Projection of Socio-Economic and Climate Scenarios’ Impacts on Food Security in West Africa: Case of Mali and Burkina Faso ()

1. Introduction
Climate change is nowadays one of the major prerogatives of the whole world. It is obvious to see that this is a threat for any continent, country and individual that are aware of it. West Africa is one of the most impacted regions by the effects of climate change. Indeed, it has been stated that the West African Sahel, between 10N and 20N, was experiencing a 25 years drought that began in the late 1960s and is still in progress [2] . This long period of drought could be ascribed to the El Nino Southern Oscillation (ENSO). Other studies had shown that the region has experienced one of the most striking shifts in climate known globally since instrumental records began to keep, from anomalously abundant rains in the 1950s and 1960s to progressively drier conditions in the 1970s and 1980s [3] . Moreover, the changes in the mean of climate over the years are affecting a lot of sectors. Such sectors could be forestry, the economy, health, and agriculture. It has been stated in the IPCC report that when rainfall regimes change, it is expected to drive changes in vegetation cover and composition, which may be a cause of land degradation [4] .
On one hand, the African continent’s economy is mostly based on primary sectors such as agriculture, fishery, animal breeding, etc. Moreover, the agricultural sector is playing an important role in the economy of West African countries. Thereby, the agriculture sector is the main provider of employment in this region. About 175 million people are working in the smallholder farms [5] . On the other hand, on average, this sector contributes about 15% of the GDP of the region [5] . Moreover, eradicating hunger and improving food security rely on agriculture.
Despite its importance in the regional economy, agriculture faces several huge constraints. However, rainfall high variability seems to be already settled as a future increased uncertainty [6] . This situation induces structural water stresses, production losses dues to shocking droughts at crucial growth periods and uneven spatial distribution of the productions and hampers expectations over agricultural activities from farmers and policymakers with thereby a lower investment in capital in these activities. Therefore, investigating how croplands are changing over the years under climate scenarios and how these changings are impacting crop production constitute a major stake in terms of development and food security over the region. The objectives of this study were:
● analyze the current trend of cultivated areas in Burkina Faso and Mali;
● determine the likely future changes in the cultivated areas in Burkina Faso and Mali under RCP4.5 climate scenarios;
● point out the effects of the likely changes in crop yield due to changes in cultivated areas on food security in Burkina Faso and Mali under climate and socio-economic scenarios;
● spatially represent the future cropland areas and daily intake per capita in both Burkina Faso and Mali.
2. Material and Methods
As any research project is conducted in a specific study area, targeted locations where to conduct this study were firstly identified. This includes two countries in West Africa, Mali and Burkina Faso. Once the study area was chosen, the needed data for this study were gathered. After collecting the data, defining the data tools, and data analysis process, was the next step in this research. Thus, the master plan is as follows in Figure 1.
2.1. Methods
2.1.1. Study Area
This study includes the spatial variability of climate change impacts in West Africa, especially in its two Sahelian countries: Burkina Faso and Mali as depicted in the figure below (Figure 2).
Burkina Faso and Mali are located between 12˚W, 4˚E, 9˚S and 30˚N. These two countries are part of the West African region where the rural population draws most of their resources from agricultural activities. Land use in West Africa has been experiencing changes. According to USGS, in Mauritania, Mali, Niger and the northern Sahel, the droughts of the 1970s and 1980s degraded or reduced some of the savannas and steppes and led to a 47% increase in sandy soils.
2.1.2. Data Collection
Diverse data sources were used in this study (Table 1). The socio-economic data were extracted from the Global Food Demand projections based on the IPCC Special Report on Emission Scenarios (SRES) storylines for the period 2005 until the year 2100 [7] . The dataset includes the national population and per capita food demand at the country level. The land use (cropland areas) data were extracted from Land-Use Harmonization (LUH) project database [8] . The LUH provides harmonized land use data for the years 1500 - 2100 at 0.5˚ × 0.5˚
![]()
Figure 1. Material and methods’ diagram.
