Detection and Diagnosis of Urban Rail Vehicle Auxiliary Inverter Using Wavelet Packet and RBF Neural Network ()
1. Introduction
Auxiliary inverters are one of the most important and common components in urban rail vehicle applications, and their failures can cause both personal damage and economic loss, if the fault cannot be detected and diagnosed well in advance. The quality and reliability of the vehicle auxiliary inverter directly affects the running safety and passenger’s comfort. Therefore, it is significant to be able to accurately and automatically detect and diagnose the existence and severity of the faults occurring in the auxiliary inverter.
With the development of failure diagnosis, there are many methods which are used to detection and diagnosis sites, for example, FFT, STFT, wavelet transform, neutral networks [1-4] and soon. Wavelet packet analysis has been proved as effective signal processing techniques for a variety of signal processing problems. It is a time-frequency analysis tool that allows an adaptive subband decomposition of the signal. It is possible to choose in a binary decomposition tree, the optimal subtree optimizing a given criterion [5]. Neural networks have become a popular tool in the fault diagnosis due to their fault tolerance and capacity for self-organization [6]. Among the various types of ANNs, RBF (Radial Basis Function) neural network has become more and more popular in engineering applications, due to its ability to approximate complex nonlinear mappings directly from the input-output data with a simple topological structure [7]. The purpose of this paper is to establish an effective method for fault diagnosis of urban rail vehicle auxiliary inverter based on wavelet packet and RBF neural network. The feasibility of this method is demonstrated through the data of voltage signal. These results reported in this paper clearly demonstrate the accuracy of implementing this approach for urban rail vehicle auxiliary inverter.
The study is outlined as follows. In Section 2, the wavelet and wavelet packet analyses are introduced. In Section 3, we introduce the wavelet packet energy vector algorithm. Section 4 defines the RBF neural network model. Section 5 demonstrates the effectiveness of the proposed wavelet packet and RBF neural network algorithm for faults diagnosis of urban rail vehicle auxiliary inverter. Finally, the conclusions are drawn in Section 6.
2. Wavelet and Wavelet Packet Analysis
If
and its Fourier transform,
, satisfy the admissibility condition [8]
(1)
A wavelet
is a function of zero average
(2)
is a wavelet function and
is the space of square integrable complex functions. The corresponding family of wavelets consists of a series of son wavelets, which are generated by dilation and translation from the mother wavelet
, is shown as follows:
(3)
where a and b are the dilation and translation parameters, respectively.
Wavelet packet analysis decomposes signals not only into low-frequency signals, but also into a high-frequency part. WPD can adaptively select the appropriate frequency spectrum to match the signal spectrum from the analysis of the signal characteristics and requirements, it is relatively refined method. As shown in Figure 1, it is the diagram of wavelet packet decomposition. S represents original signal,
represents the 1st low frequency coefficient
, which decomposed by wavelet packet,
represents the 1st low frequency coefficient
, others is so on.
3. Wavelet Packet Energy Vector Algorithm
1) The wavelet packet is adopted to decompose the original signals.
2) The energy feature of each band
(4)
where,

represents amplitude of the reconstructed signal.
3) The wavelet packet energy eigenvector.
The definition of all the energy of signal:
(5)
A band of relative wavelet packet energy:

