Investigation of Combining Serum Tumor Biomarkers and Clinical Features for Elderly Lung Cancer Diagnosis and Classification ()
Lacking an effective and sensitive method to detect and screen the lung cancer in a large population is the main reason for the early finding of lung cancer. The treatment of lung cancer has already begun to be individualized in clinic currently. The individualized treatment depends on the lung tumor molecular characteristics, i.e., a selected subtype of lung cancers need to be treated in a specific way. In IPSS [4], the patients were treated with EGFR-TKI for the selected patients of EGFR mutations of lung cancer. Bang [5] used Crizotinib to treat the patients with EML4-ALK fusion the genotypes lung cancer. In JMBD [6] study, the Subgroup analysis suggested that non-squamous cell carcinoma non-small cell lung cancer (NSCLC) benefits more from pemetrexed therapy. Data from the NCI and SEER (Surveillance Epidemiology and End Results Date) [7] show that more than half of lung cancer patients are ≥65 years of age and most elderly lung cancer patients in the later stages. It is almost impossible to get the specimens for the molecular biological analysis. To make up the differences between the diagnosis and the treatment, there is an increasing attention on using the serum tumor markers (TM) to detect tumor for clinic diagnosis and classification [8]. A study published in 2008 used the six tumor markers, such as CA125, CA19-9 etc in a multiple detecting methods to diagnose ovarian cancer which could reach a high sensitivity and specificity of 97.5% and 99.7%, respectively [9]. These results have brought great interests in gynecological oncology community as a specific diagnosis of ovarian cancer. In 2011, Zhou’s [10] study found that plasma microRNA could be used as an effectively diagnostic marker for hepatitis B-related liver cancer and also it could be used as diagnostic model to distinguish the hepatic benign and malignant tumors.
Above findings of the lung cancer tumor markers provide some useful lessons: 1) multi-markers combination may replace a single one to give a better diagnostic value [11]; 2) It may be easier to get a breakthrough by focusing on a particular type of tumor than identification of the tumors from the normal or benign diseases [12]. Thus, this study selected the most commonly used clinical CEA, NSE, CA125, CA242, CA19-9, CA15-3 and SF lung cancer serum tumor markers for the objectives and the results were validated on the elderly lung cancer patients to check how the markers combination model assists the lung cancer diagnosis. For further application of this model in identifying the molecular typing of lung cancer patients, a non-invasive, simple and effective method for elderly patients with lung cancer diagnosis and classification needs to be conducted. This method will be used to distinguish among the major types of lung cancer such as adenocarcinoma, squamous cell carcinoma and small cell lung cancer and to guide the more efficacious clinical treatment.
2. Materials and Methods
2.1. Study Population
447 cases of elderly patients with lung disease detected by the tumor markers since July 2009 to October 2012 were selected, which included 246 cases of lung cancer and 201 cases of benign lung disease. All of these hospitalized patients have a complete clinical and pathological data such as detailed history, physical signs, chest and cranial CT, adrenal gland, hepatic-biliary and pancreatic spleen B ultrasound and radionuclide bone scan. There were 31 patients underwent the 18F-deoxyglucose-positron-emission-computed tomography (18F-FDG PET/CT) examination. Data for all of the patients’ age, gender, smoking status, general physical condition score (PS), the pathological type of lung cancer, diagnosis, clinical stage, concomitant diseases, fever and pleural effusions are shown in Table 1. The serum of lung cancer and other patients were collected but the patients who were carrying hepatitis B virus infection, rheumatoid and other autoimmune diseases were rejected. The lung cancer patients did not receive the radiotherapy and chemotherapy. The patient’s Performance Status was scored as 0 - 5 by Zubrod-ECOG-WHO (ZPS). The clinical TNM stages of Lung cancer patients were standardized by the American Joint Committee on Cancer staging of lung cancer, AJCC 7th Edition. The non-lung cancer patients were the benign lung disease that included chronic obstructive pulmonary disease, pulmonary fungal and bacterial pneumonia, interstitial lung disease and pulmonary heart disease.
