Theories on the Relationship between Price Process and Stochastic Volatility Matrix with Compensated Poisson Jump Using Fourier Transforms ()
1. Introduction
Volatility measures uncertainty of returns which plays a major role in cash flows from selling assets at a precise future date. It is very essential in financial markets due to price fluctuations, prediction of stock prices, option pricing, portfolio management and hedging. Decision and policy makers depend on volatility to determine the bullish and bearish nature of the market to avoid loss. The varying nature of volatility makes it difficult to predict stock prices. The Black-Scholes framework assumes constant volatility but empirical evidence has proved otherwise leading researchers to explore more into modeling volatility of an asset. It is important to note that the original Black-Scholes framework did not include jumps into price processes. It is then of interest to explore to what extend the inclusion of jumps affects the dynamics of stock price volatility. Volatility can be estimated through parametric and nonparametric methods. When using the parametric methods, it is modeled using its functional form of observed variables in the market. These include discrete-time volatility models such as Autoregressive Conditional Heteroscedasticity(ARCH) models, where volatility relies on the past returns and other variables that are directly observed only [1] . Also, the implied volatilities are based on parametric models. Com- putation of the historical volatility without assuming its functional form is done by nonparametric methods. The expost variation in the frequency domain of equity prices was analyzed by Wang [2] . He proposed a realized periodogram based estimator based on Fourier transform which estimated the quadratic variation consistently using the price process which was log equilibrium. For contaminated prices caused by the micro-structure noise from the market data, the estimator filtered out the high frequency periodograms which converted the high frequency data to a low frequency periodograms. Its application was done to electric prices transaction that was general through simulation. The proposed estimator was said to be insensitive to sample frequency choices.
Malliavin and Mancino [3] presented the computation of a time series volatility using Fourier series analysis method from observations of a semi- martingale data. The method was nonparametric and model free. The Fourier coefficient of the volatility was estimated based on integration of the time series. They stated that the method was well suitable for financial market and specifi- cally for analysis of time series data which are of high frequency and cross volati- lities computations. Kanatani [4] derived a linear interpolation bias of realized volatility. He used Fourier series estimator proposed by Malliavin and Mancino [3] to avoid the biasness. He examined the theoretical relationship between realized volatility and Fourier estimator and showed that the Fourier estimator was most efficient than the realized volatility. He also proposed that, linear interpolation should not be used as the preparation for realized volatility calcula- tions. Hoshikawa et al. [5] compared alternative estimators theoretically and empirically for the performance of the classical quadratic variation method by Hayashi and Yoshida [6] and Fourier series estimator by Malliavin and Mancino [3] in the presence of high frequency data. They found out that, the Hayashi and Yoshida [6] estimator performed the best among the alternatives in view of the bias and the Mean Square Error for integrated multivariate volatility since the biasness of Hayashi and Yoshida [6] was mostly due to the biasness of the drift. The other estimators were shown to have possibly heavy bias mostly toward the origin. They also applied these estimators to Japanese Government Bond futures to obtain the results which was consistent with their simulation. Mancino and Sanfelici [7] studied the Fourier estimator of an integrated volatility in the presence of micro-structure noise. They presented the properties of finite sample of the Fourier estimator and derived an analytic expression for the contaminated estimator in terms of biasness and Mean Square Error. They also revealed that, the estimate could be used experimentally to design an optimal Mean Square Error-based estimator, which was efficient and robust with noise. Their conclu- sion was that, the Fourier estimator was relatively unbiased and the biasness of the finite sample could be made small by appropriately cutting off the highest frequencies. Mattiussi and Iori [8] analyzed a method based on Fourier analysis to estimate volatility and correlation when the observed prices are at a high frequency rate. Their method did not require data manipulation and led to more robust estimates than the traditional methods that have been proposed. They evaluated the performance of the Fourier algorithm to reconstruct the time volatility of simulated bivariate and univariate models. They also used the Fourier method to investigate the volatility and the correlation dynamics of the future market over the Asian crisis period. They detected possible interdepen- dencies and volatility transmission. A nonparametric estimation method based on Fourier analysis applied to continuous semi-martingales was proposed by Malliavin and Mancino [9] . It was mainly constructed for measuring instanta- neous univariate and multivariate volatility and co-volatility using observations from high frequency data. The Fourier transform of log-returns took into account asynchronous observations and unevenly spaced data. Asymptotic nor- mality and the consistency of the statistical properties of the Fourier estimator was analyzed. They defined the Fourier estimator purposely for co-volatility computation without manipulating the data based on integration embedded in Fourier transform. Also, with high frequency data, the reconstruction of co-vo- latility as a stochastic function was done in an effective way which allowed the volatility function to be handled as a variable observed in financial applications. Cuchiero and Teichmann [10] presented a new nonparametric method to compute the trajectory of instantaneous covariance using Fourier transform. The observation used was discrete from a multidimensional price process in the presence of jump. They extended the work of Malliavin and Mancino [3] , [9] by adding a classical jump-robust estimator of a realized covariance estimation integration to estimate the Fourier coefficient. The path of the instantaneous covariance was reconstructed using Fourier-Fejer inversion. They proved the central limit theorem and the consistency of the estimator. Also, they analyzed the asymptotic estimator variance which was smaller by a factor 2/3 as compared to the classical local estimators. They investigated its robustness and showed how to empirically and theoretically estimate the integrated realized covariance of a stochastic covariance process which was instantaneous. Barucci et al. [11] studied the performance of forecasting the volatility estimator using Fourier with micro-structure noise presence. They compared analytically the Fourier estimator which significantly performed better than the realized volatility esti- mator types, especially for high-frequency data and without noise component. They showed that the Fourier estimator outperformed the other methods designed for handling the market micro-structure contamination.