![]()
Figure 2. Location map of Mali and Burkina Faso.
resolution under for different countries (Table 2). The four RCPs have been simulated using four Integrated Assessment Models (IAM) such as MESSAGE, IMAGE, MiniCam, and AIM. These RCPs’ scenarios have their corresponding socio-economic scenarios. The SRES and RCPs scenarios that are closed are RCP4.5 and SRES B1, RCP8.5 and SRES A2 [9] . Additional data have been extracted from the FAO database and from the literature review. These data concern the yields of the main cereal produced in Burkina Faso and Mali.
1) Crop items’ selection (FAOSTAT)
![]()
Table 2. correspondence table between RCPs and SRES.
As the objective of this study is to assess climate change impacts on food security, three main crops to be taken into account were identified: Maize, millet, and sorghum. These constitute the sources of nutrients in the diets of people in semi-arid regions of Africa. From the literature, it has been found in several studies that the three major crops grown in Mali and Burkina Faso are maize, millet and sorghum. According to Yarnell, maize constitutes the highest percentage of calorie intake in the national diet of 22 countries in the world among them 16 African countries [13] . In sub-Saharan Africa, maize accounts for almost half of the calories and protein consumed, and one-fifth of the calories and protein consumed in West Africa. [14] Santpoort stated that maize provides 19% of the average calorie intake per capita in southern and eastern Africa. Moreover, in 2015 FAOSTAT stated that Burkina Faso and Mali are among the top 20 countries that account for 96% of the total maize production in Sub-Saharan Africa [13] . In addition, sorghum and millet are also part of the most important consumed food in Africa. In a recent study, it has been stated that sorghum and millet constitute the main source of nutrition in the diets of people in the semi-arid regions of Africa [15] . According to Yarnell, Sorghum and millet are the most important cereals in West Africa after maize [13] . In addition, millet, sorghum, maize, and occasionally rice are the main crops in most of the provinces in Burkina Faso [16] . For the reasons mentioned above, maize, millet and sorghum were selected for this study.
Consequently, the research hypotheses that could assess climate change impacts on maize, millet and sorghum production were made to help have good perspectives of climate change impacts on food security in Burkina Faso and Mali.
2) Climate scenarios and socio-economic scenarios (RCPs and SRES)
It is important to assess some uncertainties while projecting climate change patterns. Such uncertainties might be how the climate might be sensitive to some increased concentration of Greenhouse gas in the atmosphere on one hand. On the over hand, the quantity of energy emission is involved to simulate different scenarios of the future emission. According to IPCC scientists, scenarios provide a framework by which the process of building experiments can be streamlined. Scientists then use the results of different scenarios to run complex models to project possible changes in the state of the climate in the future.
Numerous scenarios are nowadays available to simulate climate sensitivity and parameters. Indeed, the most used to drive climate model runs are among others:
• six IPCC 1992 (IS92) used in the IPCC second assessment report (SAR);
• six Special Report on Emission Scenarios (SRES) used in the IPCC third (TAR) and fourth (AR4) assessment report;
• four RCP scenarios used in the IPCC fifth assessment report (AR5);
• nine forcing scenarios developed for the sixth assessment report (AR6) based on the Shared Socioeconomic Pathways (SSPs).
a) Climate scenarios (RCPs)
The RCPs were developed after the publication of AR4 in 2007 to fit the needs of climate scientists in a relatively short time in which an update of the old SRES could not be ready for them to use and run simulations for the following report (AR5) supposed to come out by 2013. The following four pathways were developed: RCP2.6 which indicates 2.6 watts per meter squared increase relative to pre-industrial conditions; RCP4.5, RCP6.0, and RCP8.5. These are useful tools for modeling potential climate outcomes. Moreover, one could use SSPs rather than RCPs. But, because of the fact that there is some correspondences between RCPs and SSPs that explain their similarities coupled with the data availability, using the RCPs will be a better choice in this study. In addition, the RCP4.5 is known as a stabilization scenario of radiative forcing at 4.5 Watts × m−2 in the year 2100 including long-term global emission of Green House Gases (GHG), short-lived species and land-use-land-cover in a global economic framework [17] . This scenario is based on the minicam level 3 with incorporating updated land use modeling. It assumes that climate policies are invoked to achieve the global goal of limiting emissions, concentrations and radiative forcing. Moreover, it employed an updated historical data series, and a sophistical land-use-land-cover model.
