Overview of Cancer Management—The Role of Medical Imaging and Machine Learning Techniques in Early Detection of Cancer: Prospects, Challenges, and Future Directions ()

1. Introduction
Cancer is one of the leading causes of early mortality. According to the World Health Organization (WHO), cancer accounts for the highest worldwide burden based on disease-specific disability-adjusted life. WHO estimates that there are roughly 29.5 million new cases of cancer in low- and middle-income countries (LMIC) each year, making cancer mortality higher in these countries, even though high-income countries (HIC) have a higher overall cancer incidence [1] [2] [3] . Cancer morbidity and mortality are frequently linked to some factors like the stage of the disease’s manifestation, the tumour’s grade, and the accessibility of treatment options [4] . Several risk factors are associated with cancer, they can be classified into non-modifiable and modifiable risk factors. Age, gender, and inherited variables are among the non-modifiable factors [5] . Some tumours are linked to hereditarily mutated genes. For example, in breast cancer, breast cancer genes 1 and 2 (BRCA 1/2) are associated with a higher incidence of breast cancer in carriers of the mutated gene [6] . Another gene with a high risk of cancer is the adenomatous polyposis coli (APC) gene, it’s associated with colorectal cancer with a 100% chance of colorectal cancer in its carrier over its lifetime [7] . The modifiable causes of cancers include factors such as diet, obesity, sedentary lifestyle, alcohol consumption, and smoking. The population-attributable fraction of cancers associated with tobacco smoking is high in oral, and lung cancer [8] . In addition to these factors, there are several forms of cancer, including breast and prostate cancer.
Breast cancer is the commonest female malignancy, with increasing incidence worldwide. In Nigeria, the incidence rate is 54.3 per 100,000 [9] with about 80% of patients presenting with locally advanced breast cancer with a high rate of mortality compared to patients in HIC [10] . There are different types of breast cancer, ductal carcinoma in situ (DCIS) is a premalignant lesion; its cancer cells are confined to the breast ducts without invasion of the surrounding tissues. According to the grade of the lesion at the time of detection, there is a 14% - 53% chance that it will proceed to invasive breast cancer [11] [12] . Invasive Ductal Carcinoma is the most common type of breast cancer, it involves the breast ducts with the invasion of the surrounding tissues. It has the propensity to metastasize to various body parts. Invasive breast cancer can be classified into four stages, stage I and II are classified as early breast cancer while staging III (locally advanced) and IV (metastatic) [13] . General treatment for breast cancer involves breast-conserving surgeries, mastectomy, chemotherapy, hormonal therapy, and radiotherapy. Table 1 presents the different risk factors associated with breast cancer. Prostate Cancer is the second most common male malignancy after lung cancer worldwide. It has a higher incidence in African Americans, and it is associated with increasing age. However, its incidence in Africa and Asia is low. The mortality rate is associated with increasing age [3] [14] . The risk factors associated with prostate cancer include increasing age, genetic factors, ethnicity, family history, diet, and other factors [3] . Genetic mutations have also been associated with prostate cancer because the hereditary prostate cancer 1 (HPC 1) gene which encodes the ribonuclease enzyme is seen in patients with prostate cancer. The treatment options are based on the age of the patient, the physiological status of the patient, the hormonal sensitivity, the stage of the disease, and recurrence. As cancer incidence has increased over time, researchers have made strides in their comprehension of the molecular and cellular processes involved. A variety of imaging-based methods have been developed to track these processes in vivo, giving them the chance to better understand and treat cancer [15] .
Imaging in medicine is the technique of using technologies to acquire visual data about a specific patient. These technologies use algorithms or reconstruction techniques to produce images from different types of nonvisual data [16] . The amount of imaging data available for clinical decision-making has increased as a result of significant advancements in medical imaging (MI) and various methodologies including 3D ultrasound imaging, diffusion-weighted magnetic resonance imaging (MRI), and positron emission tomography (PET)/CT among others [17] . The rapid expansion of the potential use of artificial intelligence, including machine learning (ML), in several radiological imaging activities, such as risk assessment, disease detection, disease diagnosis, and prognosis, is another effect of this advancement in MI technology and the large amount of data produced [18] . ML techniques in MI analysis have increased tremendously due to their capacity to extract hierarchical features with strong representational capabilities, and this has led to several applications of ML in cancer prediction, and diagnosis [19] . Therefore, in this review, we discuss cancer management and imaging techniques used in cancer, we also examine the role of ML techniques in the early detection of cancer including the prospects, challenges, and future directions.
