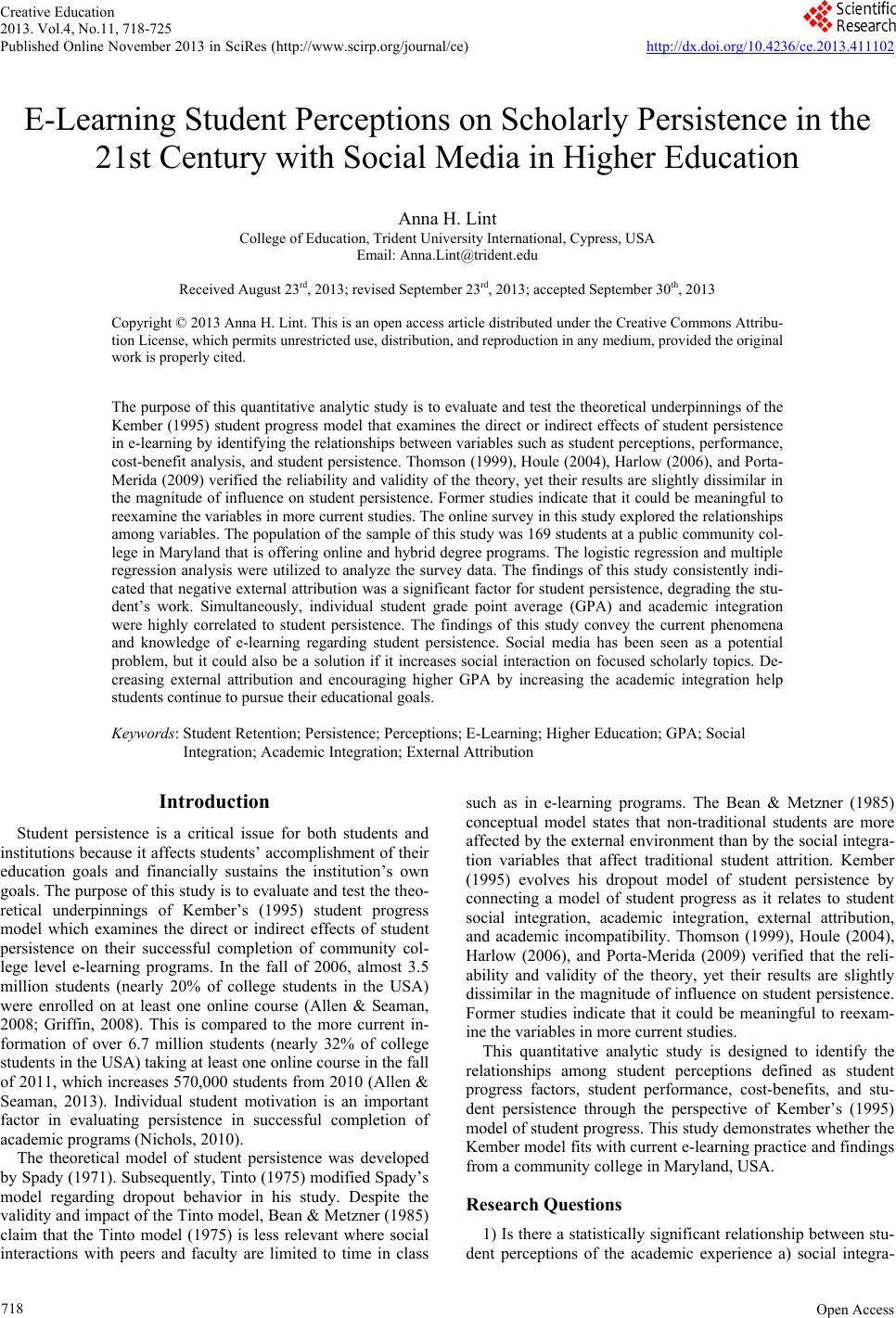 Creative Education 2013. Vol.4, No.11, 718-725 Published Online November 2013 in SciRes (http://www.scirp.org/journal/ce) http://dx.doi.org/10.4236/ce.2013.411102 Open Access 718 E-Learning Student Perceptions on Scholarly Persistence in the 21st Century with Social Media in Higher Education Anna H. Lint College of Education, Trident University International, Cypress, USA Email: Anna.Lint@trident.edu Received August 23rd, 2013; revised September 23rd, 2013; accepted September 30th, 2013 Copyright © 2013 Anna H. Lint. This is an open access article distributed under the Creative Commons Attribu- tion License, which permits unrestricted use, distribution, and reproduction in any medium, provided the original work is properly cited. The purpose of this quantitative analytic study is to evaluate and test the theoretical underpinnings of the Kember (1995) student progress model that examines the direct or indirect effects of student persistence in e-learning by identifying the relationships between variables such as student perceptions, performance, cost-benefit analysis, and student persistence. Thomson (1999), Houle (2004), Harlow (2006), and Porta- Merida (2009) verified the reliability and validity of the theory, yet their results are slightly dissimilar in the magnitude of influence on student persistence. Former studies indicate that it could be meaningful to reexamine the variables in more current studies. The online survey in this study explored the relationships among variables. The population of the sample of this study was 169 students at a public community col- lege in Maryland that is offering online and hybrid degree programs. The logistic regression and multiple regression analysis were utilized to analyze the survey data. The findings of this study consistently indi- cated that negative external attribution was a significant factor for student persistence, degrading the stu- dent’s work. Simultaneously, individual student grade point average (GPA) and academic integration were highly correlated to student persistence. The findings of this study convey the current phenomena and knowledge of e-learning regarding student persistence. Social media has been seen as a potential problem, but it could also be a solution if it increases social interaction on focused scholarly topics. De- creasing external attribution and encouraging higher GPA by increasing the academic integration help students continue to pursue their educational goals. Keywords: Student Retention; Persistence; Perceptions; E-Learning; Higher Education; GPA; Social Integration; Academic Integration; External Attribution Introduction Student persistence is a critical issue for both students and institutions because it affects students’ accomplishment of their education goals and financially sustains the institution’s own goals. The purpose of this study is to evaluate and test the theo- retical underpinnings of Kember’s (1995) student progress model which examines the direct or indirect effects of student persistence on their successful completion of community col- lege level e-learning programs. In the fall of 2006, almost 3.5 million students (nearly 20% of college students in the USA) were enrolled on at least one online course (Allen & Seaman, 2008; Griffin, 2008). This is compared to the more current in- formation of over 6.7 million students (nearly 32% of college students in the USA) taking at least one online course in the fall of 2011, which increases 570,000 students from 2010 (Allen & Seaman, 2013). Individual student motivation is an important factor in evaluating persistence in successful completion of academic programs (Nichols, 2010). The theoretical model of student persistence was developed by Spady (1971). Subsequently, Tinto (1975) modified Spady’s model regarding dropout behavior in his study. Despite the validity and impact of the Tinto model, Bean & Metzner (1985) claim that the Tinto model (1975) is less relevant where social interactions with peers and faculty are limited to time in class such as in e-learning programs. The Bean & Metzner (1985) conceptual model states that non-traditional students are more affected by the external environment than by the social integra- tion variables that affect traditional student attrition. Kember (1995) evolves his dropout model of student persistence by connecting a model of student progress as it relates to student social integration, academic integration, external attribution, and academic incompatibility. Thomson (1999), Houle (2004), Harlow (2006), and Porta-Merida (2009) verified that the reli- ability and validity of the theory, yet their results are slightly dissimilar in the magnitude of influence on student persistence. Former studies indicate that it could be meaningful to reexam- ine the variables in more current studies. This quantitative analytic study is designed to identify the relationships among student perceptions defined as student progress factors, student performance, cost-benefits, and stu- dent persistence through the perspective of Kember’s (1995) model of student progress. This study demonstrates whether the Kember model fits with current e-learning practice and findings from a community college in Maryland, USA. Research Questions 1) Is there a statistically significant relationship between stu- dent perceptions of the academic experience a) social integra-