2.2. Tumor Marker Testing Equipment and Methods
All the 5 ml blood specimens were taken in a fasting condition and put into a non-phylogenic, endotoxin-free test tube without anticoagulant and then centrifuged to collect the no-hemolytic. The tumor markers were tested by clinical laboratory in accordance with the instructions and operating processes of C-12 protein chip detection, the application software of the biological chip image analysis system (China, Zhejiang) and the HD-2001A biochip reader. Recommended tumor markers of diagnostic positive reference sector value are: CEA < 5 ng/mL, CA-199 < 35 U/mL, CA242 < 1.5 U/mL, CA15- 3 < 35 U/mL, CA-125 < 35 U/mL, NSE < 13 ng/mL, and SF < 219 ug/L.
3. Data Processing and Discriminant Analysis
SPSS16.0 statistical software was used for variable data statistics and analysis. All collected cases and controls would use the normality and homogeneity of variance test. Each variable rate between the two groups compared by t-test, P < 0.05 was considered statistically significantly different. Discriminate analysis process involves : opening the main discriminate analysis dialog window, specifying the tumor type for categorical variables and categorical variables, defining Range 0 - 3 and the re-
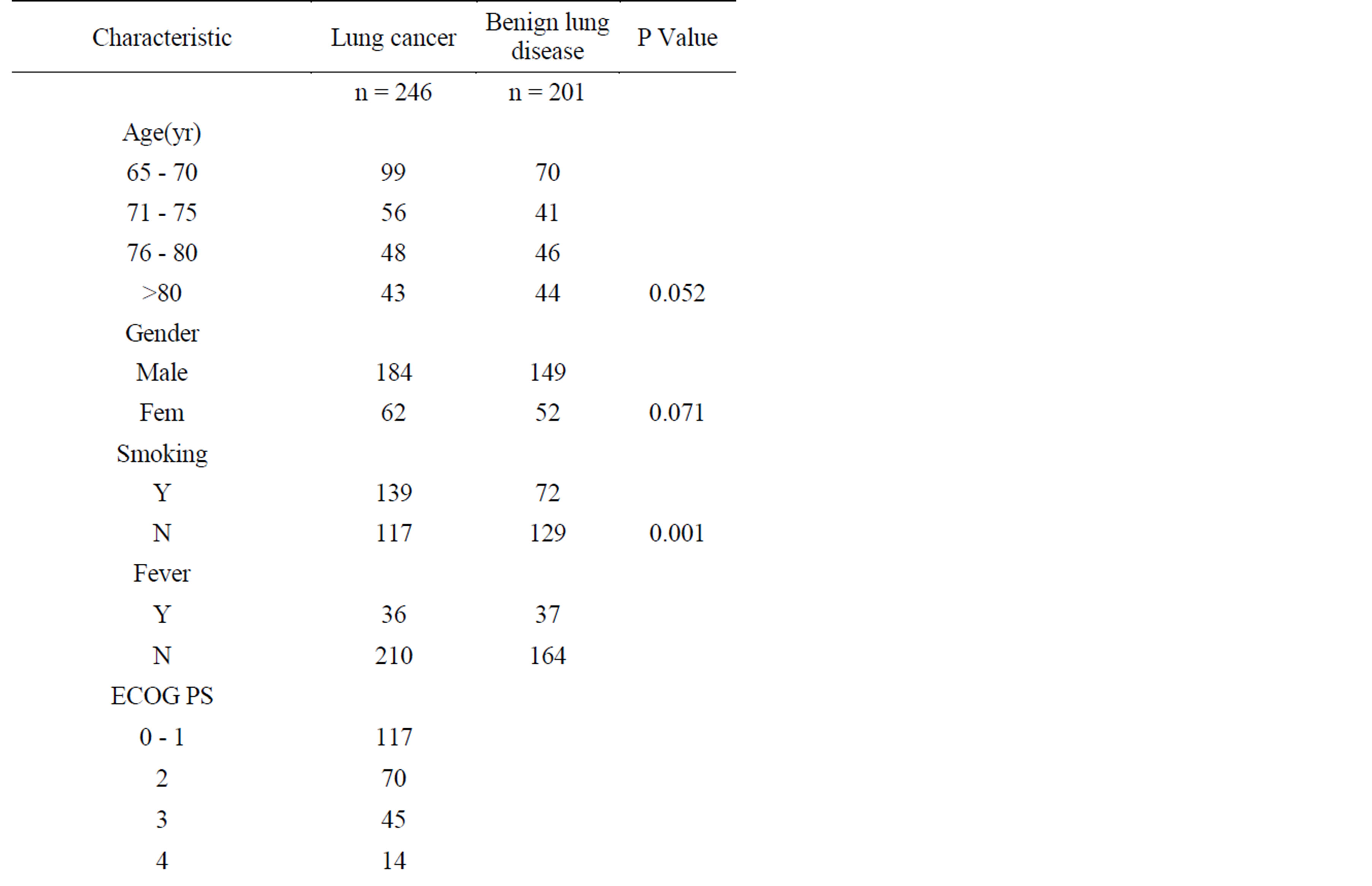
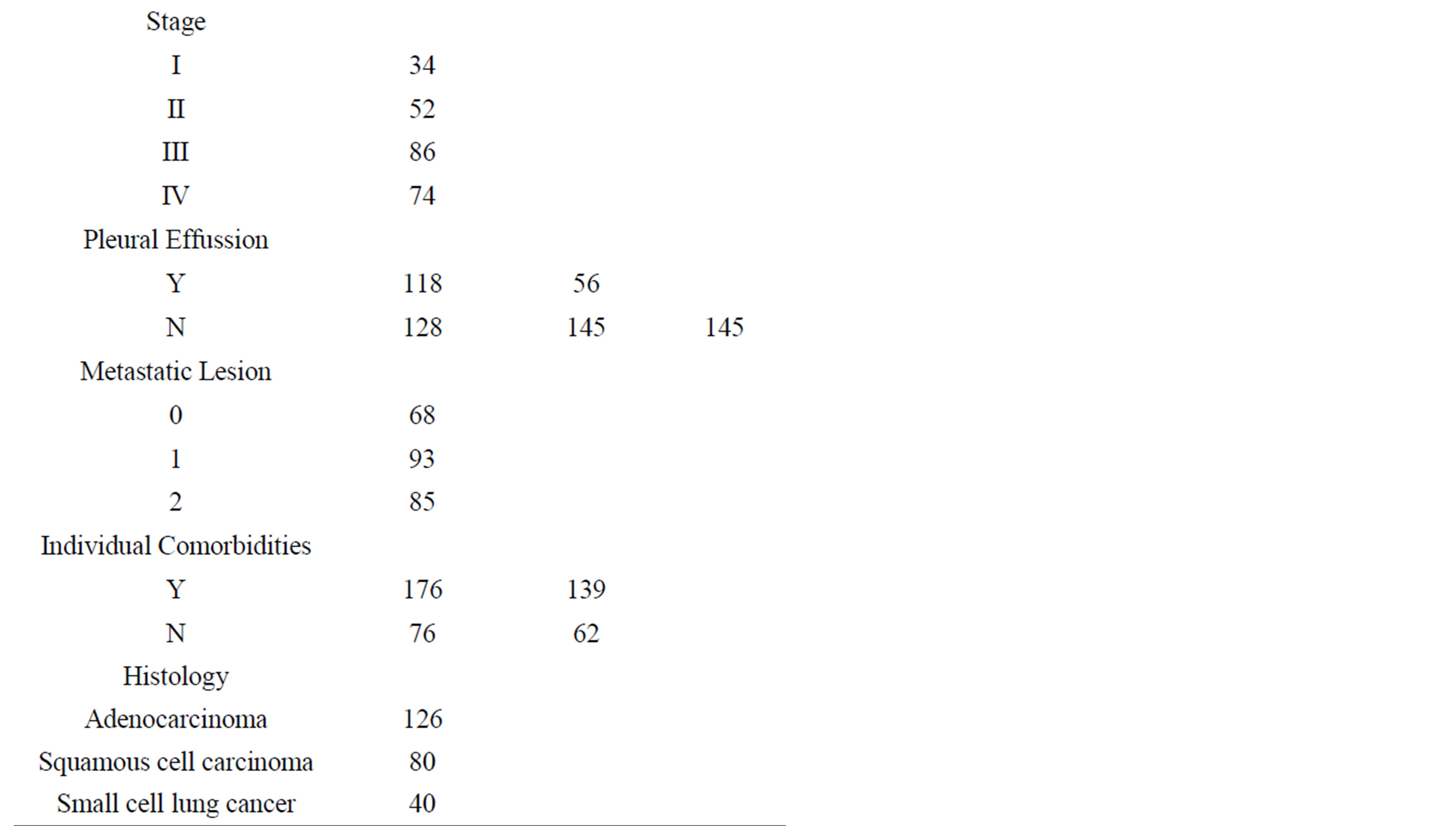
Table 1. The baseline characteristic of lung cancer patients and benign lung disease.
maining variables for the analysis of the independent variables, establishing the full model and using the Mahalanobis’ distance as a discriminant analysis method; defaulting entry value of 3.84 and the removal value of 2.71. The Select Display Statistics dialog box, including Means, Function Coefficients and the Fishern’s. The discriminate rules: calculate classification discriminate function which overall distance of the sample will be a discriminating point distance. It may be part of a class, define the overall category of the focus of the discriminate function. In this study the variable value is the tumor type, where 0 represents the non-cancer patients, value 1 represents lung adenocarcinoma, value 2 represents lung squamous cell carcinoma and value 3 represents small cell lung cancer. The remaining variables are the measured value of serum markers and also include patient’s age, gender, smoking status, a merger of the pleural effusion, fever and other laboratory and clinical indicators.
4. Results
1) The various tumor markers balancing test between the tumor group and the controls showed no statistically significant difference (P > 0.05) between the two groups in terms of age, fever, complications. There is a significant difference between the smoking and non-smoking patients (P < 0.01).The results are listed in Table 1.
2) Comparing the tumor marker expression in different groups, there is a statistically significant difference (P < 0.01) between tumor and control patients. The results suggest that a single tumor marker for lung cancer diagnosis and control patients has the clinical significance. The results are showed in Table 2.
3) The single and combined detection of tumor markers for lung cancer and controls groups with qualitative is still not satisfactory, just by calculating various indicators of sensitivity and specificity and Youden’s index between trail or controls groups the patients without lung cancers. The results are demonstrated in Table 3.