2. Imaging Techniques Used in Cancer Management
Medical imaging (MI) is a technique and process used to image the interior organs and tissues of the body for clinical examination, and medical intervention, and to portray the function of certain organs or tissues visually [20] . Clinical disease diagnosis, treatment evaluation, and the detection of abnormalities in several human organs, including the eye, the lungs, the brain, the breast, and the
![]()
Table 1. Risk Factors associated with Breast cancer.
stomach, depending heavily on medical imaging [21] . Various medical imaging techniques have been utilized in all phases of cancer management, and imaging modalities play a significant part in the management of cancer, including testing, diagnosis, treatment planning, and therapy monitoring [22] [23] . X-rays, computed tomography (CT), ultrasound, positron emission tomography (PET), magnetic resonance imaging (MRI), and mammography are a few of the well-known imaging modalities that are frequently utilized to observe anatomical, physiological, and molecular changes in malignant cells in both clinical and pre-clinical settings [15] - [28] . The general design of the MI system is shown in Figure 1. It is composed of a sensor that includes a source of energy that can penetrate the human body; as the energy passes through the body, it is absorbed or attenuated at varying levels depending on the density and the atomic number of the different tissues, resulting in the generation of signals. These signals are detected by the detectors that are compatible with the energy source and then mathematically manipulated to produce an image. The images acquired are through the energy from human tissue, resulting in a classification according to the energy that the body receives [29] .
2.1. X-Ray Radiographer, And Computerized X-Ray
X-ray is an imaging procedure that generates images of the internal structures of the human organ, especially the bones. X-ray radiography is a diagnostic method that uses ionizing radiation to see objects [29] . To create a profile for clinical imaging, x-rays move through the body and are absorbed or attenuated at varying rates, depending on the density and the atomic number of the various tissues. A detector registers these X-ray profile, and then produce an image of the internal part of the body [29] [30] .
Computed Tomography (CT) is a computerized X-ray imaging technique that consists of X-ray equipment, a monitor, and a cathode ray tube display to create images of the human body cross sections [29] [31] . Unlike the X-ray, a detector that records the X-ray profile has been installed in place of the radiographic film in the X-ray. Inside the CT scanner, there is a revolving frame with an X-ray source positioned on one side and a detector on the other. An image or slice is recorded each time the X-ray tube and detector complete one full rotation. As these X-ray tubes and detector rotate, the detector captures many profiles of the attenuated X-ray beam [29] . Every profile is converted into a 2D representation of the scanned slice by the computer. In addition, spiral CT can be used to
![]()
Figure 1. Schematic illustration of medical imaging systems.
obtain 3D CT images since it collects a large amount of data while keeping the patient’s anatomy in one place. To produce three-dimensional (3D) photographs of intricate structures, this amount of data set can then be reconstructed in the computer. The generated 3D CT scans aid in the three-dimensional imaging of the tumour masses [29] . Examples of X-ray and CT images are shown in Figure 2.
2.2. Ultrasound
Ultrasound is a diagnostic technique that creates medical images by using high-frequency, wideband sound waves in the megahertz range that reflect differently from tissue [29] [32] [33] . To achieve this, the patient’s skin is rubbed with an ultrasonic transducer close to the area of interest. High-frequency sound waves are emitted by the transducer, entering the human body and bouncing off the inside organs. The transducer picks up sound waves as they return from the internal structures. These sound waves are reflected differently by various tissues, creating a signature that can be quantified and converted into an image. The ultrasound device picks up these waves and converts them into real-time images [29] . In the diagnosis of cancer, endoscopic ultrasonography can detect lesions in the mediastinum, which can then be used to direct a fine-needle aspiration biopsy to find original tumours as well as spread from lung cancer that had previously been seen on CT [22] . This has demonstrated a significant advantage in preventing pointless thoracotomies. The diagnosis of gastrointestinal tumours such as oesophageal, and pancreatic cancer is also done with endoscopic ultrasound [20] .