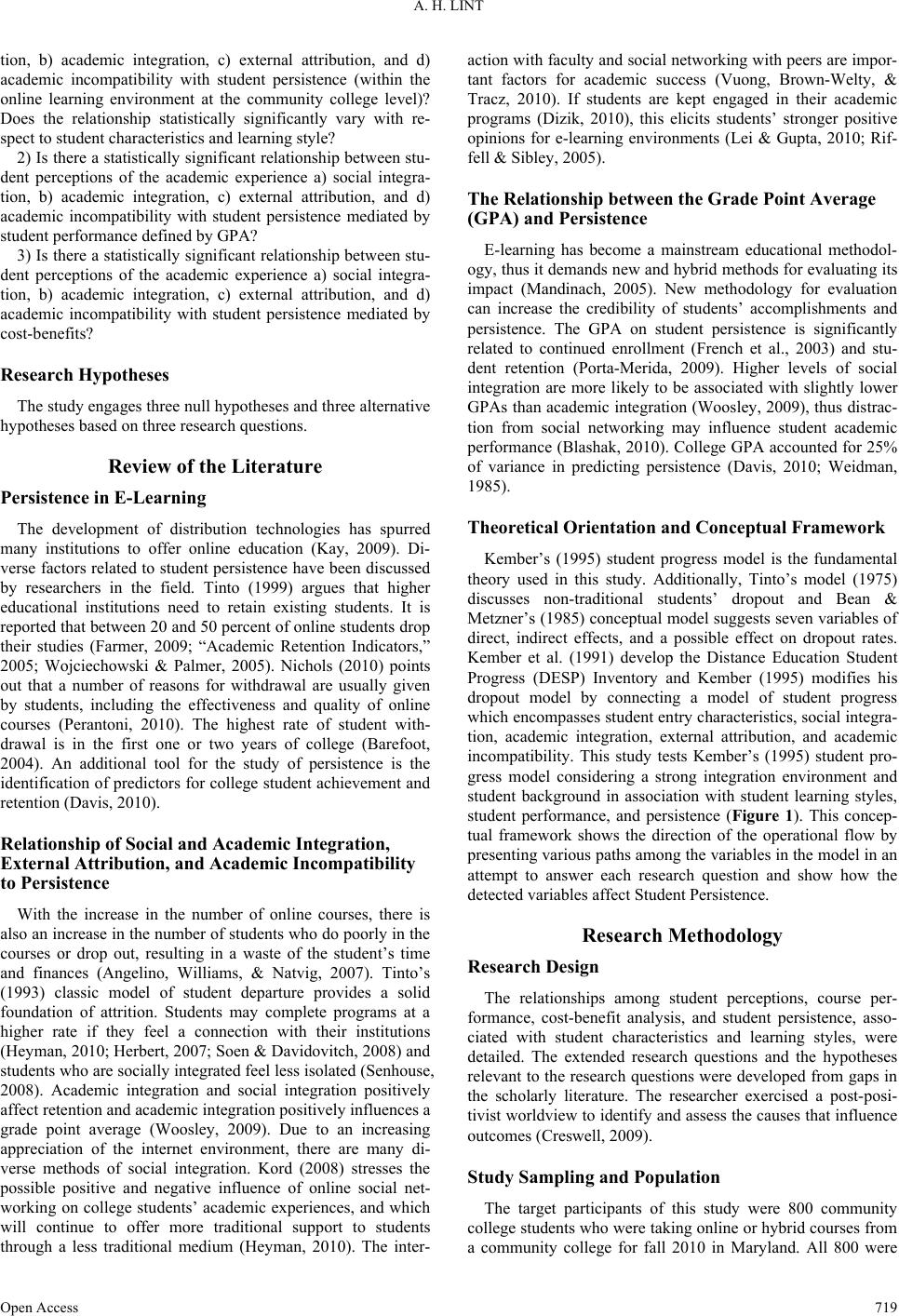 A. H. LINT tion, b) academic integration, c) external attribution, and d) academic incompatibility with student persistence (within the online learning environment at the community college level)? Does the relationship statistically significantly vary with re- spect to student characteristics and learning style? 2) Is there a statistically significant relationship between stu- dent perceptions of the academic experience a) social integra- tion, b) academic integration, c) external attribution, and d) academic incompatibility with student persistence mediated by student performance defined by GPA? 3) Is there a statistically significant relationship between stu- dent perceptions of the academic experience a) social integra- tion, b) academic integration, c) external attribution, and d) academic incompatibility with student persistence mediated by cost-benefits? Research Hypotheses The study engages three null hypotheses and three alternative hypotheses based on three research questions. Review of the Literature Persistence in E-Learning The development of distribution technologies has spurred many institutions to offer online education (Kay, 2009). Di- verse factors related to student persistence have been discussed by researchers in the field. Tinto (1999) argues that higher educational institutions need to retain existing students. It is reported that between 20 and 50 percent of online students drop their studies (Farmer, 2009; “Academic Retention Indicators,” 2005; Wojciechowski & Palmer, 2005). Nichols (2010) points out that a number of reasons for withdrawal are usually given by students, including the effectiveness and quality of online courses (Perantoni, 2010). The highest rate of student with- drawal is in the first one or two years of college (Barefoot, 2004). An additional tool for the study of persistence is the identification of predictors for college student achievement and retention (Davis, 2010). Relationship of Soci al an d A c ademi c Integration, External Attribution, and Academic Incompatibility to Persistence With the increase in the number of online courses, there is also an increase in the number of students who do poorly in the courses or drop out, resulting in a waste of the student’s time and finances (Angelino, Williams, & Natvig, 2007). Tinto’s (1993) classic model of student departure provides a solid foundation of attrition. Students may complete programs at a higher rate if they feel a connection with their institutions (Heyman, 2010; Herbert, 2007; Soen & Davidovitch, 2008) and students who are socially integrated feel less isolated (Senhouse, 2008). Academic integration and social integration positively affect retention and academic integration positively influences a grade point average (Woosley, 2009). Due to an increasing appreciation of the internet environment, there are many di- verse methods of social integration. Kord (2008) stresses the possible positive and negative influence of online social net- working on college students’ academic experiences, and which will continue to offer more traditional support to students through a less traditional medium (Heyman, 2010). The inter- action with faculty and social networking with peers are impor- tant factors for academic success (Vuong, Brown-Welty, & Tracz, 2010). If students are kept engaged in their academic programs (Dizik, 2010), this elicits students’ stronger positive opinions for e-learning environments (Lei & Gupta, 2010; Rif- fell & Sibley, 2005). The Relationship between the Grade Point Average (GPA) and Persistence E-learning has become a mainstream educational methodol- ogy, thus it demands new and hybrid methods for evaluating its impact (Mandinach, 2005). New methodology for evaluation can increase the credibility of students’ accomplishments and persistence. The GPA on student persistence is significantly related to continued enrollment (French et al., 2003) and stu- dent retention (Porta-Merida, 2009). Higher levels of social integration are more likely to be associated with slightly lower GPAs than academic integration (Woosley, 2009), thus distrac- tion from social networking may influence student academic performance (Blashak, 2010). College GPA accounted for 25% of variance in predicting persistence (Davis, 2010; Weidman, 1985). Theoretical Orientation and Conceptual Framework Kember’s (1995) student progress model is the fundamental theory used in this study. Additionally, Tinto’s model (1975) discusses non-traditional students’ dropout and Bean & Metzner’s (1985) conceptual model suggests seven variables of direct, indirect effects, and a possible effect on dropout rates. Kember et al. (1991) develop the Distance Education Student Progress (DESP) Inventory and Kember (1995) modifies his dropout model by connecting a model of student progress which encompasses student entry characteristics, social integra- tion, academic integration, external attribution, and academic incompatibility. This study tests Kember’s (1995) student pro- gress model considering a strong integration environment and student background in association with student learning styles, student performance, and persistence (Figure 1). This concep- tual framework shows the direction of the operational flow by presenting various paths among the variables in the model in an attempt to answer each research question and show how the detected variables affect Student Persistence. Research Methodology Research Design The relationships among student perceptions, course per- formance, cost-benefit analysis, and student persistence, asso- ciated with student characteristics and learning styles, were detailed. The extended research questions and the hypotheses relevant to the research questions were developed from gaps in the scholarly literature. The researcher exercised a post-posi- tivist worldview to identify and assess the causes that influence outcomes (Creswell, 2009). Study Samplin g and Popul at ion The target participants of this study were 800 community college students who were taking online or hybrid courses from a community college for fall 200 in Maryland. All 800 were 1 Open Access 719