All these and many researches have been done in volatility estimation using Fourier analysis without the consideration of the addition of a compensated Poisson jump. In this paper, we are motivated to propose a nonparametric estimation method based on Fourier analysis incorporated with Bohr’s convolu- tion and quadratic variation, applying it to continuous semi-martingale process with the addition of a compensated Poisson jump as an extension of the results of Malliavin and Mancino [9] . We investigate to see whether the addition of compensated Poisson jump has effect on the results obtained. This method will be suitable for measuring instantaneous multivariate volatility. This process will incorporate discontinuities on the returns on stocks and help fit a better market data with regards to the reflection of the reality in the stock market [12] . The estimation of volatility with a compensated Poisson jump will give a fair idea on how the movement of the stock prices will be in order for investors to position themselves well in terms of investing or hedging.
The volatility is reconstructed as a function of time. The volatility matrix
on the time window
is computed by changing the origin of time, rescaling it to reduce the time window to
and using Fourier transform with Bohr’s convolution and quadratic variation to estimate it. We prove a general identity relating the Fourier transform of the price process
with a compensated Poisson jump to that of the instantaneous multivariate volatility
under the hypothesis that the volatility process is square integrable. We then derive an instantaneous volatility estimator from the identity based on a discrete, unevenly spaced asset prices. This is important when the derivation of stochastic volatility is performed along the time evolution in terms of contingent claim pricing-hedging [13] . This method can be generalized to measure the cross-correlations or co-variances which is a multivariate case. The paper is organized as follows: Section 2 deals with theoretical concepts. Section 3 dis- cusses the estimation of stochastic volatility using Fourier Transform and Sec- tion 5 presents the conclusion.
2. Theoretical Concepts
We present some important mathematical preliminaries that are significant to this paper. These preliminaries will outline some definitions and theorems that will be used in this paper.
Definition 1. A stochastic process
is a counting process if there exists an increasing family of sequence of random variables
where
is finite and
and
[14]
Definition 2. Suppose
is a metric space of a real line with metric
then a continuous real-valued function
on
is called an almost periodic function if, for every
, there exists
such that every interval
contains at least one number
for which
for
. [15]
Definition 3. Let
be functions on
, where
is an integer, then their Bohr convolution is:
Definition 4. The map
from
to
is an Itô map where
is the set of continuous maps from
to
and
is the set of continuous maps
, from
to
such that
and stochastic moving frame
such that
and
is the identity [16] .
Definition 5. Let
be an n-dimensional Riemannian Manifold,
be a tangent space and
be the dual space of
then a vector field
along
is a map from
to
such that for
,
. If
is an adapted vector field with respect to
being an adapted differential one form along
, then
with respect to
is an adapted vector on
[16] .
Definition 6. Suppose
is the dual space of
then a differential form
of degree one along
is a map from
to
such that for any
,
. If
is an adapted differential one form along
, then
is in a linear form [16] .
Theorem 1. (Ito energy identity). Let
be an adapted differential form and
be an adapted vector field along
; then it implies that we have
(1)
Proof: see [16] .
Theorem 2. (Ito Formula for a complex variable case). If
, where
are martingales and we apply complex Itô formula to it, then we get,
(2)
Proof: see [17] .
Theorem 3. (Burkholder-Davis-Gundy inequality) For every
there exist positive constants
such that, for all local martingales
with
and stopping times
, the following inequality holds.
If
, then we have a special case, then it implies that this inequality holds in this case with constants
.
See proof [18] .
Remark 1. The compensated Poisson process
,
is a martingale with respect to its own filtration
[14] .
Definition 7. Let
be a real-valued stochastic process defined on the probability space
and with time
that ranges over non-negative real numbers then the pth variation is defined as,
where
and
is the norm of the partition
such that we have
if the above sum converges [19] .