2.3. Single Photon Emission Computed Tomography (SPECT) and Positron Emission Tomography (PET)
SPECT is an imaging method that uses drugs that have been marked with atoms that, when they decay, release at least one gamma ray. Since these gamma rays are often equally distributed throughout the electromagnetic spectrum, it is required to place a collimator in front of the detector so that only gamma photons that are directed at the detector can be detected. The collimator defines the radiation’s direction if it is detected in this manner. If the detector is rotated around the patient, a 360˚ image is obtained [29] . An example of SPECT brain image is
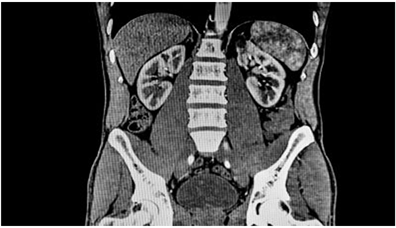
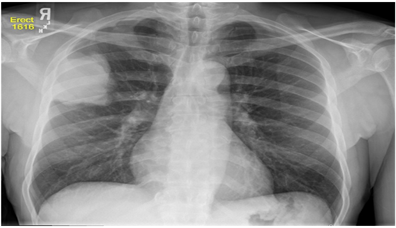
(a) Chest X-ray (b) Abdomen CT
Figure 2. Typical illustrations of X-ray and CT images (image courtesy of Duke University Medical School and National Institute of biomedical imaging and bioengineering (NIBIB) respectively).
seen in Figure 5. PET is becoming a significant imaging tool where images can be generated in 3D, and the intensity of the signal is proportional to the amount of tracer used. This suggests that the method is potentially quantitative [34] . PET and SPECT have similar techniques in that they both reveal details about a disease’s metabolism. Other imaging methods are unable to image certain physiological processes with the same specificity as PET, such as the rate of glucose or fatty acid metabolism. For cancer diagnosis, patients with androgen-independent tumours benefit greatly from the use of 18F-fluoro-2-deoxy-2-d-glucose (18F-FDG), which is the most used tracer for PET imaging [34] . According to recent studies, image fusion has been utilized to create special perspectives for gathering the data from two distinct exams to be correlated and evaluated on one image. This involves integrating PET images with CT images or with MRI images. This provides more reliable information and diagnoses. There are three combinations used in medicine: SPECT/CT, PET/CT, and PET/MRI [35] [36] [37] [38] [39] . Figure 3 shows an example of ultrasound, SPECT, and PET/CT images. Additionally, Table 2 outlines the primary distinctions between SPECT and PET.
2.4. Magnetic Resonance Imaging (MRI)
MRI is an imaging tool that records body chemistry and images body tissues using magnetic and radio frequency fields [29] [40] . The MRI machine has a scanner made up of three main components: the main magnet, a magnetic field gradient system, and a Radio Frequency (RF) system [29] . A permanent magnet serves as the primary magnet and produces the magnetic field. Three orthogonal gradient coils make up the typical magnetic field gradient system, which is crucial for signal localization. The RF system is consisting of a receiver coil that turns processing magnetization into electrical signals and a transmitter coil that can create a rotating magnetic field to excite a spin system. For cancer detection and therapy response monitoring, magnetic resonance is commonly employed. One of the first types of cancer to be evaluated by MRI is breast cancer, which is
(a) Ultrasound of gallstones
(b) SPECT brain image
(c) PET/CT images
Figure 3. Examples of ultrasound images, SPECT images, and PET/CT images (image courtesy of Mayo Clinic, London Health Sciences Centre, UK, and Mayo Clinic PET respectively).
![]()
Table 2. Key differences between SPECT and PET.
now beginning to gain acceptance as a supplementary tool comparable to mammography and ultrasound after years of clinical use [20] . Because breast MRI has a better sensitivity than mammography or ultrasound for the detection of breast cancer, it has proven to be helpful. Breast MRI has also been advised for recurrent examination of high-risk patients with a higher chance of radiation-induced DNA alterations because it does not use ionizing radiation. Additionally, it is frequently utilized to examine women who have a history of breast cancer in their families, has particularly dense breast tissue, or has silicone implants, which could mask disease on a mammogram [20] .