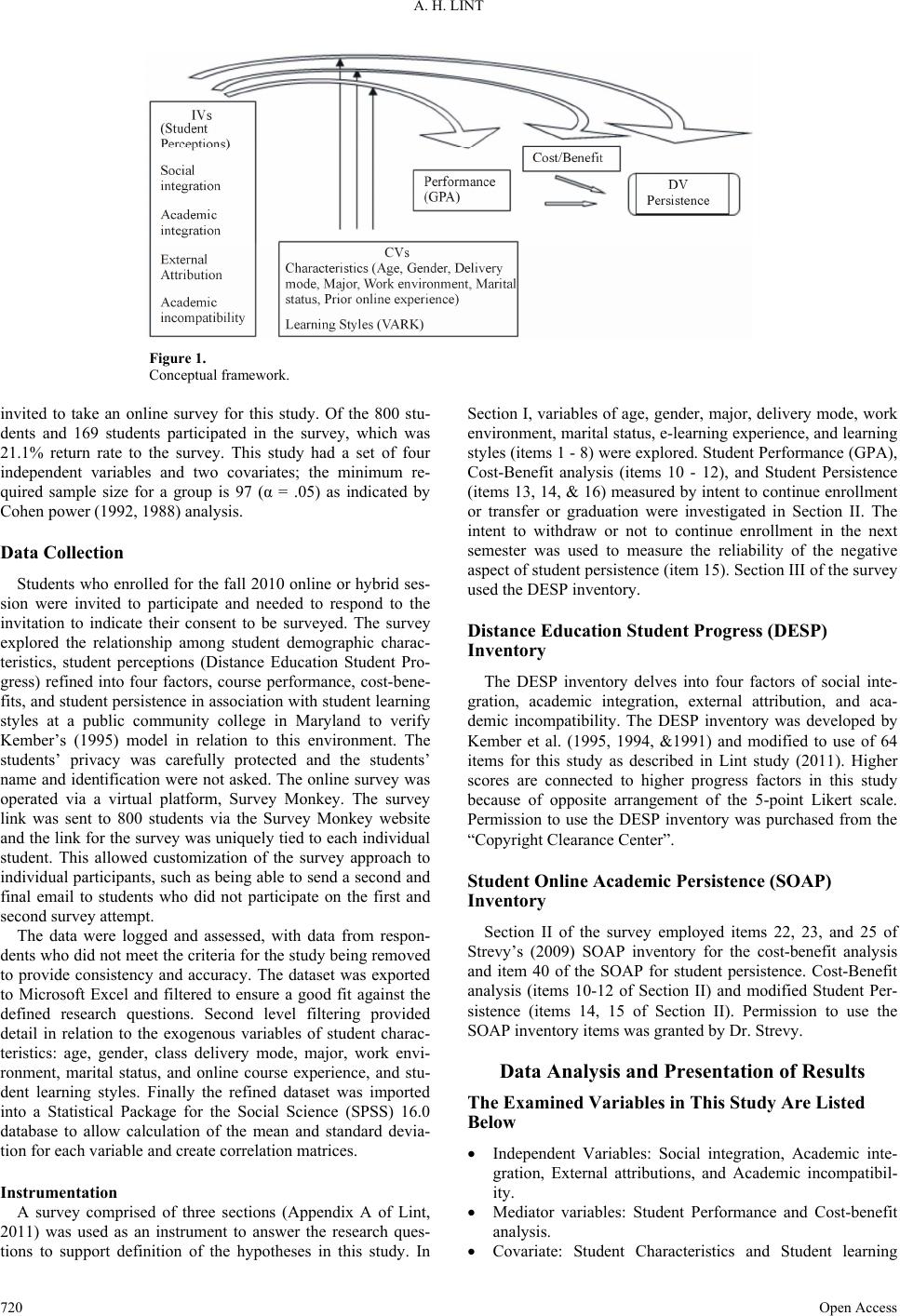 A. H. LINT Open Access 720 Figure 1. Conceptual framework. invited to take an online survey for this study. Of the 800 stu- dents and 169 students participated in the survey, which was 21.1% return rate to the survey. This study had a set of four independent variables and two covariates; the minimum re- quired sample size for a group is 97 (α = .05) as indicated by Cohen power (1992, 1988) analysis. Data Collection Students who enrolled for the fall 2010 online or hybrid ses- sion were invited to participate and needed to respond to the invitation to indicate their consent to be surveyed. The survey explored the relationship among student demographic charac- teristics, student perceptions (Distance Education Student Pro- gress) refined into four factors, course performance, cost-bene- fits, and student persistence in association with student learning styles at a public community college in Maryland to verify Kember’s (1995) model in relation to this environment. The students’ privacy was carefully protected and the students’ name and identification were not asked. The online survey was operated via a virtual platform, Survey Monkey. The survey link was sent to 800 students via the Survey Monkey website and the link for the survey was uniquely tied to each individual student. This allowed customization of the survey approach to individual participants, such as being able to send a second and final email to students who did not participate on the first and second survey attempt. The data were logged and assessed, with data from respon- dents who did not meet the criteria for the study being removed to provide consistency and accuracy. The dataset was exported to Microsoft Excel and filtered to ensure a good fit against the defined research questions. Second level filtering provided detail in relation to the exogenous variables of student charac- teristics: age, gender, class delivery mode, major, work envi- ronment, marital status, and online course experience, and stu- dent learning styles. Finally the refined dataset was imported into a Statistical Package for the Social Science (SPSS) 16.0 database to allow calculation of the mean and standard devia- tion for each variable and create correlation matrices. Instrumentation A survey comprised of three sections (Appendix A of Lint, 2011) was used as an instrument to answer the research ques- tions to support definition of the hypotheses in this study. In Section I, variables of age, gender, major, delivery mode, work environment, marital status, e-learning experience, and learning styles (items 1 - 8) were explored. Student Performance (GPA), Cost-Benefit analysis (items 10 - 12), and Student Persistence (items 13, 14, & 16) measured by intent to continue enrollment or transfer or graduation were investigated in Section II. The intent to withdraw or not to continue enrollment in the next semester was used to measure the reliability of the negative aspect of student persistence (item 15). Section III of the survey used the DESP inventory. Distance Education Student Progress (DESP) Inventory The DESP inventory delves into four factors of social inte- gration, academic integration, external attribution, and aca- demic incompatibility. The DESP inventory was developed by Kember et al. (1995, 1994, &1991) and modified to use of 64 items for this study as described in Lint study (2011). Higher scores are connected to higher progress factors in this study because of opposite arrangement of the 5-point Likert scale. Permission to use the DESP inventory was purchased from the “Copyright Clearance Center”. Student Online Academic Persistence (SOAP) Inventory Section II of the survey employed items 22, 23, and 25 of Strevy’s (2009) SOAP inventory for the cost-benefit analysis and item 40 of the SOAP for student persistence. Cost-Benefit analysis (items 10-12 of Section II) and modified Student Per- sistence (items 14, 15 of Section II). Permission to use the SOAP inventory items was granted by Dr. Strevy. Data Analysis and Presentation of Results The Examined Variables in This Study Are Listed Below Independent Variables: Social integration, Academic inte- gration, External attributions, and Academic incompatibil- ity. Mediator variables: Student Performance and Cost-benefit analysis. Covariate: Student Characteristics and Student learning
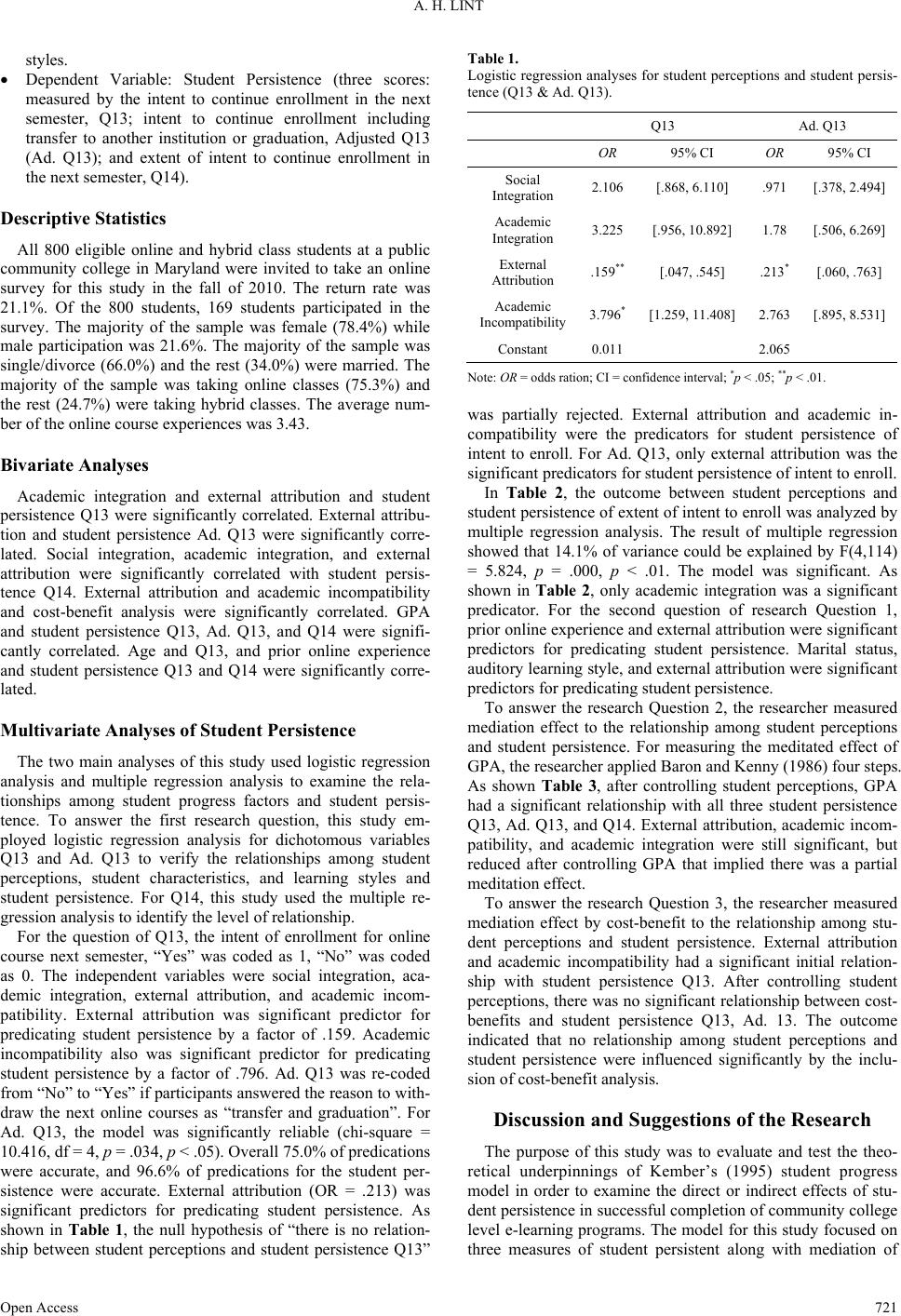 A. H. LINT styles. Dependent Variable: Student Persistence (three scores: measured by the intent to continue enrollment in the next semester, Q13; intent to continue enrollment including transfer to another institution or graduation, Adjusted Q13 (Ad. Q13); and extent of intent to continue enrollment in the next semester, Q14). Descriptive Statistics All 800 eligible online and hybrid class students at a public community college in Maryland were invited to take an online survey for this study in the fall of 2010. The return rate was 21.1%. Of the 800 students, 169 students participated in the survey. The majority of the sample was female (78.4%) while male participation was 21.6%. The majority of the sample was single/divorce (66.0%) and the rest (34.0%) were married. The majority of the sample was taking online classes (75.3%) and the rest (24.7%) were taking hybrid classes. The average num- ber of the online course experiences was 3.43. Bivariat e Analyses Academic integration and external attribution and student persistence Q13 were significantly correlated. External attribu- tion and student persistence Ad. Q13 were significantly corre- lated. Social integration, academic integration, and external attribution were significantly correlated with student persis- tence Q14. External attribution and academic incompatibility and cost-benefit analysis were significantly correlated. GPA and student persistence Q13, Ad. Q13, and Q14 were signifi- cantly correlated. Age and Q13, and prior online experience and student persistence Q13 and Q14 were significantly corre- lated. Multivariate Analyses of Student Persistence The two main analyses of this study used logistic regression analysis and multiple regression