b) Socioeconomic scenarios (SRES)
According to IPCC, the development of SRES took into account various possible future changes in the world’s development in the 21st century. This includes socioeconomic factors such as economic development and technological development, energy use and population growth. Four main storylines (A1, A2, B1, B2) describe the relationship between the forces driving GHG and aerosol emission and their global evolution. According to the IPCC scientist, B1 is a scenario with rapid changes in the economy, population with the reduction in resource-efficient technology.
c) Selection of climate and socio-economic scenarios
Our focus in terms of RCP is on RCP4.5. This is one of the most representative scenarios of the West African context. Its corresponding socio-economic data were used. It has been stated that, SRES and RCP scenarios that are closed are RCP4.5 and SRES B1 [9] . Population data from SRES B1 were used to estimate the impact of population growth on cultivated area per capita under RCP4.5. This correspondence has been established in the table of similarities between RCP and SRES Table 3.
![]()
Table 3. Similarities between RCPs and SRES.
2.1.3. Data Processing and Analysis
The analysis of data has been achieved in two steps:
1) Estimation of cropland evolution: the objective was to estimate cropland evolution from 2005 to 2100 under RCP4.5;
2) Assessment of climate change and population growth impacts on food security: the objective was to assess climate change and population growth impacts on cultivated land under a socio-economic and climate scenario.
Step 1: Cropland trends assessment
Firstly, cropland trends in different RCPs were assessed at two levels: grid and national levels. This assessment has been done in order to estimate the spatiotemporal impact of cropland trends from 2005 to 2100 in Burkina Faso and Mali.
As stated earlier, there is a relationship between population and cropland evolution. For that, the impacts of population growth on per capita cropland variability were estimated based on the national food demand in the selected RCP and SRES scenarios.
Based on this estimation, the per capita cropland cereal has been predicted from 2020 to 2100 in the selected RCP and SRES scenarios and taking into account the share of cereal in cultivated areas in both countries: Burkina Faso and Mali in the past 20 years.
Step 2: Assessment of population growth and climate change impacts on food security. For this assessment, the food demand per capita was projected for Burkina Faso and Mali under the SRES scenario. And the food production per capita was estimated after all. This assessment has been achieved using the projected cultivated areas.
1) Computation of the cultivated crops areas per capita
FAOSTAT provides a dataset about cultivated areas on almost all the crops in most of the countries. Projecting changes in cropland over the years to come, one needs to consider the changes in the past. Thereby, the dataset of the last 20 years about the cropland areas of all crops included was used to compute the percentage of cultivated areas of maize, millet, and sorghum. Accordingly, the mean values of these percentages were used to compute the areas attributed to respectively each crop type under the climatic scenarios considered in this study. Below is the formula used to compute the mean values of the percentage of cropland attributed to each selected crop item:
Equation (1): Crop item area under RCP. Source: author’s computation
(1)
where:
А is the area of the selected item;
δ is the projected cropland areas all crops included (global model output under RCP4.5);
n is the number of years;
κ is the area of the crop item;
γ is the year;
β is the total crop area all crops included.
2) Projection of crop area per capita
Defining the surface area exploited for an individual could be done once the total area and the number of populations are known. The details are obtained by dividing the former by the second. The formula below is an illustration of this statement.
Equation (2): Crop area per capita. Source: author’s computation
(2)
where:
a is the crop area per capita;
A is the total area of a crop;
ε is the population.
3) Yield Projection under climate scenarios
Yield projection in this study requires the changing rates that have been estimated in previous studies using simulation of crop yields under climate scenarios. These rates concern the values to which the crop yields may be changing in the future (e.g., 30 years to come). In our case we considered the projected changing rate estimated in 2019 by Freduah [12] . They estimated a decrease in yield of −0.13% and −0.13 respectively in Burkina Faso and Mali under RCP4.5. The formula below was used to compute the likely changes in crop yield.
Equation (3): Yield projection under RCPs. Source: author’s computation
(3)
where:
Y is the yield;
γ is the year;
r is the changing rate.
4) Projection of yield per capita
On one hand, assessing food security requires the quantitative values in terms of yield and population. Thereby, dividing the yield by the population will give insights on the quantity of grain per individual. The formula below was used for that.