2.5. Mammography
Mammography is a type of imaging that assists in the early detection and diagnosis of breast tumours in females [23] . It can also be referred to as a human breast X-ray that creates a breast image using low dosage X-ray [23] [41] . A mammogram image consists of a colour bar made up of a range of colours that correspond to the image’s brightness or grey scale values. This serves as a reference for the radiologist interpreting the mammogram to accurately assess the density of the breast tissue. Also, diagnostic mammograms are utilized for patients with erratic symptoms, while screening mammograms are effective in determining the cancer risk in women without obvious symptoms. Furthermore, mammography may show breast alterations up to a year or two before the patient or clinician notices any symptoms [23] . Mammography has long been the mainstay of population breast cancer screening because it often identifies more in situ lesions and smaller invasive cancers than other screening methods like MRI and Ultrasound and is useful in discriminating between breast cancers [42] . Figure 4 shows some examples of images from MRI, and mammogram scans while Table 3 highlights the benefits, ionizing radiation effect, and applications of different imaging techniques used in the identification and staging of cancer.
2.6. Recent Studies on Machine Learning Techniques in Medical Imaging
Machine learning (ML) is a subfield of artificial intelligence that focuses on the development of algorithms and statistical models that allow computer systems to automatically learn patterns and relationships in data, without being explicitly programmed and this, can be applied to medical imaging data. This is possible because machine learning enables the extraction of meaningful patterns from images, which is a component of human intelligence [45] . There are several types of ML algorithms, including supervised learning, unsupervised learning, and reinforcement learning. In supervised learning, the algorithm is trained on a labelled dataset and can be used to predict labels for new or unseen data [46] . In unsupervised learning, the algorithm is trained on an unlabeled dataset and must learn to identify patterns and relationships in the data without any external guidance. This type of learning can be used for tasks such as clustering, dimensionality reduction, and anomaly detection [46] . Reinforcement learning is a type of machine learning where an agent learns to interact with an environment by taking actions and receiving feedback in the form of rewards or punishments. This type of learning has shown promise in applications such as robotics and game-playing. In the context of medical imaging, machine learning has been used for tasks such as segmentation, classification, and detection of tumours [46] .
Machine and deep learning algorithms hold a vital role in training the MI systems as a specialist which can be used further for prognosis and decision-making. The first step in ML typically involves computing the image features that are significant in making the prediction or diagnosis of interest. Several
(a) MRI images
(b) Mammogram scan
Figure 4. Examples of images from MRI, and mammogram scan (image courtesy of Massachusetts Institute of Technology (MIT), and University of Pittsburgh Medical Center respectively).
![]()
Table 3. Benefits, and medical applications of different imaging techniques used in the identification and staging of cancer.
machine-learning approaches have been adopted in medical imaging over the years. [47] surveyed image classification, pattern recognition, reasoning, and a few other concepts in MI. These concepts were adopted to increase accuracy in MI by identifying the important patterns for a specific application. There have been other studies on image segmentation such as the work of [48] , which introduced a new transductive method for 3-D prostate segmentation. The proposed method adopted doctors’ interactive information on labelling, to enhance accurate segmentation, mostly in a situation where there is an occurrence of large irregular prostate movement. To achieve this, the doctor manually assigns the labels for a few subsets of prostate and non-prostate voxels, specifically the regions of the start and last slice of the prostate. Thereafter, the transductive lasso was used to choose which features are discriminative slice-by-slice. This method was further used to assess prostate CT datasets and yielded promising results. The adoption of the transductive prostate segmentation method was informed by the transductive lasso for discriminative feature selection; also, the segmentation of transductive prostate for CT Image Guided Radiotherapy, as well as a weighted Laplacian, regularized least squares to predict a likely occurrence of the prostate. The use of computer-aided diagnosis (CADx) and computer-aided detection (CADe) has also been used in image analyses over the years. These techniques involve characterizing a region or tumour that was first identified by either a radiologist or a computer. This is followed by the characterization of suspicious areas or lesions well as the estimation of a probable disease by the computer, which leaves the management of a patient to a physician [8] [49] .