analysis to examine the rela- tionships among student progress factors and student persis- tence. To answer the first research question, this study em- ployed logistic regression analysis for dichotomous variables Q13 and Ad. Q13 to verify the relationships among student perceptions, student characteristics, and learning styles and student persistence. For Q14, this study used the multiple re- gression analysis to identify the level of relationship. For the question of Q13, the intent of enrollment for online course next semester, “Yes” was coded as 1, “No” was coded as 0. The independent variables were social integration, aca- demic integration, external attribution, and academic incom- patibility. External attribution was significant predictor for predicating student persistence by a factor of .159. Academic incompatibility also was significant predictor for predicating student persistence by a factor of .796. Ad. Q13 was re-coded from “No” to “Yes” if participants answered the reason to with- draw the next online courses as “transfer and graduation”. For Ad. Q13, the model was significantly reliable (chi-square = 10.416, df = 4, p = .034, p < .05). Overall 75.0% of predications were accurate, and 96.6% of predications for the student per- sistence were accurate. External attribution (OR = .213) was significant predictors for predicating student persistence. As shown in Table 1, the null hypothesis of “there is no relation- ship between student perceptions and student persistence Q13” Table 1. Logistic regression analyses for student perceptions and student persis- tence (Q13 & Ad. Q13). Q13 Ad. Q13 OR 95% CI OR 95% CI Social Integration 2.106[.868, 6.110] .971 [.378, 2.494] Academic Integration 3.225[.956, 10.892] 1.78 [.506, 6.269] External Attribution .159** [.047, .545] .213* [.060, .763] Academic Incompatibility 3.796*[1.259, 11.408] 2.763 [.895, 8.531] Constant 0.011 2.065 Note: OR = odds ration; CI = confidence interval; *p < .05; **p < .01. was partially rejected. External attribution and academic in- compatibility were the predicators for student persistence of intent to enroll. For Ad. Q13, only external attribution was the significant predicators for student persistence of intent to enroll. In Table 2, the outcome between student perceptions and student persistence of extent of intent to enroll was analyzed by multiple regression analysis. The result of multiple regression showed that 14.1% of variance could be explained by F(4,114) = 5.824, p = .000, p < .01. The model was significant. As shown in Table 2, only academic integration was a significant predicator. For the second question of research Question 1, prior online experience and external attribution were significant predictors for predicating student persistence. Marital status, auditory learning style, and external attribution were significant predictors for predicating student persistence. To answer the research Question 2, the researcher measured mediation effect to the relationship among student perceptions and student persistence. For measuring the meditated effect of GPA, the researcher applied Baron and Kenny (1986) four steps. As shown Table 3, after controlling student perceptions, GPA had a significant relationship with all three student persistence Q13, Ad. Q13, and Q14. External attribution, academic incom- patibility, and academic integration were still significant, but reduced after controlling GPA that implied there was a partial meditation effect. To answer the research Question 3, the researcher measured mediation effect by cost-benefit to the relationship among stu- dent perceptions and student persistence. External attribution and academic incompatibility had a significant initial relation- ship with student persistence Q13. After controlling student perceptions, there was no significant relationship between cost- benefits and student persistence Q13, Ad. 13. The outcome indicated that no relationship among student perceptions and student persistence were influenced significantly by the inclu- sion of cost-benefit analysis. Discussion and Suggestions of the Research The purpose of this study was to evaluate and test the theo- retical underpinnings of Kember’s (1995) student progress model in order to examine the direct or indirect effects of stu- dent persistence in successful completion of community college level e-learning programs. The model for this study focused on three measures of student persitent along with mediation of s Open Access 721
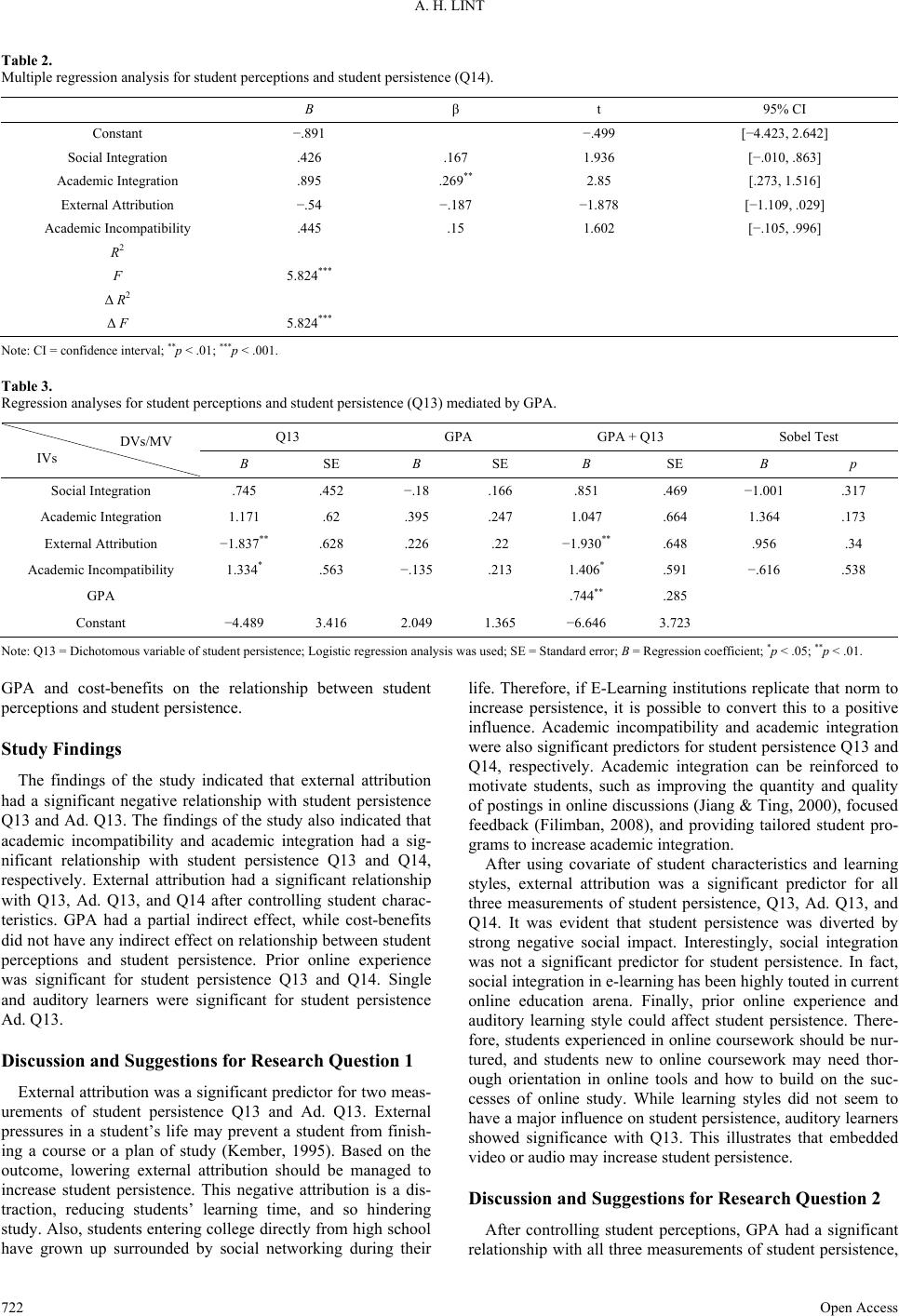 A. H. LINT Open Access 722 Table 2. Multiple regression analysis for student perceptions and student persistence (Q14). B β t 95% CI Constant −.891 −.499 [−4.423, 2.642] Social Integration .426 .167 1.936 [−.010, .863] Academic Integration .895 .269** 2.85 [.273, 1.516] External Attribution −.54 −.187 −1.878 [−1.109, .029] Academic Incompatibility .445 .15 1.602 [−.105, .996] R2 F 5.824*** Δ R2 Δ F 5.824*** Note: CI = confidence interval; **p < .01; ***p < .001. Table 3. Regression analyses for student perceptions and student persistence (Q13) mediated by GPA. Q13 GPA GPA + Q13 Sobel Test DVs/MV IVs B SE B SE B SE B p Social Integration .745 .452 −.18 .166 .851 .469 −1.001 .317 Academic Integration 1.171 .62 .395 .247 1.047 .664 1.364 .173 External Attribution −1.837** .628 .226 .22 −1.930** .648 .956 .34 Academic Incompatibility 1.334* .563 −.135 .213 1.406* .591 −.616 .538 GPA .744** .285 Constant −4.489 3.416 2.049 1.365 −6.646 3.723 Note: Q13 = Dichotomous variable of student persistence; Logistic regression analysis was used; SE = Standard error; B = Regression coefficient; *p < .05; **p < .01. GPA and cost-benefits on the relationship between student perceptions and student persistence. Study Findings The findings of the study indicated that external attribution had a significant negative relationship with student persistence Q13 and Ad. Q13. The findings of