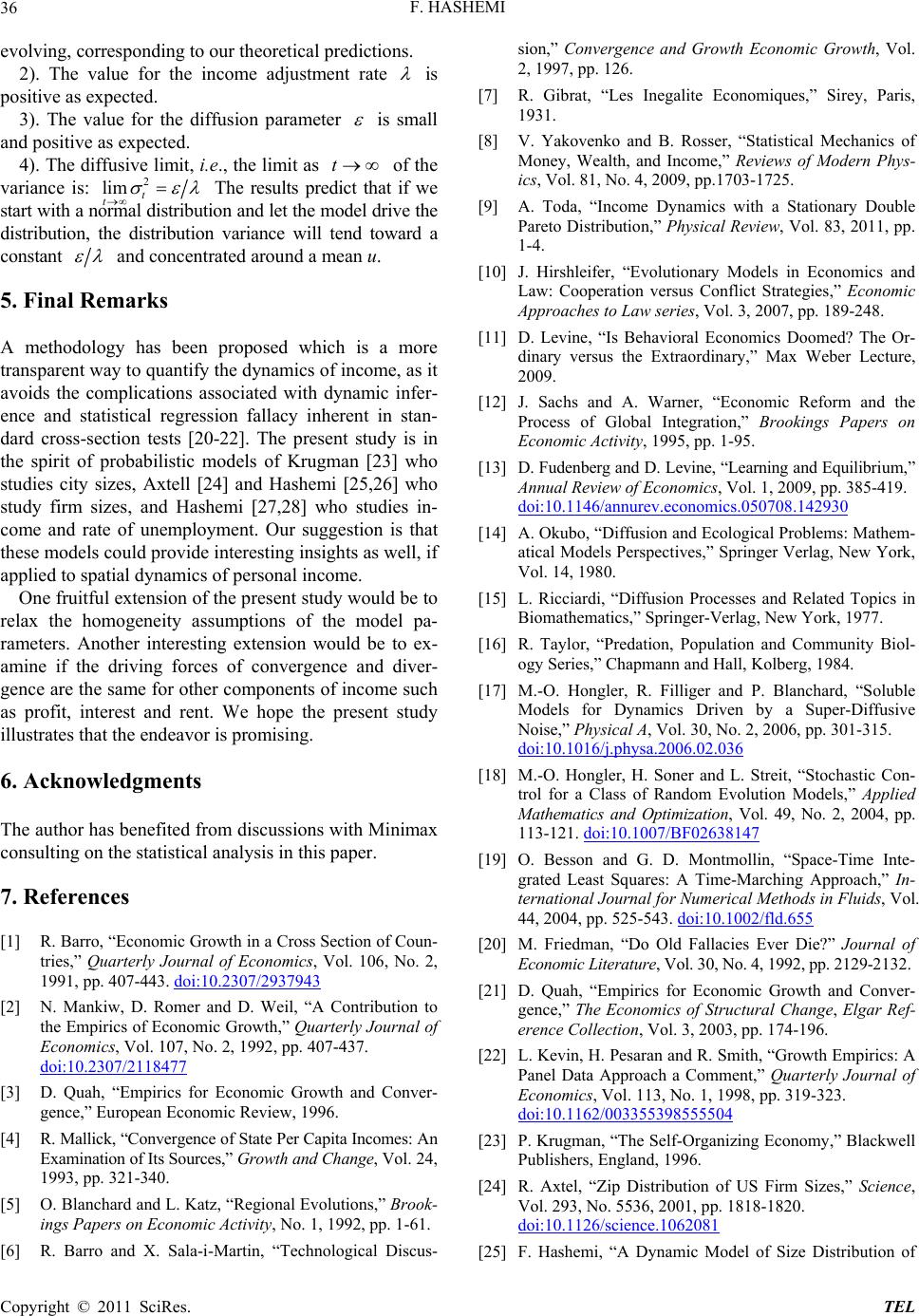
F. HASHEMI
36
evolving, corresponding to our theoretical predictions.
2). The value for the income adjustment rate
is
positive as expected.
3). The value for the diffusion parameter
is small
and positive as expected.
4). The diffusive limit, i.e., the limit as of the
variance is:
t
2
lim t
t
The results predict that if we
start with a normal distribution and let the model drive the
distribution, the distribution variance will tend toward a
constant
and concentrated around a mean u.
5. Final Remarks
A methodology has been proposed which is a more
transparent way to quantify the dynamics of income, as it
avoids the complications associated with dynamic infer-
ence and statistical regression fallacy inherent in stan-
dard cross-section tests [20-22]. The present study is in
the spirit of probabilistic models of Krugman [23] who
studies city sizes, Axtell [24] and Hashemi [25,26] who
study firm sizes, and Hashemi [27,28] who studies in-
come and rate of unemployment. Our suggestion is that
these models could provide interesting insights as well, if
applied to spatial dynamics of personal income.