Equation (4): projected yield per capita. Source: author’s computation
(4)
where:
σ is the production per capita;
Y is the Yield computed in the section above;
γ is the year;
and ε is the population.
5) Projection of daily intake under climate scenarios
Daily intake is defined as calorific energy that is taken by humans from their daily diet. It is expressed in kilo calories (kcal) in the international system. To define the daily intake, we need to convert the yield grain in calories, and divide the result by the number of days in the year (360, 265 days). The energy stored in, 100 g of maize, 100 g of millet, and 100 g of sorghum correspond respectively to 359 kcal, 341 kcal, and 339 kcal [18] . The formula below was used to compute it.
Equation (5): Daily intake per capita. Source: author’s computation
(5)
where:
λ is the quantity of energy (kcal) stored in 1 kg of the crop item;
σ is the yield per capita;
I is the iterator;
n is the number of items.
2.1.4. Spatial Visualization of the Results
1) Spatial database:
a) Database
A database is a collection of information that is organized in a such way that it can be easily accessed, managed and updated. Computer databases typically contain aggregation of data records or files, containing information about a company’s business such as billing and payment transaction with customers. Building a database either spatial or not, require data on one hand, and a DBMS on the other hand. Thereby the different results of our computation are used as input for our database. Of course, they are converted into SQL format to meet the DBMS requirements. In addition, a database is made up of tables which are organized into rows and columns. Moreover, a relational database that is targeted in this study is organized into tables and they are more often linked to each other. These links are called relations.
b) Database management system (DBMS)
A DBMS is a software package that is designed to define, access, retrieve and manage data in a database. It contains all the features such as tables and their relations. There are many kinds of DBMS, among others we have MySQL, PostgreSQL, MariaDB, etc. The used DBMS in this study is PostgreSQL. It is a DBMS with spatial functionalities and has a huge range of users.
c) Table:
In database management, tables refer to major entities or subjects of the system. A table is made of columns and rows. A column lists the different elements of an occurrence or a record and each row contains a record of the entity.
d) Relation:
Once the tables are ready, one can now think of how to link them in a meaningful way. Thereby, the established links refer to relations. One table can therefore be linked to one or more other tables.
Making data more attractive and decision driven is nowadays one of the main purposes of data science. One could see nice plots in a thesis report but, it could be more interesting to see in an interactive way the charts and graphs built from a dataset. Here is where the visualization tools come in.
2) Data visualization tools:
Data visualization is the graphical representation of information and data using charts, graphs, and maps. Thereby, data visualization tools make it easy to understand trends, outliers and patterns in the data. Like the databases, there are numerous data visualization tools such as tableau public, Power BI, visme, infogram, whatagraph, Sisense, DataBox, ChartBlocks etc… Unlike most of the rest, the first two tools (Tableau public and Power BI) are free and suitable for nice visualization. Thereby a description of each of them will make it easy to understand our choice.
a) Tableau public
It is a free platform where anyone can create charts, graphs, reports, dashboards, etc. Though they provide great possibilities with tableau public, some of the options cannot be freely accessed. In addition, tableau public can handle only excel sheets and text files.
b) Power BI
Like tableau public, Power BI is considered as the most preferred tool, it's also free to use and download. Among its peculiarities, it can use DBMS as a source of data. The data can be published in different views (mobile and desktop views). Thereby, this is our choice in terms of data visualization tools.
2.2. Materials (Tools)
A range of tools were used in this study to store, analyse, and visualize data. PostgreSQL was the database management system that much better with the context of our study for the data storage. Python is a great data analysis tool that was used to analyse our data and PowerBI was use to interactively visualize our data.
2.2.1. PostgreSQL/Postgis
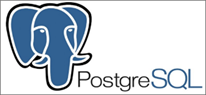
PostgreSQL often called just Postgres, is an object-oriented relational database management system with more flexibility that safely stores and scales the most complicated data workloads. It has a strong reputation for its best architecture in structuring data to ensure data integrity and reliability. It has more features with a wide community that consistently delivers performant and innovative solutions. In addition, one of its major flexibilities is its spatial features which allow users to build spatial databases dedicated to storing georeferenced data. That is what guided our choice on it.