the study also indicated that academic incompatibility and academic integration had a sig- nificant relationship with student persistence Q13 and Q14, respectively. External attribution had a significant relationship with Q13, Ad. Q13, and Q14 after controlling student charac- teristics. GPA had a partial indirect effect, while cost-benefits did not have any indirect effect on relationship between student perceptions and student persistence. Prior online experience was significant for student persistence Q13 and Q14. Single and auditory learners were significant for student persistence Ad. Q13. Discussion and Suggestions for Research Questi on 1 External attribution was a significant predictor for two meas- urements of student persistence Q13 and Ad. Q13. External pressures in a student’s life may prevent a student from finish- ing a course or a plan of study (Kember, 1995). Based on the outcome, lowering external attribution should be managed to increase student persistence. This negative attribution is a dis- traction, reducing students’ learning time, and so hindering study. Also, students entering college directly from high school have grown up surrounded by social networking during their life. Therefore, if E-Learning institutions replicate that norm to increase persistence, it is possible to convert this to a positive influence. Academic incompatibility and academic integration were also significant predictors for student persistence Q13 and Q14, respectively. Academic integration can be reinforced to motivate students, such as improving the quantity and quality of postings in online discussions (Jiang & Ting, 2000), focused feedback (Filimban, 2008), and providing tailored student pro- grams to increase academic integration. After using covariate of student characteristics and learning styles, external attribution was a significant predictor for all three measurements of student persistence, Q13, Ad. Q13, and Q14. It was evident that student persistence was diverted by strong negative social impact. Interestingly, social integration was not a significant predictor for student persistence. In fact, social integration in e-learning has been highly touted in current online education arena. Finally, prior online experience and auditory learning style could affect student persistence. There- fore, students experienced in online coursework should be nur- tured, and students new to online coursework may need thor- ough orientation in online tools and how to build on the suc- cesses of online study. While learning styles did not seem to have a major influence on student persistence, auditory learners showed significance with Q13. This illustrates that embedded video or audio may increase student persistence. Discussion and Suggestions for Research Questi on 2 After controlling student perceptions, GPA had a significant relationship with all three measurements of student persistence,
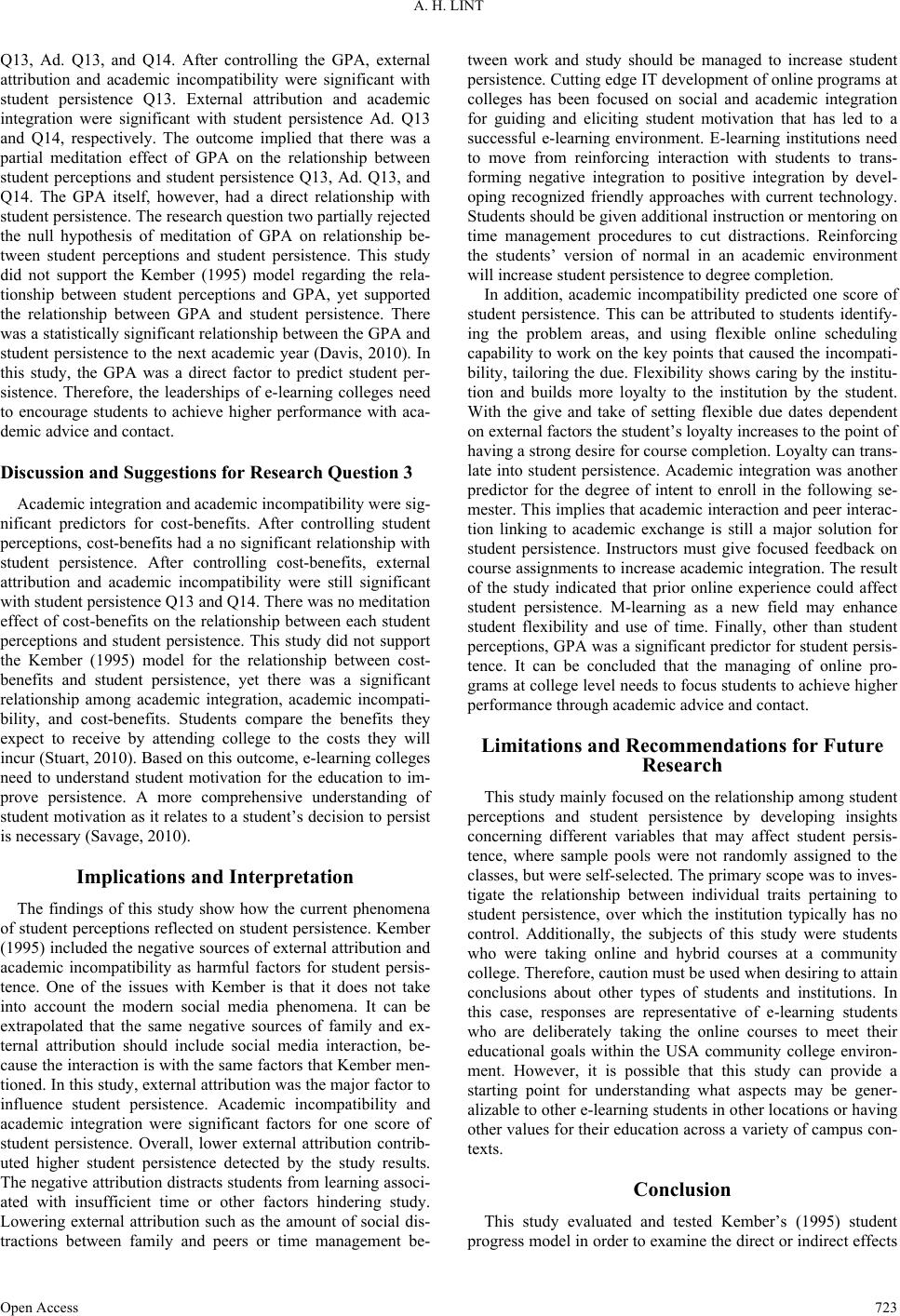 A. H. LINT Q13, Ad. Q13, and Q14. After controlling the GPA, external attribution and academic incompatibility were significant with student persistence Q13. External attribution and academic integration were significant with student persistence Ad. Q13 and Q14, respectively. The outcome implied that there was a partial meditation effect of GPA on the relationship between student perceptions and student persistence Q13, Ad. Q13, and Q14. The GPA itself, however, had a direct relationship with student persistence. The research question two partially rejected the null hypothesis of meditation of GPA on relationship be- tween student perceptions and student persistence. This study did not support the Kember (1995) model regarding the rela- tionship between student perceptions and GPA, yet supported the relationship between GPA and student persistence. There was a statistically significant relationship between the GPA and student persistence to the next academic year (Davis, 2010). In this study, the GPA was a direct factor to predict student per- sistence. Therefore, the leaderships of e-learning colleges need to encourage students to achieve higher performance with aca- demic advice and contact. Discussion and Suggestions for Research Questi on 3 Academic integration and academic incompatibility were sig- nificant predictors for cost-benefits. After controlling student perceptions, cost-benefits had a no significant relationship with student persistence. After controlling cost-benefits, external attribution and academic incompatibility were still significant with student persistence Q13 and Q14. There was no meditation effect of cost-benefits on the relationship between each student perceptions and student persistence. This study did not support the Kember (1995) model for the relationship between cost- benefits and student persistence, yet there was a significant relationship among academic integration, academic incompati- bility, and cost-benefits. Students compare the benefits they expect to receive by attending college to the costs they will incur (Stuart, 2010). Based on this outcome, e-learning colleges need to understand student motivation for the education to im- prove persistence. A more comprehensive understanding of student motivation as it relates to a student’s decision to persist is necessary (Savage, 2010). Implications and Interpretation The findings of this study show how the current phenomena of student perceptions reflected on student persistence. Kember (1995) included the negative sources of external attribution and academic incompatibility as harmful factors for student persis- tence. One of the issues with Kember is that it does not take into account the modern social media phenomena. It can be extrapolated that the same negative sources of family and ex- ternal attribution should include social media interaction, be- cause the interaction is with the same factors that Kember men- tioned. In this