3. Advances in Cancer Diagnosis and Prognosis Using Machine Learning Techniques
Machine learning (ML) has gained popularity due to its cheaper, and fast computing power and memory for storing, processing, and in the analysis of massive amounts of data than the conventional methods of data analysis. This is especially significant to healthcare since the introduction of advanced technology in the medical field has led to the accumulation of a large volume of data, which has presented a new problem for medical practitioners and researchers in the realm of biosciences [50] . This accumulation of data comes from patients and advanced technology and utilization of ML technologies have proven to be effective as mixed data, like clinical, and genetic data can be integrated using machine learning approaches [51] . Over the years, advanced algorithms have also been deployed on huge datasets to uncover human-specific understanding and identify disease correlations [52] . A typical example is the use of Bayesian Inference for diagnosing Alzheimer’s which is dependent upon results of memory function and demographic information [53] . Nevertheless, in cancer, artificial neural networks (ANN) have been used to classify images of cancerous cells to determine their progression and severity. Other ML techniques for cancer diagnosis and prediction include Support Vector Machines (SVM) and Decision Trees (DT) [54] . These techniques can detect patterns and relationships within complex datasets while giving a prognosis of a cancer type. This shows the importance of machine learning in early cancer diagnosis and disease modelling, which have equally aided clinicians and patients in making informed treatment decisions and improving their quality of life [51] . Several studies like [55] - [57] have discussed the importance of ANN and the work of [58] , and [59] have shown several ways in which ANN has been applied in the prediction of cancer as highlighted in Table 4. The work of [60] which shows the application of DT in the diagnosis and prognosis of cancer is also highlighted in Table 5, and Figure 5 shows how DT can be used as a professional assessment in detecting and treating breast cancer. SVMs are one of the most recently used ML techniques in tumour prediction and prognosis [61] [62] . Table 6 also shows some research relevant to the use of SVMs in cancer diagnosis and prognosis [63] - [70] .
Some Advances in Predicting Cancer Susceptibility, Survival, and Recurrence
According to recent research, when compared to traditional approaches, ML improves cancer prediction accuracy by 15 - 25 percent on average [71] . In cancer susceptibility prediction, genetic algorithms are rarely used, and health professionals are unfamiliar with SVMs, DTs, and naive Bayes (NBs) [72] . For example, in 2004, a strategy for retrospective prediction of breast cancer occurrences using single nucleotide polymorphism (SNP) profiles of steroid metabolizing enzymes was developed [73] . For these techniques, the SVM classifier is found to be 69 percent accurate, whereas the Bayesian and DT classifications are 67 percent and 68 percent accurate respectively, with a 23 - 25 percent edge to chance [59] . Also, for cancer survivability, approximately half of the ML cancer prediction research focused on survivorship apart from life expectancy, progression, and treatment sensitivity [59] . Study [73] shows the combination of clinical and genetic data used to predict Diffuse Large B-Cell Lymphoma (DLBCL) patient outcomes using hybrid ML. Information from about 56 patients was mined from the International Prediction Index (IPI) to create a Bayesian classifier. The DLBCL classifier was 73.2% accurate. There is also the designing of an Evolving Fuzzy Neural Network (EFuNN) in the work carried out by [59] to harmonize genomic data utilizing 17 genes, providing 78.5% accuracy. The blend of EFuNN and Bayesian classifiers achieved 87.5% accuracy, 10% better than the top ML
![]()
Table 4. Summary of some relevant research adopting ANN in cancer diagnosis and prognosis.
![]()
Table 5. Summary of some relevant research adopting DT techniques in cancer diagnosis and prognosis.
![]()
Table 6. Summary of some relevant research adopting SVM in cancer diagnosis and prognosis.
classifier at the time [59] . The EFuNN classifier was verified using a leave-one-out cross-validation technique, probably because of the limited sample volume of 56 patients classified by 17 gene characteristics, producing a sample per feature ratio (SFR) of slightly above 3, this study is a huge process in cancer
![]()
Figure 5. An illustration of a simple DT as applied in detecting and treating breast cancer.
survivability [59] [71] [73] . Furthermore, some studies are aimed at predicting cancer’s recurrence, for example, studies [74] and [75] , combined some prognostic variations in forecasting the likelihood of recurrence among cancer patients for 5 years, including patient age, tumor size, estrogen, and progesterone levels, and the number of axillary metastases, among others. The study [76] used an ANN model to examine the data of 4926 breast cancer patients while the study [77] used an ANN model to examine the data of 2441 breast cancer patients with an SFR of more than 5. This data was externally validated using samples from over 300 breast cancer patients in another facility. ANN was found to be better than the standard tumor-node-metastasis (TNM) model, which had an area beneath the receiver operator characteristic (ROC) gradient of 0.677, whereas ANN with an area beneath the ROC gradient of 0.726.
4. Prospects, Challenges, and Recommendations in Using Machine Learning in Cancer Management
Machine learning has been used increasingly in recent years to extract gene expression data from microarray studies involving cancer [76] . Additionally, microarray data is used in computational biology to investigate various ML tasks where supervised learning is used to train the classifiers. Also, clustering algorithms have the potential to assist in the provision of more detailed and extensive knowledge necessary for biological inference about the fold of genes or samples [77] . However, there are also several challenges to be overcome in the use of ML for cancer diagnosis.