2.2.2. Python
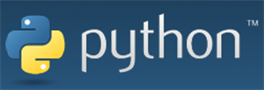
Python is an object-oriented, high-level, and interpreted programming language. Its dynamic typing and dynamic bidding combined with its high-level built-in data structures make it easy to manipulate datasets having numerous lines of information. In addition, its interpreter and extended standard library are freely available for all major platforms and can be freely distributed. Python provides increased productivity because of its fastness in debugging and its ability to save time by scaping the compilation step, therefore programmers love it. Moreover, it is easy to manipulate various types of data with Python by just importing the right library at the right place and at the right time. It has several text notebooks that give users an extremely flexible choice in what to use while writing their code. Jupiter-notebook is the one that is chosen in this study. In dealing with, CSV and excel files in this study, the corresponding libraries are always imported in the computation steps. The libraries to be mostly used are pandas and NumPy for data manipulation and matplotlib for the plotting stuff.
2.2.3. PowerBI
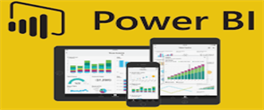
Power BI is a collection of software services, apps and connectors that work in synergy to turn unrelated sources of data into coherent, visually immersive and interactive insights. Considered the most preferred tool, Power BI is used for different purposes based on one’s needs. But mostly, it is used to make reports and dashboards. It also allows nontechnical business users with tools for their data analysis. Among its peculiarities, DBMS can be used as a source of data and the reports can be published in different views (mobile and desktop views). Thereby, this is our choice in terms of visualization tools. Though it has some great advantages, one should be linked to an organization in order to use it efficiently.
3. Results and Discussion
This study aims to assess the capacity of Burkina Faso and Mali to feed their own population in the future. It includes the historical, and the projection, of the cultivated areas, the projection of yield, and the projection of daily needed energy in calori, under the RCP4.5 scenario.
3.1. Historical Data of Cultivated Areas in Mali and Burkina Faso
The historical data in terms of areas dedicated to the different crops selected in this study are presented in the stacked histograms in Figure 3 below. It is important to note that each of the items in the histogram starts at the zero level on the x-axis. In addition, the data units are in millions of hectares.
Figure 3 shows an almost uniform decrease in cultivated land for different crops around 2000 in Mali. From 2001 until 2002, millet crops have seen a considerable increase while those of the other two crops have not experienced significant changes. From 2002 going towards 2004, a considerable decrease in cultivated areas of millet appeared. However, a small decrease was also noticed in the cultivated areas of maize and sorghum. Between the periods 2004 and 2010, the cultivated areas evolved almost uniformly and resumed their peaks around 2010 and 2011. Then they start to increase for a few years before showing a shallow change. In addition, the total change in cropland surface in Mali has been
(a)
(b)
Figure 3. Cultivated areas of maize, millet, and sorghum in Mali and Burkina Faso from 1999 to 2019.
gradually increased from 2000 to 2019 with continuous increases between the years 2000-2002, 2010-2011 and 2013-2017, and negative trends appeared between the years 2002-2003, 2011-2012. Almost constant evolutions appeared between 2005-2010 and 2012-2013.
Similarly, the historical cropland areas of the three main crop items in Burkina Faso have been analysed cf. Figure 3. The evolution of maize cultivated areas seems to not change as much as such between 2000 and 2007 while they are increased upwards for the two other crops between 2000 and 2003, then experienced decreases during the period between 2003 and 2006. From 2006 going towards 2013, the fluctuations are a little more significant in the cultivated areas of sorghum and millet. The cultivated surface areas of these crops then recorded more or less constant decreases until 2019. However, the corn has recorded an evolutionary growth over the years in terms of cultivated area. In general, the total surface area allocated to the three crops in Burkina Faso is evolving in an increasing way with some decreases observed in between the years 2000, 2004, 2006, 2007, 2009, 2011, 2014 and 2015. Table 4 summarizes the dataset used in both of the countries.
The following values were obtained: 11%, 30% and 20% respectively for maize, millet and sorghum as mean of dedicated crop area to these items in Mali.
Similarly, the changes were computed over Burkina Faso and occurred to be 10%, 22% and 28% respectively for maize, millet and sorghum.