study, external attribution was the major factor to influence student persistence. Academic incompatibility and academic integration were significant factors for one score of student persistence. Overall, lower external attribution contrib- uted higher student persistence detected by the study results. The negative attribution distracts students from learning associ- ated with insufficient time or other factors hindering study. Lowering external attribution such as the amount of social dis- tractions between family and peers or time management be- tween work and study should be managed to increase student persistence. Cutting edge IT development of online programs at colleges has been focused on social and academic integration for guiding and eliciting student motivation that has led to a successful e-learning environment. E-learning institutions need to move from reinforcing interaction with students to trans- forming negative integration to positive integration by devel- oping recognized friendly approaches with current technology. Students should be given additional instruction or mentoring on time management procedures to cut distractions. Reinforcing the students’ version of normal in an academic environment will increase student persistence to degree completion. In addition, academic incompatibility predicted one score of student persistence. This can be attributed to students identify- ing the problem areas, and using flexible online scheduling capability to work on the key points that caused the incompati- bility, tailoring the due. Flexibility shows caring by the institu- tion and builds more loyalty to the institution by the student. With the give and take of setting flexible due dates dependent on external factors the student’s loyalty increases to the point of having a strong desire for course completion. Loyalty can trans- late into student persistence. Academic integration was another predictor for the degree of intent to enroll in the following se- mester. This implies that academic interaction and peer interac- tion linking to academic exchange is still a major solution for student persistence. Instructors must give focused feedback on course assignments to increase academic integration. The result of the study indicated that prior online experience could affect student persistence. M-learning as a new field may enhance student flexibility and use of time. Finally, other than student perceptions, GPA was a significant predictor for student persis- tence. It can be concluded that the managing of online pro- grams at college level needs to focus students to achieve higher performance through academic advice and contact. Limitations and Recommendations for Future Research This study mainly focused on the relationship among student perceptions and student persistence by developing insights concerning different variables that may affect student persis- tence, where sample pools were not randomly assigned to the classes, but were self-selected. The primary scope was to inves- tigate the relationship between individual traits pertaining to student persistence, over which the institution typically has no control. Additionally, the subjects of this study were students who were taking online and hybrid courses at a community college. Therefore, caution must be used when desiring to attain conclusions about other types of students and institutions. In this case, responses are representative of e-learning students who are deliberately taking the online courses to meet their educational goals within the USA community college environ- ment. However, it is possible that this study can provide a starting point for understanding what aspects may be gener- alizable to other e-learning students in other locations or having other values for their education across a variety of campus con- texts. Conclusion This study evaluated and tested Kember’s (1995) student progress model in order to examine the direct or indirect effects Open Access 723
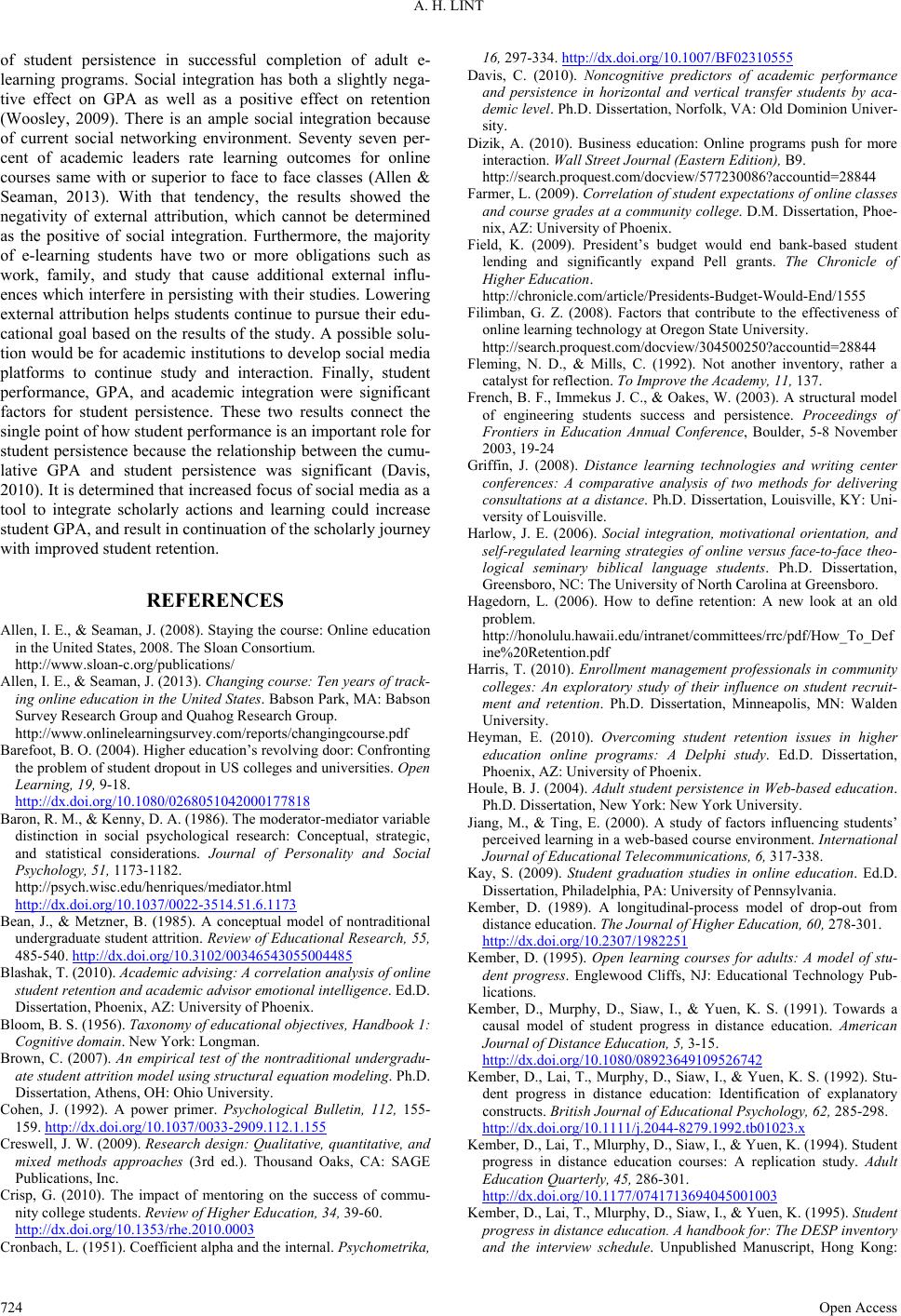 A. H. LINT of student persistence in successful completion of adult e- learning programs. Social integration has both a slightly nega- tive effect on GPA as well as a positive effect on retention (Woosley, 2009). There is an ample social integration because of current social networking environment. Seventy seven per- cent of academic leaders rate learning outcomes for online courses same with or superior to face to face classes (Allen & Seaman, 2013). With that tendency, the results showed the negativity of external attribution, which cannot be determined as the positive of social integration. Furthermore, the majority of e-learning students have two or more obligations such as work, family, and study that cause additional external influ- ences which interfere in persisting with their studies. Lowering external attribution helps students continue to pursue their edu- cational goal based on the results of the study. A possible solu- tion would be for academic institutions to develop social media platforms to continue study and interaction. Finally, student performance, GPA, and academic integration were significant factors for student persistence. These two results connect the single point of how student performance is an important role for student persistence because the relationship between the cumu- lative GPA and student persistence was significant (Davis, 2010). It is determined that increased focus of social media as a tool to integrate scholarly actions and learning could increase student GPA, and result in continuation of the scholarly journey with improved student retention. REFERENCES Allen, I. E., & Seaman, J. (2008). Staying the course: Online education in the United States, 2008. The Sloan Consortium. http://www.sloan-c.org/publications/ Allen, I. E., & Seaman, J. (2013). Changing course: Ten years of track- ing online education in the United States. Babson