This is true under certain conditions for example,
defines the first variation or total variation process, for
, the pth variation equals the quadratic variation if the sum converges. Also, it is a bounded variation if and only if for
.
For a generalize Itô processes,
where
is a standard Brownian motion, its quadratic variation is given by
[20] .
The quadratic variation of a compensated Poisson process
is
[21] .
3. Stochastic Volatility Estimation with Fourier Transforms
Fourier Transform is a nonparametric method which is the representation of frequency domain, and the mathematical operation that links the frequency domain representation to a function of time. A time varying data can be transformed from one domain into a different domain (frequency domain) and that is the main idea behind Fourier [22] . To represent functions in Fourier transform, the function has to be non-periodic and its integral of the absolute of the function must converge [23] . A function can be reconstructed from its Fourier transform by using inverse Fourier transform. When volatility changes with time, its computation which is done by nonparametric methods centers around small time windows which can be daily, weekly or monthly in a high frequency data.
Fourier transform constructs the volatility as a function for it’s iteration and computation of the cross-correlation between price and volatility. Fourier Transform takes into consideration all the observations and avoids inconsistency in data since it is based on integration. Let
be the log-price of assets which is a continuous semi-martingale on a fixed time window, then
where
and
is a Poisson process with intensity
,
is the drift,
is the volatility, time is
and the standard Brownian motion
.
and
are independent.
We solve for the price process with a compensated Poisson jump as
(3)
where
is adapted to a filtration but
is not necessarily adapted and it’s bounded by
, for
.
Now we let
, satisfying
(4)
where
are independent Brownian motions on a probability space, such that
and
are random processes which are adapted to a filtration and satisfies the conditions in (QA) below;
(QA)
Definition 8. Suppose we have two assets whose prices are
, then its respective volatilities will be
(co-volatilities), hence the entries of its volatility matrix
, is
When
, the volatility matrix will be
known as the instantaneous volatilities.
Theorem 4. Suppose the function
has Fourier transform:
(5)
and its differential form:
(6)
then
(7)
Proof. From Equation (5),
From Equation (6)
but
, and
,
, then we have
that is
The Identity Relation for a Complex Martingale Case
We present the following propositions with their proofs below.
Proposition 5. The identity that relates the price process and volatility matrix with the compensated Poisson jump is
(8)
Proof. Here we establish an identity which relates the Fourier transform of the price process
to the Fourier transform of the volatility matrix
. The drift
does not contribute to the quadratic variation [24] , so without loss of generality, we let
; then
is a semi-martingale.
Suppose we have a price process which has a volatility matrix and a com- pensated Poisson jump,
(9)
where
does not depend on
then from Equation (9), we have,
let
then,
Again, let
,
and
then it suffices from the theory of convolution that,
Since
then from Bohr’s convolution, the functions that convolve with
will be zero and also functions that convolve with
apart from itself will be zero, hence we have,
From Definition 3, we have,
(10)
From Equation (10), we define the Bohr’s convolution of the volatility and the compensated Poisson jump as:
which implies that
But
We define
[2]
which implies
where
is:
Let
and
Then we have,
Hence the identity that relates the volatility matrix and the compensated Poisson jump is
Proposition 6. The volatility matrix and compensated Poisson jump are related to the price process by the identity
(11)
with the volatility independent of the stock’s Brownian motion.
Proof. In the case where
is independent of
, we introduce complex martingales
for any integers
, where
is the price process and we have two assets,
The Fourier transform of the complex martingale is,
That is:
which implies
Using Itô formula in Theorem 2 to solve the complex martingale we have,
From Equation (4), if
we have
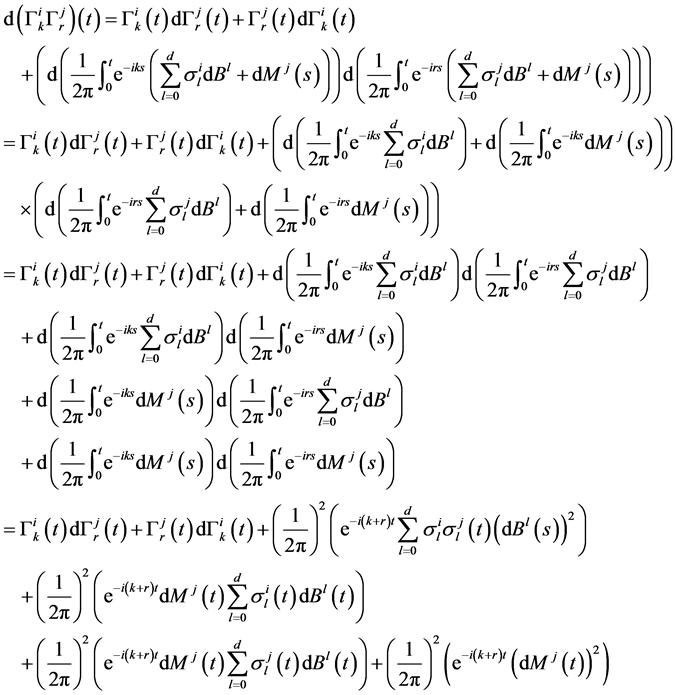
But
and
, which implies,
Since
and
we have,
Let
and from the definition of a Fourier transform,
we have
which follows that,
If we have an integer
, then for any integer q, where
and from the Bohr’s convolution theory we have,
(12)
which simplifies to
From Equation (12) it implies that,
Hence,
(13)
where
but
which implies
and
can also be reduced to
by symmetry as,
[9]
From
,
Let
(it refers to the Fourier transform of the initial price) then it implies that
Now
Since
and
, we have
Hence
can be reduced to
by symmetry as
.