This shows a global dedicated area of about 50% of the total cultivated area to these three staple crops from 1999 to 2019 in both of the two countries.
![]()
Table 4. Summary of the cultivated area of maize, millet, sorghum within the past 20 year in Mali and Burkina Faso.
3.2. Projection of Cultivated Areas
3.2.1. Projected Cultivated Areas of the Selected Crop Items under RCP4.5 Climate Scenario
Projecting changes over the years requires some statistics based on the past. Thus, the mean of changes in cultivated areas was firstly computed. This mean value was then used to compute the likely changes in the future considering that all the conditions (climatic and socioeconomic) remain the same. The projection is made using a multiplier based on the fact that a minimum threshold of variation of the cultivated areas in the future was considered. The figure below is an illustration of these changes.
Figure 4 shows that the cultivated areas are globally reducing in both Mali and Burkina Faso under the climate scenario RCP4.5. Though some increases could be noticed between 2040 and 2070, the actual state of cropland will not remain stable and will likely be decreasing from the current day up to 2100 by about 3%. In addition, the expected changes in cultivated land areas per capita were also computed under RCP4.5. The results are shown in the section below.
3.2.2. Projected Cultivated Areas per Capita of the Selected Crop Items under RCP4.5 Climate Scenario
After computing how the cropland areas could be evolving in a general way, it will be more interesting to see their evolution per capita. Thereby, the outputs of the projected cultivated areas are divided by the corresponding population estimated. The figures below illustrate the possible changes.
Figure 5 shows a global decrease of −3% in cultivated area per capita of millet and sorghum in Mali. Though the changes in harvested cropland areas seem to
(a)
(b)
Figure 4. Changes in cultivated areas under RCP4.5 in Mali and Burkina Faso.
(a)
(b)
Figure 5. Cultivated area per capita in Mali and Burkina Faso under RCP4.5.
be stable from 2045 all the way to 2100 in Mali and Burkina Faso. In addition, the cultivated areas per capita will likely be about 0.31 ha in Mali and could be about 0.46 ha in Burkina Faso. These rates in cultivated areas are in line with the FAO’s announcement that arable land per capita is decreasing. It fell from 0.38 ha in 1970 to 0.23 ha in 2000, with a projected decline to 0.15 ha per person by 2050 [19] . However, the difference between these figures and the results of our study can be explained by the different time horizons and the different scale.
3.3. Projection of Yield under Climate Scenarios
Cropland changes in its own will not be sufficient to narrow down its impacts on food security. Thereby, crop yield production is a great parameter which will reinforce and strengthen the results from cropland changes while stating their impacts on food security. Crop yield is the total quantity of grain that is harvested from a specific cultivated area (farm). It may be expressed in kg when the quantity is not so much significant, or in tones most of the time when the quantity is highly important. The FOASTAT database provides also some data about crop yield of the different crops that are grown in the two locations of our study area. We therefore computed the future possible changes in crop yield.
3.3.1. Projecting Yield under the RCP4.5 Scenarios
As stated in the methodology section, the projection requires some additional information that has been obtained from previous studies that used simulation of crop yields under climate scenarios (Figure 6). This information is values to which the crop yields may be changing in the future. Thereby, in 2019 Freduah [12] estimated a decrease in the yield of −0.13% as rate, under RCP4.5 in Mali and Burkina Faso.
The average projected changes in yield will likely be decreasing by 2% for each crop item between 2020 and 2100 in both Mali and Burkina Faso. This result aligns with some previous findings where Havlík and collaborators projected crop yield under RCP2.6 and RCP8.5 using global climate models’ outputs such as the HadGEM2-ES model and got some results that range between −10.2% and 10.2% [20] . In addition, Chisanga predicted some similar ranges in changes in crop yield rate. According to him the percentage change in grain yield would range from −3.81% to −8.88% under the RCP4.5 scenario [21] .