4.1. Challenges, and Recommendations of Machine Learning in Cancer
Despite the continued use of ML for cancer, it is crucial to note that there are still certain challenges, such as the need for appropriate benchmarks of microarray data sets relevant to new cancer databases, which has been complicated by an ineffective comparison or testing of ML algorithms [78] . The current barrier is that the data sets required to benchmark ML studies targeted at cancer research are scattered across personal, public, and academic repositories rather than being fixed in one location [79] . A carefully planned machine learning experiment is also essential, however not all experiments may be carried out with the same level of care as those indicated above. Both users and implementers of the machine learning model need to be able to detect any challenges within the system [63] . However, deficiency in the eagerness to improve data and trainee volumes proved a huge challenge, and this called for one to validate an assessment by use of an information source from outside [64] .
Another challenge includes the quality of the dataset, carefully selecting the feature as well as the quality of the characteristics selected to train the model. It is encouraged for a classifier to be compatible with various datasets in the long run. In addition, the use of many prediction models that are anchored on diverse ML techniques is valuable. The process of ML is hypothetical and should be based on computational processes. For this reason, it is important to have good documentation [59] . Knowledge of how to train as well as test the datasets needs to be well explained and made available to the public. Specifics about algorithms along with their codebases must be transcribed to allow for reproducibility as with any other standard lab protocol.
4.2. Prospects and Future Direction of Machine Learning in Cancer
Disease prediction by machine learning is strongly believed to have a strong future in the medical community [80] [81] . The development of the adjutorium, an online prediction tool based on machine learning that clinicians use to predict survival outcomes and treatment benefits for patients diagnosed with early breast after breast-conserving surgery, is an example of the explicit use of ML for disease prediction [82] . This model is a state-of-the-art automated ML, one that was trained using historical patient data. Adjutorium has the potential to improve accuracy and precision for every person by forecasting the patient’s most likely outcomes considering the most recent study and data. The model is constructed to give requests for use of a machine learning algorithm to forecast the patient’s survival profile under various treatment roles using data for comparable women diagnosed with breast cancer in the past. Another potential field is the crucial function of ML in the treatment of cervical cancer through cervical images, as early diagnosis of cervical cancer would lead to reduced costs in early screening processes [81] . This implies that high efficiency and flexibility in the treatment of cervical cancer teeming from optimal predictive risk factors can be addressed in genomic sequencing data which is the ML strategy in predicting with high accuracy. It’s also important to note that, in the past, high-risk patient tissue biopsies were only diagnosed as benign after surgery. However, it has been shown that AI assistance, which is a subset of machine learning, significantly contributes to a 30.6% decrease in the incidence of breast-conserving surgery (mastectomy), which is expected to rise significantly in the nearest future.
5. Conclusion
The World Health Organization cites cancer as one of the deadliest diseases in the modern era. A patient’s survival depends on the timing and accuracy of the diagnosis. Therefore, early diagnosis is essential, and deploying intelligent equipment to assist in this process is critical for treating patients. Hence, MI devices are excellent diagnostic and staging tools for cancer. Furthermore, in the current medical system, imaging is the primary method for cancer diagnosis and staging. This is accomplished using X-rays, computed tomography, ultrasound, positron emission tomography, magnetic resonance imaging, mammography, and other imaging devices. Some of these methods employ the use of magnetic fields to produce images of internal body organs, while others employ the service of sound waves or ionizing radiation. This review had shown that cancer can be diagnosed using well-established techniques in the medical imaging field. Also, ML techniques for MI analysis have increased dramatically in recent years due to their ability to extract hierarchical features with solid representational capabilities, and machine learning-based research on cancer molecular images is also expanding rapidly. In addition, most recent studies have focused on creating prediction models employing supervised machine-learning approaches and classification algorithms to predict accurate cancer outcomes. The paper also reviewed some studies that used machine learning concepts and their applications in cancer prognosis. In summary, it can be concluded that integrating multidimensional heterogeneous data and various strategies for feature selection and classification can give promising tools for inference in cancer detection and diagnosis.
Acknowledgements
The authors would like to thank the director and the entire executive team of Pan Africa Research Group (PARG) for their unique ideas in establishing a research platform where researchers from different backgrounds can collaborate to discuss revolutionary research.