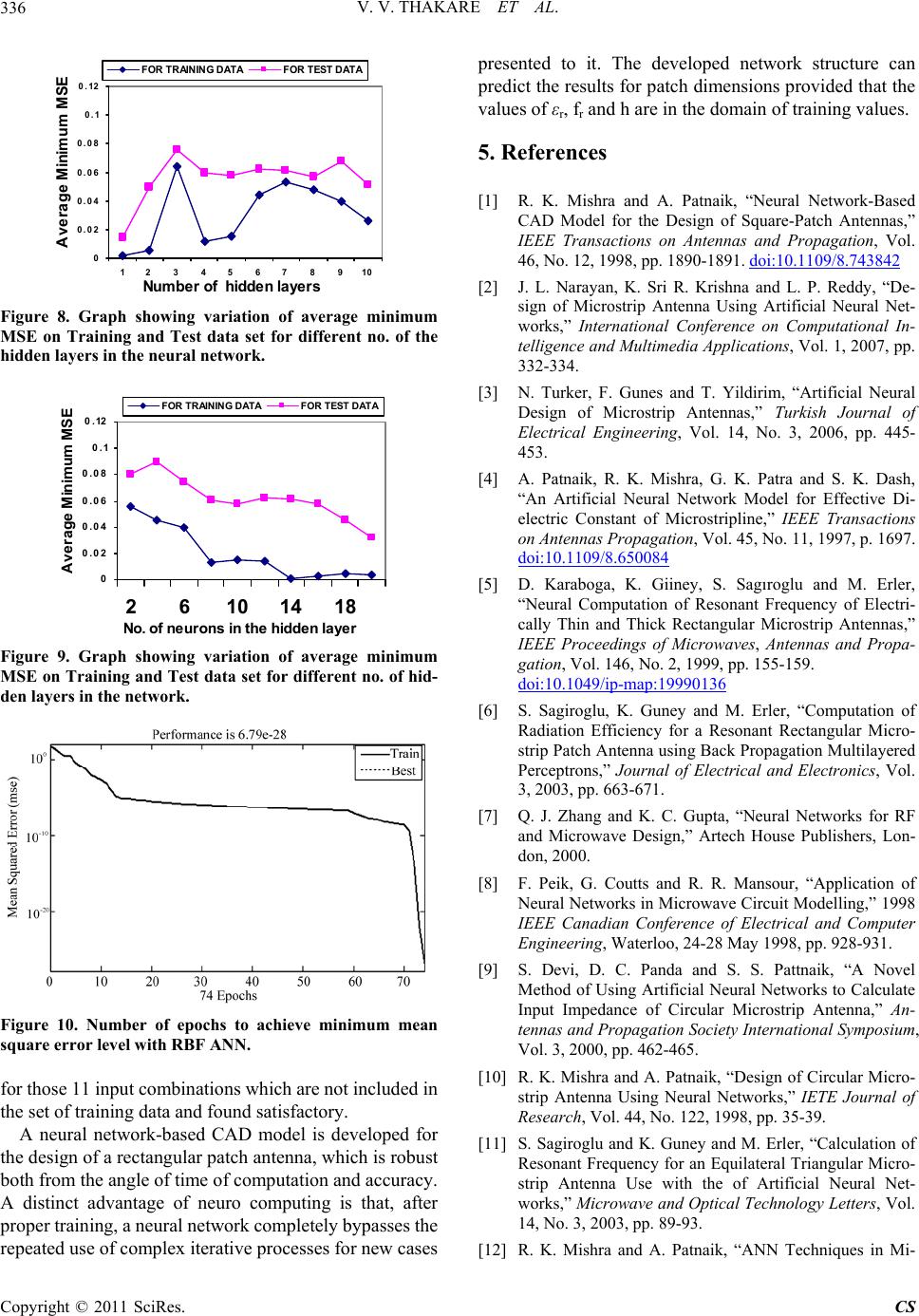
V. V. THAKARE ET AL.
336
0
0.02
0.04
0.06
0.08
0.1
0.12
12345678910
Number of hidden la
ers
Average Minimum MSE
FOR TRAINING DATAFOR TEST DATA
Figure 8. Graph showing variation of average minimum
MSE on Training and Test data set for different no. of the
hidden layers in the neural network.