3.3.2. Crop Yield per Capita under the RCP4.5
The confidence of food security in a place depends sometimes on the number of tones that is produced to feed an inhabitant of this area. Thereby, getting insights of the harvested grain per capita will require both the information or data about crop production and the population data. The ratio of the formal by the second will give the quantity of grain produced for an inhabitant. In this section the population data were retrieved from the socioeconomic scenario (B1) which match with the RCP4.5 climate scenario cf. Table 3
1) Projected Population under RCP4.5
(a)
(b)
Figure 6. Yield projection in Mali and Burkina Faso under RCP4.5.
Among Global Models’ outputs, the socio-economic data such as population, and food demand were collected. In this section, the population data will be used to find out the specific size of cropland area that is been harvested to feed an inhabitant and the quantity of yield that is produced to meet his need in terms of grain. The colorific energy contained in the produced grain that could satisfy a person’s energy demand will be defined using the food demand dataset. The food demand dataset is compared with the projected calorific energy produced from the grain to point out the status of food security in Burkina Faso and Mali. Below is the plot:
In both Burkina Faso and Mali, the current population is above 20 million inhabitants. This is expected to grow till 2060 then it will reach its pick and remains constant till 2085. From this date, a decreasing slope is pointing out the possible reduction of the population under RCP4.5 scenarios (Figure 7).
2) Crop yield per capita under RCP4.5 scenario
As described up, the climate scenario RCP4.5 correspond to the socioeconomic scenario B1. Dividing the produced grain obtained under RCP4.5 by the population dataset will give the corresponding grain per capita. The following plot describes how an inhabitant is likely to be fed in the future.
In Figure 8, it is clear that the production per individual will gradually be experiencing some decreases between 2020 and 2050. These gradual decreases may continue until 2060, from which point stability is likely to be established until 2100.
![]()
Figure 7. Projected population in Mali and Burkina Faso under RCP4.5.
(a)
(b)
Figure 8. Production per capita in Mali and Burkina Faso under RCP4.5.
Considerable changes could be noticed in available yield per capita in the future. In Mali, the mean value of the changes is different from one crop to the other. Changes projected show a global decrease in yield produced per capita. Specifically, a decrease of 4% is expected in each crop item in both countries except sorghum which’s yield may be reduced by 5% in Mali under the RCP4.5 scenario. These changes could be explained by the fact that each crop area is expected to remain the same or to be decreasing and the fact that, sorghum and millet surface areas recorded a possible decrease of 3% in Mali.
Like in Mali, the production per capita in Burkina Faso also is likely to be reduced in crop yields between the years 2020 and 2050 from where production will be stabilized until the 2080s. An increase in yield per capita of some crop items can be expected from there until the 2100s under the RCP4.5 scenario.
3.4. Projection of Daily Intake in Terms of Calories under the RCP4.5 Climate Scenario
The human organism needs energy to be able to function well. This energy that allows him to breathe, to think, to move is in the calories. These calories are found in foods and drinks one consumes.
3.4.1. Food Demand
On one hand, knowing the possible food demand of a growing population such as the case of Burkina Faso or Mali, could help define the status of its hunger and thereby, have an overview of its food security status. Thereby, the socio-economic output gotten from global Models estimates possible food demand in both of the countries Burkina Faso and Mali. Below is the plot.
A growing population of a country will obviously lead to some increases in its food demand. Thereby, the food demand is just growing up from the current date till 2100 in Figure 9. On the other hand, getting insights into the calorific energy coming from crop yield production could highlight the level of satisfaction of the needs of a population, and could help a decision maker to take appropriate measures to meet these needs. Moreover, knowing the number of calories that seeds of a crop can contain could serve as an indicator of the satisfaction of the population’s needs for food.
3.4.2. Daily Intake Projection under RCP4.5
The likely daily intake estimation is projected by multiplying the yield per capita of each crop by the number of calories in this crop. The sum of calories of all three crops will then be divided by 360 and compared to the food demand in calories simulated by Bodirsky and collaborators [22] .
In the light of yield per individual under RCP4.5 in Mali, Figure 10 shows an overall decrease in the daily intake in kcal per individual in Mali under the effect of scenario RCP4.5. In addition, this decrease has been a savior from 2020 to 2050. From this point, though it’s shown the stability in the evolution of the daily intake, it could be an indicator of food insecurity since the daily intake is going
![]()
Figure 9. Projected food demand per capita in Burkina Faso and Mali under RCP4.5.
