this research work, only Word, PDF and HTML documents were used; future work could accommodate other types of documents like Excel, PowerPoint and so on. Modification can also be done to this work in the nearest future to see that not only Google Chrome is able to do the extraction completely, but all internet browsers. A four-level cross validation is suggested for future work. Other means of validation apart from accuracy, precision, recall and f-measure can be used for evaluation.
NOTES
![]()
*Corresponding author.