4) The discriminating analysis model is built by using Discriminate Analysis that conduct the Stepwise Statistics and full-access mode screened CEA, NSE, smoking and age, (the four variables of the discriminate model,) and Eigen values 0.162 to produce an equation into the analysis process. Wilks, s Lambda is 0.860. The Function at Group Centroids in the lung cancer group is 0.363 and −0.445 in control group. The Fisher’s linear discriminant equation for non-lung-cancer patients can be presented as:
(1)
and for lung cancer patients:
(2)
Classification of patients can be determined by calculating individual case using the above two equations. The variables were randomized to verify the discriminant function correctly which distinguished 67.6% of patients
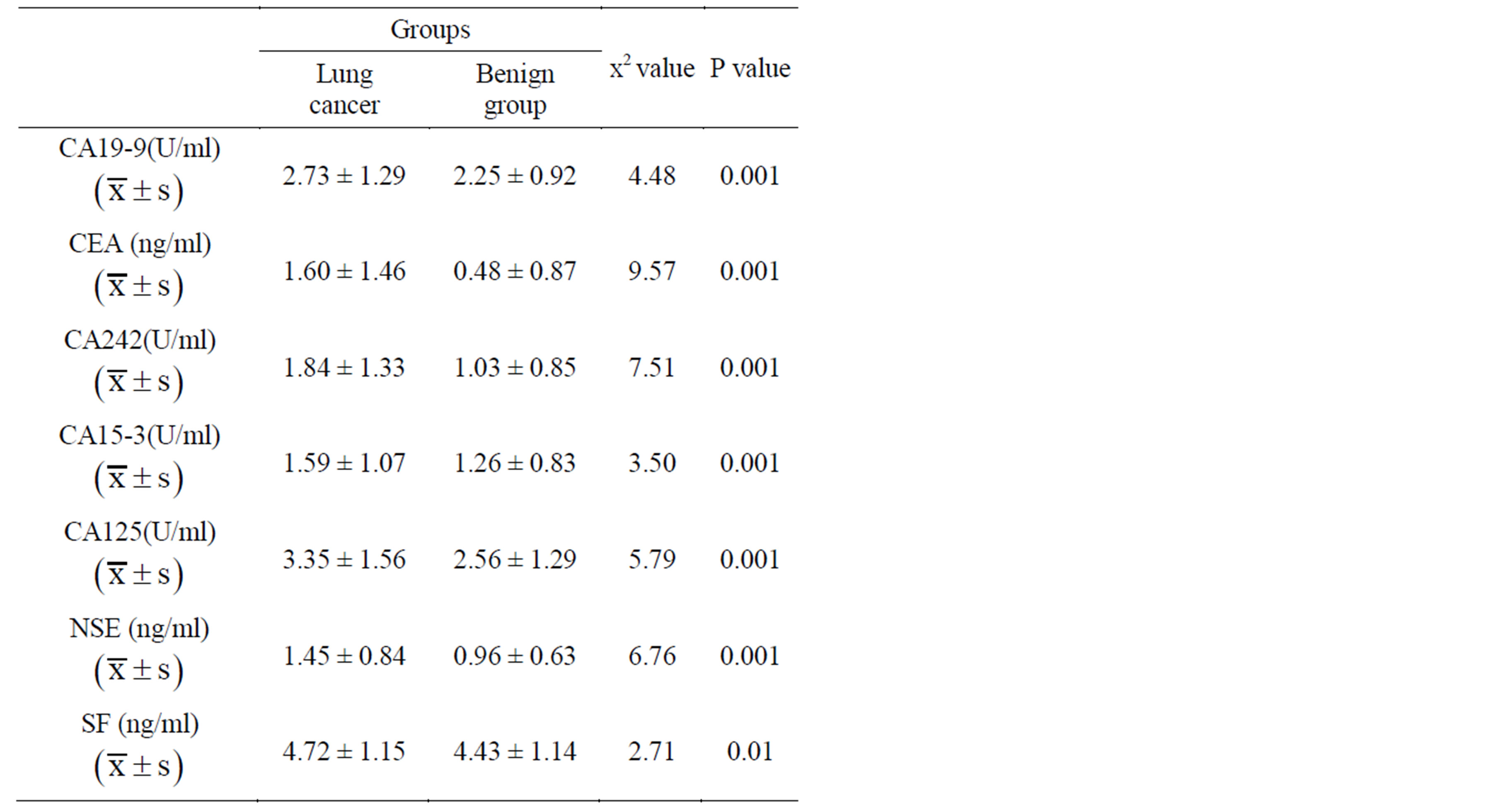
Table 2. Comparison of biomarkers between trail group and compared group.