0
0.02
0.04
0.06
0.08
0.1
0.12
2610 1418
No. of neurons in the hidden la
er
Averag e M inimum MSE
FOR T RAI NING DAT AFOR TEST DATA
Figure 9. Graph showing variation of average minimum
MSE on Training and Test data set for different no. of hid-
den layers in the networ k.
Figure 10. Number of epochs to achieve minimum mean
square error level with RBF ANN.
for those 11 input combinations which are not included in
the set of training data and found satisfactory.
A neural network-based CAD model is developed for
the design of a rectangular patch antenna, which is robust
both from the angle of time of computation and accuracy.
A distinct advantage of neuro computing is that, after
proper training, a neural network completely bypasses the
repeated use of complex iterative processes for new cases
presented to it. The developed network structure can
predict the results for patch dimensions provided that the
values of εr, fr and h are in the domain of training values.
5. References
[1] R. K. Mishra and A. Patnaik, “Neural Network-Based
CAD Model for the Design of Square-Patch Antennas,”
IEEE Transactions on Antennas and Propagation, Vol.
46, No. 12, 1998, pp. 1890-1891. doi:10.1109/8.743842
[2] J. L. Narayan, K. Sri R. Krishna and L. P. Reddy, “De-
sign of Microstrip Antenna Using Artificial Neural Net-
works,” International Conference on Computational In-
telligence and Multimedia Applications, Vol. 1, 2007, pp.
332-334.
[3] N. Turker, F. Gunes and T. Yildirim, “Artificial Neural
Design of Microstrip Antennas,” Turkish Journal of
Electrical Engineering, Vol. 14, No. 3, 2006, pp. 445-
453.
[4] A. Patnaik, R. K. Mishra, G. K. Patra and S. K. Dash,
“An Artificial Neural Network Model for Effective Di-
electric Constant of Microstripline,” IEEE Transactions
on Antennas Propagation, Vol. 45, No. 11, 1997, p. 1697.
doi:10.1109/8.650084
[5] D. Karaboga, K. Giiney, S. Sagıroglu and M. Erler,
“Neural Computation of Resonant Frequency of Electri-
cally Thin and Thick Rectangular Microstrip Antennas,”
IEEE Proceedings of Microwaves, Antennas and Propa-
gation, Vol. 146, No. 2, 1999, pp. 155-159.
doi:10.1049/ip-map:19990136
[6] S. Sagiroglu, K. Guney and M. Erler, “Computation of
Radiation Efficiency for a Resonant Rectangular Micro-
strip Patch Antenna using Back Propagation Multilayered
Perceptrons,” Journal of Electrical and Electronics, Vol.
3, 2003, pp. 663-671.
[7] Q. J. Zhang and K. C. Gupta, “Neural Networks for RF
and Microwave Design,” Artech House Publishers, Lon-
don, 2000.
[8] F. Peik, G. Coutts and R. R. Mansour, “Application of
Neural Networks in Microwave Circuit Modelling,” 1998
IEEE Canadian Conference of Electrical and Computer
Engineering, Waterloo, 24-28 May 1998, pp. 928-931.
[9] S. Devi, D. C. Panda and S. S. Pattnaik, “A Novel
Method of Using Artificial Neural Networks to Calculate
Input Impedance of Circular Microstrip Antenna,” An-
tennas and Propagation Society International Symposium,
Vol. 3, 2000, pp. 462-465.
[10] R. K. Mishra and A. Patnaik, “Design of Circular Micro-
strip Antenna Using Neural Networks,” IETE Journal of
Research, Vol. 44, No. 122, 1998, pp. 35-39.
[11] S. Sagiroglu and K. Guney and M. Erler, “Calculation of
Resonant Frequency for an Equilateral Triangular Micro-
strip Antenna Use with the of Artificial Neural Net-
works,” Microwave and Optical Technology Letters, Vol.
14, No. 3, 2003, pp. 89-93.
[12] R. K. Mishra and A. Patnaik, “ANN Techniques in Mi-
Copyright © 2011 SciRes. CS