Figure 1. Signal diagram of wavelet packet decomposition.
(6)
The definition of relative wavelet packet energy feature vector [9]:
(7)
4. The RBF Neural Network Model
The recent development of powerful learning algorithm for the ANN (Artificial Neural Network) has led to their utilization in many engineering applications [10-12]. Among the various types of ANNs, RBF (Radial Basis Function) neural network has become more and more popular in engineering applications. The Radial Basis Function (RBF) neural network is a kind of feed forward neural network. It has higher convergence rate, it can determine corresponding network topology structure according to the specific issues and self-study, organization, adaptive function. The neurons of RBF neural network use radial basis transfer function, with two structural layers. The first layer is implied in radial basis function layer, and the second layer is output linear layer. The mapping relationship of surface is stored in network connection weights and threshold which make RBF neural network with strong fault tolerance and functional approximation ability. The RBF neural networks typically have three layers: an input layer, a hidden layer with nonlinear RBF activation functions and a linear output layer [13]. Figure 2 shows the RBF neural network structure for fault diagnosis of auxiliary inverter.
5. Experimental Results
5.1. The Process of Wavelet Packet
Figures 3(a)-5(a) are the time domain of the signal. For wavelet packet decomposition of the original data, the decomposition structure at 3 is realized and shown in figures 3(b)-5(b). After wavelet packet decomposition, the wavelet packet energy eigenvector is constructed.
5.2. RBF Neural Network for Fault Diagnosis and Verification
To validate the approach presented in this paper, the dataset of wavelet packet energy eigenvector is divided
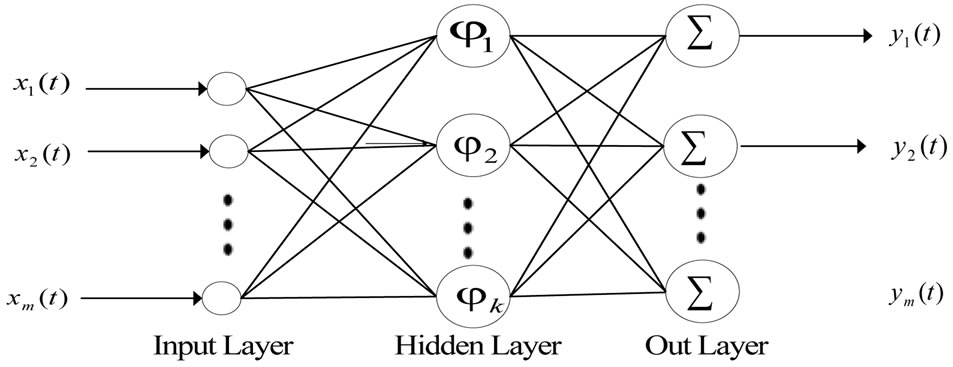
Figure 2. Framework of RBF neural network.
(a)
(b)
Figure 3. (a) The time domain of voltage fluctuation signal; (b) The reconstructed waveform of voltage fluctuation signal.
(a)
(b)
Figure 4. (a) The time domain of power interruption signal; (b) The reconstructed waveform of power interruption signal.
(a)
(b)
Figure 5. (a) The time domain of frequency variation signal; (b) The reconstructed waveform of frequency variation signal.

Table 1. Sample data of urban rail vehicle auxiliary inverter operation.

Table 2. testing data.

Table 3. testing data.
into two datasets to be used for training and testing. The training set is used for determining the RBF neural network parameters. The testing dataset is set for validating the performance of the trained model. The training group is shown in table 1, the testing group is shown in table 2. After many times of experiments, the hidden layer adopts 8 neurons with faster speed and better learning effect, so the structure of RBF neural network is 8-8-3. The input layer adopts 8 corresponding to the 8 different ranges of the frequency spectrum of a fault signal, 3 outputs corresponding to 3 respective signals, such as voltage fluctuation signal, impulsive transient signal and Frequency variation signal. The test results of this case are shown in table 3.
6. Conclusions
In this paper, an effective method for fault diagnosis of urban rail vehicle auxiliary inverter based on wavelet packet and RBF neural network is presented.
1) The wavelet packet method is an expansion of classical wavelet decomposition that presents more possibilities for signal processing.
2) RBF neural network has higher convergence rate and better robustness. It can determine corresponding network topology structure according to the specific issues and self-study, organization and adaptive function.
The experimental results show that the proposed method gives an effective and attractive approach for the fault diagnosis of the urban rail vehicle auxiliary inverter.
7. Acknowledgements
This paper was supported by the National Key Technology R&D Program in the 11th Five year Plan of china (2011BAG01B05), the State Key Laboratory of Rail Traffic Control and Safety (No. RCS2010ZZ002), Beijing Jiaotong University and Doctor Foundation of Shandong Province (BS2011DX008).