![]()
Figure 10. Projected daily intake per individual in Burkina Faso and Mali under RCP4.5.
to be under the normal. The WHO assumes that the daily dietary intake for an individual in sub-Saharan Africa is to be about 2540 kcal in 2030 which is greater than the projected.
Since the daily intake is a function of the produced yield per capita, it is then changed according to the yield production per capita under RCP4.5. The changes occur from 2020 to 2050 from which point it will get stability until 2080. In addition, some increase could occur from this date to the 2100s.
The daily intake per capita could undergo some changes in the years to come. The mean value of the changes is not for all the crops. Changes projected show a global decrease of 4% in daily intake per capita in both countries. Specifically, a decrease of 4% is expected in daily intake from each crop item in Mali, with some decreases of, 1% in daily intake from maize, and 2% from millet and sorghum in Burkina Faso over the years under the RCP4.5 scenario. Furthermore, the daily intake is likely to be about 1306.98 kcal, and 991.21 kcal respectively in Mali and Burkina Faso. But the highest daily intakes from this study are less than what was predicted by the WHO which is about 2540 kcal [23] . The differences in terms of daily intake may be explained by the fact that this study focused on three staple crops. The calories from other crops could encompass the gap in-between the WHO’s announcement and the results of this study.
3.5. Spatial Visualization of the Results
In most of the studies, the results are shown in figures and tables. An added value of this study was to make the results interactively accessible using smartphones and computers. Thus, a spatial database has been created was used as a source of data for the visualization tools. Concretely, building a spatial database implies defining steps like requirement statements, analysis and so on. But here we already have our input data for the database which are the outputs obtained
from the computation in the above sections. Thereby, these files were used to create the required tables in our database. Moreover, these files were in Comma Separated Value (CSV) format. They have been converted into SQL files to meet the requirements of the database management system (DBMS). The obtained SQL files were then executed on the database to get the corresponding tables. One more thing to be precised is that PostgreSQL has a plugin named PostGIS which is coupled with the spatial reference system to take care of the geometric aspects of the DBMS. The following steps are done after installing the PostgreSQL DBMS, the data visualization tool Power BI and the conversion of the CSV files into SQL files. The operating system was used in Windows.
Figure 11 is an overview of the web pages derived from the dashboards created with the different tables of our database. That could be accessed from anywhere by anyone it’s shared with.
4. Conclusions
This study focused on food security, considering socio-economic and climatic scenarios in two West African countries (Burkina Faso and Mali). It focused on the current situation of cultivated land and its evolution by 2100 under the RCP4.5 scenarios. An important aspect considered in this work was the availability of cultivated land to feed an individual in the future. It also considered the number of grains that could be produced in the cultivated area per individual and deducted the energy produced by this production per individual. This energy was then compared to what is the norm to verify the satisfaction of the calorific needs of an individual. Finally, a spatial representation of the results was made as an Added value to the work and which could be used as a tool to help decision-making.
The results show a current trend of increasing overall cultivated land area in both countries from 1999 to 2019. Beyond this increase, it turns out that with an increasing population and under the effect of climatic and socio-economic scenarios, the cultivated area per individual decreases over time.
In addition, the production of grains available to feed the growing population in each of the selected countries would be reduced as well. Consequently, the number of calories derived from these grains, which could be taken by an individual in either one or the other of the two countries, shows a general decrease. This energy is less than the quantity of energy that could satisfy an individual by 2100. Thereby food security is likely to be threatened in both Mali and Burkina Faso by the horizon of 2100.
Moreover, the combination of spatial database and data visualization tool enabled the spatial representation of the results of this study, which has been made as an added value to this work and can be used as a decision support tool.
Acknowledgements
My deepest gratefulness goes towards:
- WASCAL, the institution that funded my masters’ training;
- the staff of my training school Ecole Doctoral Informatique pour Changement Climatique (EDICC);
- Doctor Abdoul Aziz Belko, Doctor Kwame Oppong Hackman from WASCAL competence center
- my fellow students from bach1 and bach2;
- Mohamed Fomba, Issa Kassogue, Abdramane Doumbia, Haddy Jawla.
- Yaya Amadou Tessougue, Dr. Salif Bah, Yacouba Diallo