Park, MA: Babson Survey Research Group and Quahog Research Group. http://www.onlinelearningsurvey.com/reports/changingcourse.pdf Barefoot, B. O. (2004). Higher education’s revolving door: Confronting the problem of student dropout in US colleges and universities. Open Learning, 19, 9-18. http://dx.doi.org/10.1080/0268051042000177818 Baron, R. M., & Kenny, D. A. (1986). The moderator-mediator variable distinction in social psychological research: Conceptual, strategic, and statistical considerations. Journal of Personality and Social Psychology, 51, 1173-1182. http://psych.wisc.edu/henriques/mediator.html http://dx.doi.org/10.1037/0022-3514.51.6.1173 Bean, J., & Metzner, B. (1985). A conceptual model of nontraditional undergraduate student attrition. Review of Educational Research, 55, 485-540. http://dx.doi.org/10.3102/00346543055004485 Blashak, T. (2010). Academic advising: A correlation analysis of online student retention and academic advisor emotional intelligence. Ed.D. Dissertation, Phoenix, AZ: University of Phoenix. Bloom, B. S. (1956). Taxonomy of educational objectives, Handbook 1: Cognitive domain. New York: Longman. Brown, C. (2007). An empirical test of the nontraditional undergradu- ate student attrition model using structural equation modeling. Ph.D. Dissertation, Athens, OH: Ohio University. Cohen, J. (1992). A power primer. Psychological Bulletin, 112, 155- 159. http://dx.doi.org/10.1037/0033-2909.112.1.155 Creswell, J. W. (2009). Research design: Qualitative, quantitative, and mixed methods approaches (3rd ed.). Thousand Oaks, CA: SAGE Publications, Inc. Crisp, G. (2010). The impact of mentoring on the success of commu- nity college students. Review of Hig her Edu c a t i o n , 34, 39-60. http://dx.doi.org/10.1353/rhe.2010.0003 Cronbach, L. (1951). Coefficient alpha and the internal. Psychometrika, 16, 297-334. http://dx.doi.org/10.1007/BF02310555 Davis, C. (2010). Noncognitive predictors of academic performance and persistence in horizontal and vertical transfer students by aca- demic level. Ph.D. Dissertation, Norfolk, VA: Old Dominion Univer- sity. Dizik, A. (2010). Business education: Online programs push for more interaction. Wall Street Journal (Eastern Edition), B9. http://search.proquest.com/docview/577230086?accountid=28844 Farmer, L. (2009). Correlation of student expectations of online classes and course grades at a community college. D.M. Dissertation, Phoe- nix, AZ: University of Phoenix. Field, K. (2009). President’s budget would end bank-based student lending and significantly expand Pell grants. The Chronicle of Higher Education. http://chronicle.com/article/Presidents-Budget-Would-End/1555 Filimban, G. Z. (2008). Factors that contribute to the effectiveness of online learning technology at Oregon State University. http://search.proquest.com/docview/304500250?accountid=28844 Fleming, N. D., & Mills, C. (1992). Not another inventory, rather a catalyst for reflection. To Improve the Academy, 11, 137. French, B. F., Immekus J. C., & Oakes, W. (2003). A structural model of engineering students success and persistence. Proceedings of Frontiers in Education Annual Conference, Boulder, 5-8 November 2003, 19-24 Griffin, J. (2008). Distance learning technologies and writing center conferences: A comparative analysis of two methods for delivering consultations at a distance. Ph.D. Dissertation, Louisville, KY: Uni- versity of Louisville. Harlow, J. E. (2006). Social integration, motivational orientation, and self-regulated learning strategies of online versus face-to-face theo- logical seminary biblical language students. Ph.D. Dissertation, Greensboro, NC: The University of North Carolina at Greensboro. Hagedorn, L. (2006). How to define retention: A new look at an old problem. http://honolulu.hawaii.edu/intranet/committees/rrc/pdf/How_To_Def ine%20Retention.pdf Harris, T. (2010). Enrollment management professionals in community colleges: An exploratory study of their influence on student recruit- ment and retention. Ph.D. Dissertation, Minneapolis, MN: Walden University. Heyman, E. (2010). Overcoming student retention issues in higher education online programs: A Delphi study. Ed.D. Dissertation, Phoenix, AZ: University of Phoenix. Houle, B. J. (2004). Adult student persistence in Web-based education. Ph.D. Dissertation, New York: New York University. Jiang, M., & Ting, E. (2000). A study of factors influencing students’ perceived learning in a web-based course environment. International Journal of Educational Telecommunications, 6, 317-338. Kay, S. (2009). Student graduation studies in online education. Ed.D. Dissertation, Philadelphia, PA: University of Pennsylvania. Kember, D. (1989). A longitudinal-process model of drop-out from distance education. The Journal of Higher Education, 60, 278-301. http://dx.doi.org/10.2307/1982251 Kember, D. (1995). Open learning courses for adults: A model of stu- dent progress. Englewood Cliffs, NJ: Educational Technology Pub- lications. Kember, D., Murphy, D., Siaw, I., & Yuen, K. S. (1991). Towards a causal model of student progress in distance education. American Journal of Distance Education, 5, 3-15. http://dx.doi.org/10.1080/08923649109526742 Kember, D., Lai, T., Murphy, D., Siaw, I., & Yuen, K. S. (1992). Stu- dent progress in distance education: Identification of explanatory constructs. British Journal of Educational Psychology, 62, 285-298. http://dx.doi.org/10.1111/j.2044-8279.1992.tb01023.x Kember, D., Lai, T., Mlurphy, D., Siaw, I., & Yuen, K. (1994). Student progress in distance education courses: A replication study. Adult Education Quarterl y , 45, 286-301. http://dx.doi.org/10.1177/0741713694045001003 Kember, D., Lai, T., Mlurphy, D., Siaw, I., & Yuen, K. (1995). Student progress in distance education. A handbook for: The DESP inventory and the interview schedule. Unpublished Manuscript, Hong Kong: Open Access 724