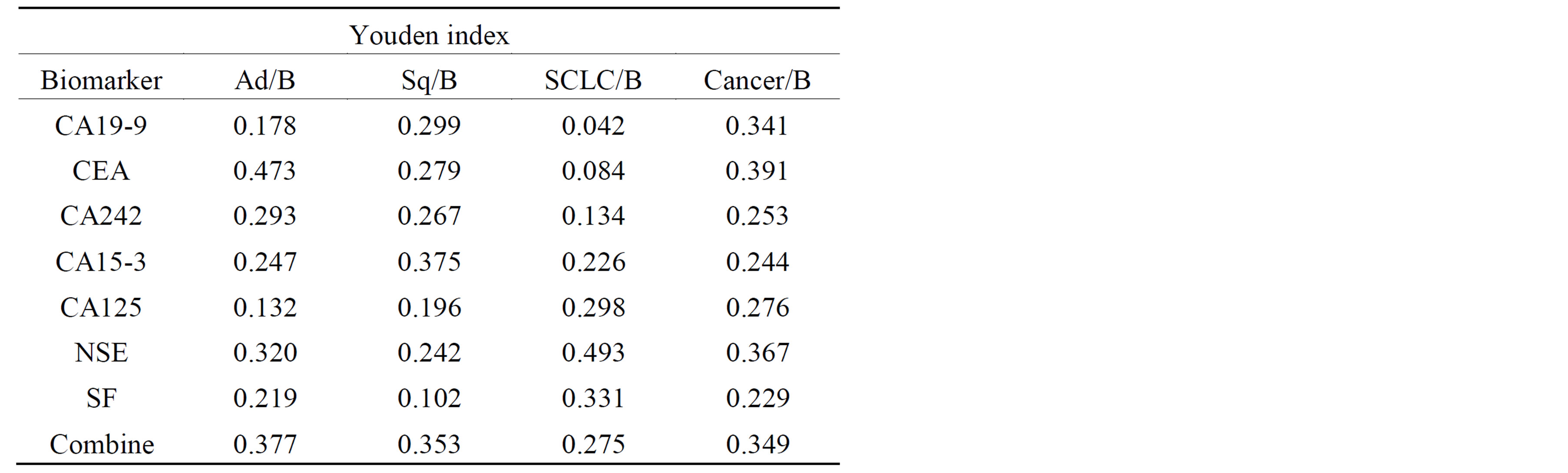
Table 3. TheYouden index of single biomarker or combine test for lung cancer over all and Ad, Sqq, SCLC.
with lung cancer and non-lung cancer patients. Crossvalidation of the equation can be obtained 66.9% correct discrimination. The results are shown in Table 4.
5) A stepwise discriminate analysis was used to get a correct classification from distinguishing the subtypes of lung cancer and the detection of tumor markers model for lung adenocarcinoma, squamous cell carcinoma, and SCLC major Lung cancer types. The lung adenocarcinoma, squamous cell carcinoma and SCLC were labeled as 1, 2 and 3. By applying Discriminate Analysis and Stepwise Statistics, fully entry mode still screened CEA, NSE, smoking and age as the four variables in discriminate model. The model generates two discriminate equations. In Equation (1), Eigen value is 0.064% of Variance 76.6 while in Equation 2 the percentage of Variance is 23.4. Thus, it has a closer relationship with Equation (1). The Wilks’s Lambda is 0.922 and Prior Probabilities for Groups include 0.512 adenocarcinoma, squamous cell carcinoma 0.325 and SCLC group 0.163, respectively. The established Fisher’s linear discriminant equations were derived separately, for lung adenocarcinoma patients
(3)
for lung squamous cell carcinoma patients
(4)
and for small cell lung cancer patients
(5)
The results were randomly selected to verify the model. The equation can correctly classify 54.9% of patients with lung cancer type and with cross-validation of the equation can obtain 52.4% correct discrimination. The results are shown in Table 5.
5. Discussion
The treatment of lung cancer has entered the new era of individualized therapy under the guidance of the molecular and gene targeted drug development. Successful applications for different physical conditions of the lung cancer patients have different treatment options and strategies. For example, in the NCCN (2012 edition) guideline even in clinical Stage Ⅳ, ZPS rated 4 classifications patients with advanced later stage can still be treated with Erlotinib [13]. Due to the lack of breakthrough in the diagnosis of lung cancer, the clinicians have to face more elderly, frail, or patients cannot obtain the histological and

Table 4. Classification results and cross-validated grouped cases correctly classified.