One fruitful extension of the present study would be to
relax the homogeneity assumptions of the model pa-
rameters. Another interesting extension would be to ex-
amine if the driving forces of convergence and diver-
gence are the same for other components of income such
as profit, interest and rent. We hope the present study
illustrates that the endeavor is promising.
6. Acknowledgments
The author has benefited from discussions with Minimax
consulting on the statistical analysis in this paper.
7. References
[1] R. Barro, “Economic Growth in a Cross Section of Coun-
tries,” Quarterly Journal of Economics, Vol. 106, No. 2,
1991, pp. 407-443. doi:10.2307/2937943
[2] N. Mankiw, D. Romer and D. Weil, “A Contribution to
the Empirics of Economic Growth,” Quarterly Journal of
Economics, Vol. 107, No. 2, 1992, pp. 407-437.
doi:10.2307/2118477
[3] D. Quah, “Empirics for Economic Growth and Conver-
gence,” European Economic Review, 1996.
[4] R. Mallick, “Convergence of State Per Capita Incomes: An
Examination of Its Sources,” Growth and Change, Vol. 24,
1993, pp. 321-340.
[5] O. Blanchard and L. Katz, “Regional Evolutions,” Brook-
ings Papers on Economic Activity, No. 1, 1992, pp. 1-61.
[6] R. Barro and X. Sala-i-Martin, “Technological Discus-
sion,” Convergence and Growth Economic Growth, Vol.
2, 1997, pp. 126.
[7] R. Gibrat, “Les Inegalite Economiques,” Sirey, Paris,
1931.
[8] V. Yakovenko and B. Rosser, “Statistical Mechanics of
Money, Wealth, and Income,” Reviews of Modern Phys-
ics, Vol. 81, No. 4, 2009, pp.1703-1725.
[9] A. Toda, “Income Dynamics with a Stationary Double
Pareto Distribution,” Physical Review, Vol. 83, 2011, pp.
1-4.
[10] J. Hirshleifer, “Evolutionary Models in Economics and
Law: Cooperation versus Conflict Strategies,” Economic
Approaches to Law series, Vol. 3, 2007, pp. 189-248.
[11] D. Levine, “Is Behavioral Economics Doomed? The Or-
dinary versus the Extraordinary,” Max Weber Lecture,
2009.
[12] J. Sachs and A. Warner, “Economic Reform and the
Process of Global Integration,” Brookings Papers on
Economic Activity, 1995, pp. 1-95.
[13] D. Fudenberg and D. Levine, “Learning and Equilibrium,”
Annual Review of Economics, Vol. 1, 2009, pp. 385-419.
doi:10.1146/annurev.economics.050708.142930
[14] A. Okubo, “Diffusion and Ecological Problems: Mathem-
atical Models Perspectives,” Springer Verlag, New York,
Vol. 14, 1980.
[15] L. Ricciardi, “Diffusion Processes and Related Topics in
Biomathematics,” Springer-Verlag, New York, 1977.
[16] R. Taylor, “Predation, Population and Community Biol-
ogy Series,” Chapmann and Hall, Kolberg, 1984.
[17] M.-O. Hongler, R. Filliger and P. Blanchard, “Soluble
Models for Dynamics Driven by a Super-Diffusive
Noise,” Physical A, Vol. 30, No. 2, 2006, pp. 301-315.
doi:10.1016/j.physa.2006.02.036
[18] M.-O. Hongler, H. Soner and L. Streit, “Stochastic Con-
trol for a Class of Random Evolution Models,” Applied
Mathematics and Optimization, Vol. 49, No. 2, 2004, pp.
113-121. doi:10.1007/BF02638147
[19] O. Besson and G. D. Montmollin, “Space-Time Inte-
grated Least Squares: A Time-Marching Approach,” In-
ternational Journal for Numerical Methods in Fluids, Vol.
44, 2004, pp. 525-543. doi:10.1002/fld.655
[20] M. Friedman, “Do Old Fallacies Ever Die?” Journal of
Economic Literature, Vol. 30, No. 4, 1992, pp. 2129-2132.
[21] D. Quah, “Empirics for Economic Growth and Conver-
gence,” The Economics of Structural Change, Elgar Ref-
erence Collection, Vol. 3, 2003, pp. 174-196.
[22] L. Kevin, H. Pesaran and R. Smith, “Growth Empirics: A
Panel Data Approach a Comment,” Quarterly Journal of
Economics, Vol. 113, No. 1, 1998, pp. 319-323.
doi:10.1162/003355398555504
[23] P. Krugman, “The Self-Organizing Economy,” Blackwell
Publishers, England, 1996.
[24] R. Axtel, “Zip Distribution of US Firm Sizes,” Science,
Vol. 293, No. 5536, 2001, pp. 1818-1820.
doi:10.1126/science.1062081
[25] F. Hashemi, “A Dynamic Model of Size Distribution of
Copyright © 2011 SciRes. TEL