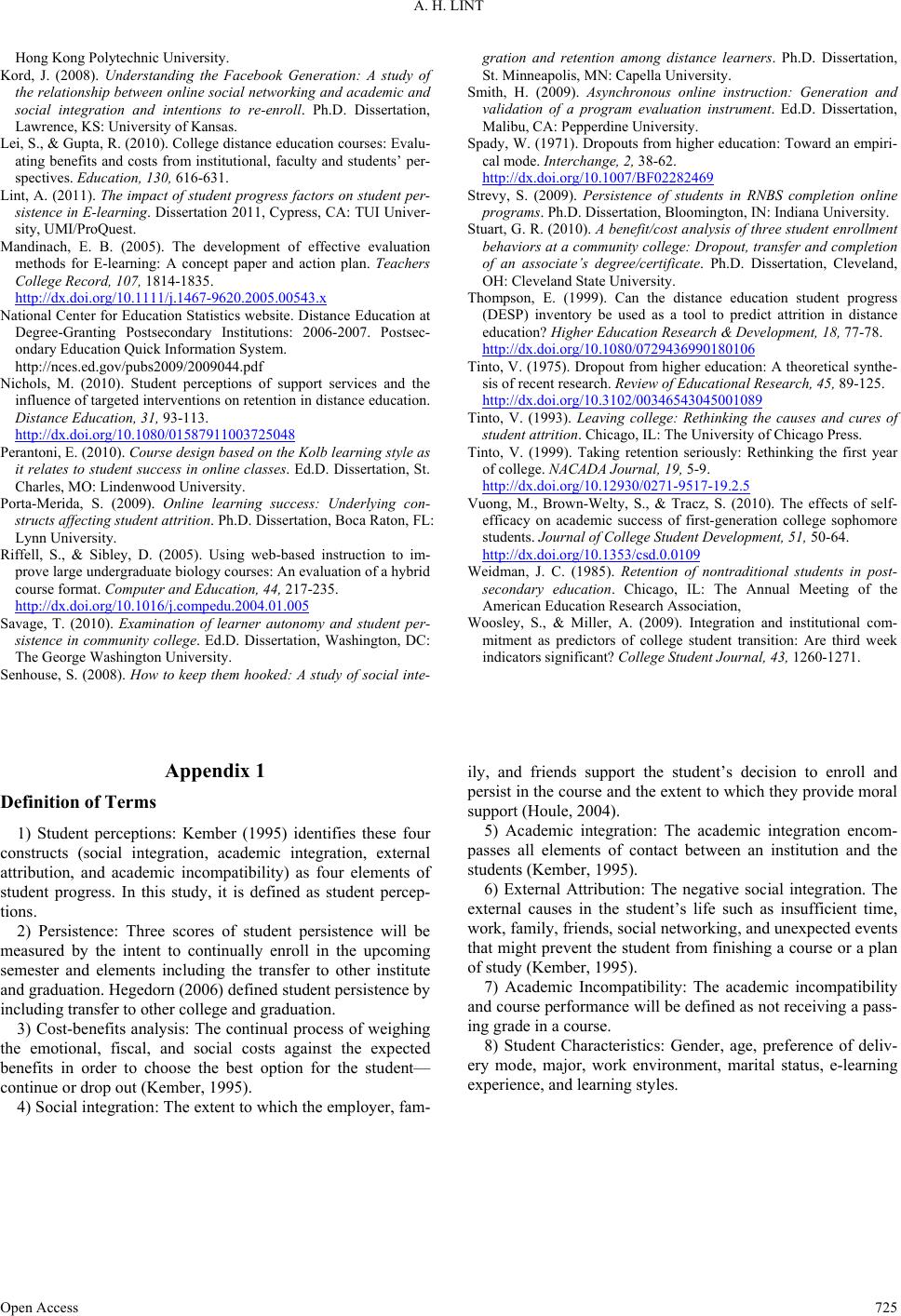 A. H. LINT Open Access 725 Hong Kong Polytechnic University. Kord, J. (2008). Understanding the Facebook Generation: A study of the relationship between online social networking and academic and social integration and intentions to re-enroll. Ph.D. Dissertation, Lawrence, KS: University of Kansas. Lei, S., & Gupta, R. (2010). College distance education courses: Evalu- ating benefits and costs from institutional, faculty and students’ per- spectives. Education, 130, 616-631. Lint, A. (2011). The impact of student progress factors on student per- sistence in E-learning. Dissertation 2011, Cypress, CA: TUI Univer- sity, UMI/ProQuest. Mandinach, E. B. (2005). The development of effective evaluation methods for E-learning: A concept paper and action plan. Teachers College Record, 107, 1814-1835. http://dx.doi.org/10.1111/j.1467-9620.2005.00543.x National Center for Education Statistics website. Distance Education at Degree-Granting Postsecondary Institutions: 2006-2007. Postsec- ondary Education Quick Information System. http://nces.ed.gov/pubs2009/2009044.pdf Nichols, M. (2010). Student perceptions of support services and the influence of targeted interventions on retention in distance education. Distance Education, 31, 93-113. http://dx.doi.org/10.1080/01587911003725048 Perantoni, E. (2010). Course design based on the Kolb learning style as it relates to student success in online classes. Ed.D. Dissertation, St. Charles, MO: Lindenwood University. Porta-Merida, S. (2009). Online learning success: Underlying con- structs affecting student attrition. Ph.D. Dissertation, Boca Raton, FL: Lynn University. Riffell, S., & Sibley, D. (2005). Using web-based instruction to im- prove large undergraduate biology courses: An evaluation of a hybrid course format. Computer and Education, 44, 217-235. http://dx.doi.org/10.1016/j.compedu.2004.01.005 Savage, T. (2010). Examination of learner autonomy and student per- sistence in community college. Ed.D. Dissertation, Washington, DC: The George Washington University. Senhouse, S. (2008). How to keep them hooked: A study of social inte- gration and retention among distance learners. Ph.D. Dissertation, St. Minneapolis, MN: Capella University. Smith, H. (2009). Asynchronous online instruction: Generation and validation of a program evaluation instrument. Ed.D. Dissertation, Malibu, CA: Pepperdine University. Spady, W. (1971). Dropouts from higher education: Toward an empiri- cal mode. Interchange, 2, 38-62. http://dx.doi.org/10.1007/BF02282469 Strevy, S. (2009). Persistence of students in RNBS completion online programs. Ph.D. Dissertation, Bloomington, IN: Indiana University. Stuart, G. R. (2010). A benefit/cost analysis of three student enrollment behaviors at a community college: Dropout, transfer and completion of an associate’s degree/certificate. Ph.D. Dissertation, Cleveland, OH: Cleveland State University. Thompson, E. (1999). Can the distance education student progress (DESP) inventory be used as a tool to predict attrition in distance education? Higher Education Research & Development, 18, 77-78. http://dx.doi.org/10.1080/0729436990180106 Tinto, V. (1975). Dropout from higher education: A theoretical synthe- sis of recent research. Review of Educational Research, 45 , 89-125. http://dx.doi.org/10.3102/00346543045001089 Tinto, V. (1993). Leaving college: Rethinking the causes and cures of student attrition. Chicago, IL: The University of Chicago Press. Tinto, V. (1999). Taking retention seriously: Rethinking the first year of college. NACADA Journal, 19, 5-9. http://dx.doi.org/10.12930/0271-9517-19.2.5 Vuong, M., Brown-Welty, S., & Tracz, S. (2010). The effects of self- efficacy on academic success of first-generation college sophomore students. Journal of College Student Development, 51, 50-64. http://dx.doi.org/10.1353/csd.0.0109 Weidman, J. C. (1985). Retention of nontraditional students in post- secondary education. Chicago, IL: The Annual Meeting of the American Education Research Association, Woosley, S., & Miller, A. (2009). Integration and institutional com- mitment as predictors of college student transition: Are third week indicators significant? College Student Journal, 43, 1260-1271. Appendix 1 Definition of Terms 1) Student perceptions: Kember (1995) identifies these four constructs (social integration, academic integration, external attribution, and academic incompatibility) as four elements of student progress. In this study, it is defined as student percep- tions. 2) Persistence: Three scores of student persistence will be measured by the intent to continually enroll in the upcoming semester and elements including the transfer to other institute and graduation. Hegedorn (2006) defined student persistence by including transfer to other college and graduation. 3) Cost-benefits analysis: The continual process of weighing the emotional, fiscal, and social costs against the expected benefits in order to choose the best option for the student— continue or drop out (Kember, 1995). 4) Social integration: The extent to which the employer, fam- ily, and friends support the student’s decision to enroll and persist in the course and the extent to which they provide moral support (Houle, 2004). 5) Academic integration: The academic integration encom- passes all elements of contact between an institution and the students (Kember, 1995). 6) External Attribution: The negative social integration. The external causes in the student’s life such as insufficient time, work, family, friends, social networking, and unexpected events that might prevent the student from finishing a course or a plan of study (Kember, 1995). 7) Academic Incompatibility: The academic incompatibility and course performance will be defined as not receiving a pass- ing grade in a course. 8) Student Characteristics: Gender, age, preference of deliv- ery mode, major, work environment, marital status, e-learning experience, and learning styles.
|