Let
be a Dirichlet kernel defined as
(14)
By the definition of
we have,
But from Equation (14)
which implies
Hence,
From Equation (4), when
and we have two assets then,
Let
(15)
and
(16)
then using Itô energy identity Equation (1) we have,
(17)
Expressing Equation (17) using Cauchy-Schwarz inequality we have
But
then eva- luating the rest of the terms using Burkholder-Gundy’s inequality, we have
and
takes the interval
and since the maximum of
is 1, we have
Similarly for
we have
Let
, then by change of variables we have,
,
,
,
We have
, and
This implies that as
,
.
. If
, then
.
Evaluating
;
but
Hence,
We express
as
When
, we have
Let
We have,
Taking the square of both sides
From De-moivre’s formula,
it im- plies
but
. It follows that,
Now,
From Equation (12),
This implies
where
is defined as
Theorem 7. Suppose
is the price process satisfying Equation(3), then the instantaneous volatility function with a compensated Poisson jump is
where
is the volatility of the price process
at time
and
are integers.
Proof. From Equation (7)
then it implies that
(18)
Then from Proposition 2 and 3, the identity that relates the Fourier transform of the price process with a compensated Poisson jump and the volatility is simplified as,
But,
then it implies that
The Fourier-Fejer summation function for
which is continuous is given as;
From Definition 8, to obtain the instantaneous volatility function
, we extract the diagonals of the matrix and sum them, that is when
,
, which gives the Fourier estimator of the instantaneous volatility with a compensated Poisson jump as,
where
Comparing the results obtained in Theorem 2.1 of Malliavin and Mancino’s [9] article, for a process
where
satisfying
and the conditions in (QA), the identity that relates the price process and the volatility matrix was
In comparison with the one obtained in this paper, the identity that relates the price process and the volatility matrix with the addition of compensated Poisson jump is
Also, the instantaneous multivariate volatility obtained by Malliavin and Man- cino [9] was
and when compensated Poisson jump was added to it we obtained
This means that the addition of compensated Poisson jump had an effect on the volatility of the price process.
4. Stochastic Volatility Estimation for a Specific Case
Suppose the price process follows the process,
then its Fourier transform is
but
, then,
Let
, then from the theory of convolution,
From Equation (10), the Bohr’s convolution of the jump diffusion process is:
Then,
, then,
Then we have,
which gives the identity relating the price process to the volatility matrix and the jump diffusion process. It implies that,
Then,
Its Fourier-Fejer summation function is given as;
Its instantaneous volatility function
is given as
For one asset we have,
(19)
where
Numerical Example
When
,
but
and
has a jump height of 1 and 0 otherwise and
, then we have,
From Definition 1, without making any assumptions in the jump, if
then
but if
, then
where
This example shows that the price process is directly proportional to the volatility meaning that with all other parameters constant, an increase in volatility,
will increase the price process and vice versa.
Comparing the result obtained in Theorem 7 to Equation (19), the parameter
added to the jump component had an effect on the volatility. An increase or decrease in the parameter
will affect the volatility in direct pro- portionality.
5. Conclusion and Future Work
We have shown the theoretical basis for estimating stochastic volatility with the presence of a compensated Poisson jump. This has been achieved by use of Fourier transforms incorporating Bohr’s convolution. We further established an identity relation for the Fourier transform of the price process with a compen- sated Poisson jump and the volatility, and also estimated the instantaneous volatility. We have also shown the identity relation for a specific case when
was added to the jump and concluded that it was directly proportional to the volatility with all other parameters constant. This estimate can be used for both univariate and multivariate volatility settings. As a future work, it will be interesting to know how other types of jump diffusion processes together with microstructure noise effect the dynamics of volatility in the financial markets.
Acknowledgements
This work was carried out with financial support from the government of Canada’s International Development Research Centre (IDRC), and within the framework of AIMS Research for Africa Project. Also our gratitude to Dr. Edward Prempeh, Department of Mathematics(KNUST), University of Cape Town, African Institute for Mathematical Science, Ghana and the reviewers.