Table 5. The classification results and cross-validated grouped cases correctly classified for lung cancer type discriminated.
pathological examination. The date show majority of the lung cancer patients are elderly and about 60% of patients are older than 60 years, and also around 40% patients are even older than 70 years [14]. How to take a simple, minimally invasive or non-invasive diagnosis of high titer method does not only depend on the histopathological diagnosis of lung cancer in these patients so accurate typing, screening targeted therapy of patients become urgent clinical problems to solve. One of the strategies is the use of tumor molecular markers for lung cancer molecular diagnostics, molecular typing and molecular staging that could effectively combine the molecular targeted therapy with the complete chain of Molecular Biology of Cancer.
There are a lot of tumors markers having been used for the clinical diagnosis of lung cancer. CEA (carcinoembryonic antigen) is one of the commonly used serum tumor markers. CEA is correlated with the histological types, TNM stage of the lung cancers [15-17]. Some of the studies found that using gefitinib treatment may associate with the CEA level and the sensitivity of targeted therapy response rate in patients [18,19]. This result suggests that CEA may be a lung cancer tumor marker molecule. Other common tumor markers such as CA19-9, NSE, CA242 and CA15-3, CA125, SF are researched extensively, but there are large differences in the coverage of each, with reasons are that related with different specimen type, the patient clinical staging and testing methods [20]. These are particular important in measuring of the tumor markers. Different methods to detect specific tumor markers and to evaluate the positive boundary could also affect the diagnostic sensitivity. Tumor protein chip used in this study is a solid chip that a specific tumor marker antigen has been fixed on the chip surface through microarray technology. The tumor marker is detected by electro-chemiluminescence method, having a high chemical stability. The sensitivity and chromogenic substrate specificity of chips are as similar as the isotopic-assay and has more advantages than the more commonly used Enzyme linked immuno-sorbent assay (ELISA) method [21]. The chip technology has been approved certificated by the state health department and also has been used of stabilized in clinics for many years. Thus, the data from this study use above method to detect Lung cancer tumor markers in serum is reliable.
The clinical features of lung cancer patients are important in the diagnosis and analysis of data collected in this study. These features include age, gender, smoking status, PS score, pleural effusion in patients with lung cancer clinical data, to more objective and concise lung cancer description for clinical diagnostic reference. Simple clinical manifestations, diagnosis of lung cancer is feasible, but less accurate. Although Spitz [22] collected clinical data of patients with lung cancer by using a fairly complex mathematical statistical method and the classification tree discriminant analysis to establish lung cancer patients with the clinical diagnosis and risk prediction model, the results are not yet fully meet the needs of clinical diagnosis. This study provides an idea of advanced mathematical statistical methods and diagnostic model that is a good way to solve this complex clinical diagnostic problem. The result from the laboratory and clinical tries in this study by using the discriminant analysis creates a model for diagnostic. The data show the CEA expression levels in the serum of lung cancer and patients with benign lung disease with significant statistical difference (P < 0.001), but the low specificity and sensitivity in the differential diagnosis of type of lung cancer and benign diseases even with the highest Youden index 0.471 have only a limited value for clinical diagnosis. The other tumor markers also have such problems. In addition, the combined of various tumor markers diagnostic series test, Youden index hovering at between 0.25 - 0.377, the results are still not satisfactory. However, in cancer patients with clinical symptoms and laboratory test data for a comprehensive analysis of discriminate analysis method used to gradually enter screening, CEA, NSE, age and smoking status as diagnostic discriminant factors, in the differential diagnosis of lung cancer and non-lung cancer patients, the discriminant function correctly distinguish 67.6% of patients with lung cancer and non-lung cancer patients, cross-validation of the equation can be obtained 66.9% correct discrimination. Faced a clinically suspected cases, it does not depend on the pathological diagnosis, nor the use of two obvious clinical indicators and serological markers, the answer is lung problems are valuable only to a certain extent. Patients age defined in the obvious, smoking status can be easily obtained through interrogation serological CEA, NSE determination of minimally invasive and easy to carry out, the burden of the patient’s medical economics is limited, and these are used to the advantage of the diagnostic model. Further after carrying out the diagnosis of lung cancer, the discriminant model is composed of four indicators above. The various indicators linear discriminant coefficient is the difference in the effectiveness of identification of lung adenocarcinoma, squamous and SCLC the ability of the three main types of lung cancer, the discriminant function correctly points 54.9% of patients with lung cancer type and after a randomized cross-validation of the equation can also be obtained 52.4% correct discrimination. As clinicians, the physical presence of a patient is essential to confirm the diagnosis, the use of simple and effective way to get a satisfactory result for clinical expectations; the combination clinical features of the tumor serum markers discriminant equation can to some extent, be part of the answer to this problem. The past clinical empirical judgment by mathematical methods to quantify a rigorous scientific process of logical operations, thereby reduced the error of empirical judgment; and, after clinical validation of large sample, the diagnostic model can be used as auxiliary automatic diagnosis system for large-scale population screening has a significant advantage compared to the lower dose spiral CT, chest X-rays and other tests etc. It’s simple, economic, and fewer adverse reactions, easy to equip and scale for the acceptance of small medical institutions where other screening tests could not be substituted.
It can be found in the analysis of the Institute screening diagnostic model, diagnostic potency of this could be improved about 70%. One such method is the need for the introduction of new markers. Lately, some lung cancer serum tumor markers, such as Cyfra21-1 [23], ProGRP [24], CEA microRNA [25] have been used for diagnosis. The results of clinical studies suggest that they have higher diagnostic values and clinical points period, tumor histological type correlation. In addition, the application of proteomics new molecular biology techniques, such as the screening of new tumor markers; as well as using the support vector machine (SVM) technology and advanced statistical methods, such as the establishment of the new combination of molecular markers diagnostic model research direction [26,27] will improve the potency. However, these new markers of clinical potency, detection methods, clinical operability need to be stored in-depth and application of advanced mathematical statistical methods for model selection needs to be further explored for feasibility and the success of clinical applications. Currently there is no uniform development of a diagnostic kit of the new markers.
6. Summary
As an important aspect of this study, the use of serum tumor markers in patients with benign disease detection level as the control group, compared with the normal population has a differential diagnosis of expanding the sample size further to the normal population as the follow-up to this study. The test is worthy of further research, as more serum markers are added and application of new technologies for biomarker discovery screening joint intelligent advanced statistical analysis method may promote the improvement of the diagnostic value of serum tumor markers. However, based on the status quo, the simple, economical and easily accepted model tested in this study can effectively solve the diagnostic problems.
Acknowledgements
Dr. Li Sun, Dr. Yingxuan Tian and Dr. Min Yu have designed the experimental project and drafted this paper. Dr. Weifeng Liang, Dr. Xiaoping Ren, Yajun Ren and Hongxia Wen have taken part in the data collation and analysis to establish the diagnosis model. Dr. Zhuo Yang, Shenghong Wei, Wenli Shang, ShufenHuo and Cairong Wang participated in the experiment. We also appreciate that Dr. lingXia Zeng, a professor of the Department of Epidemiology and Statistics, Xi’an Jiao tong University, whose guidance and assistance us in the processes of the experimental data process and analysis.
Foundations and Supports
This study was supported by Program for Social Development and Technological Projects of the Science and Technology Department of Shaanxi Province (2011K13- 02-08), Research for Key Projects of the Shaanxi Provincial Health Department (No. 2012A2) and Research for Projects of the Shaanxi Provincial Health Department (No. 2012 D85), and the Nature Science Foundation Program of Shaanxi Province (2013JM4038).
NOTES
#These authors contributed equally.
†Corresponding author.