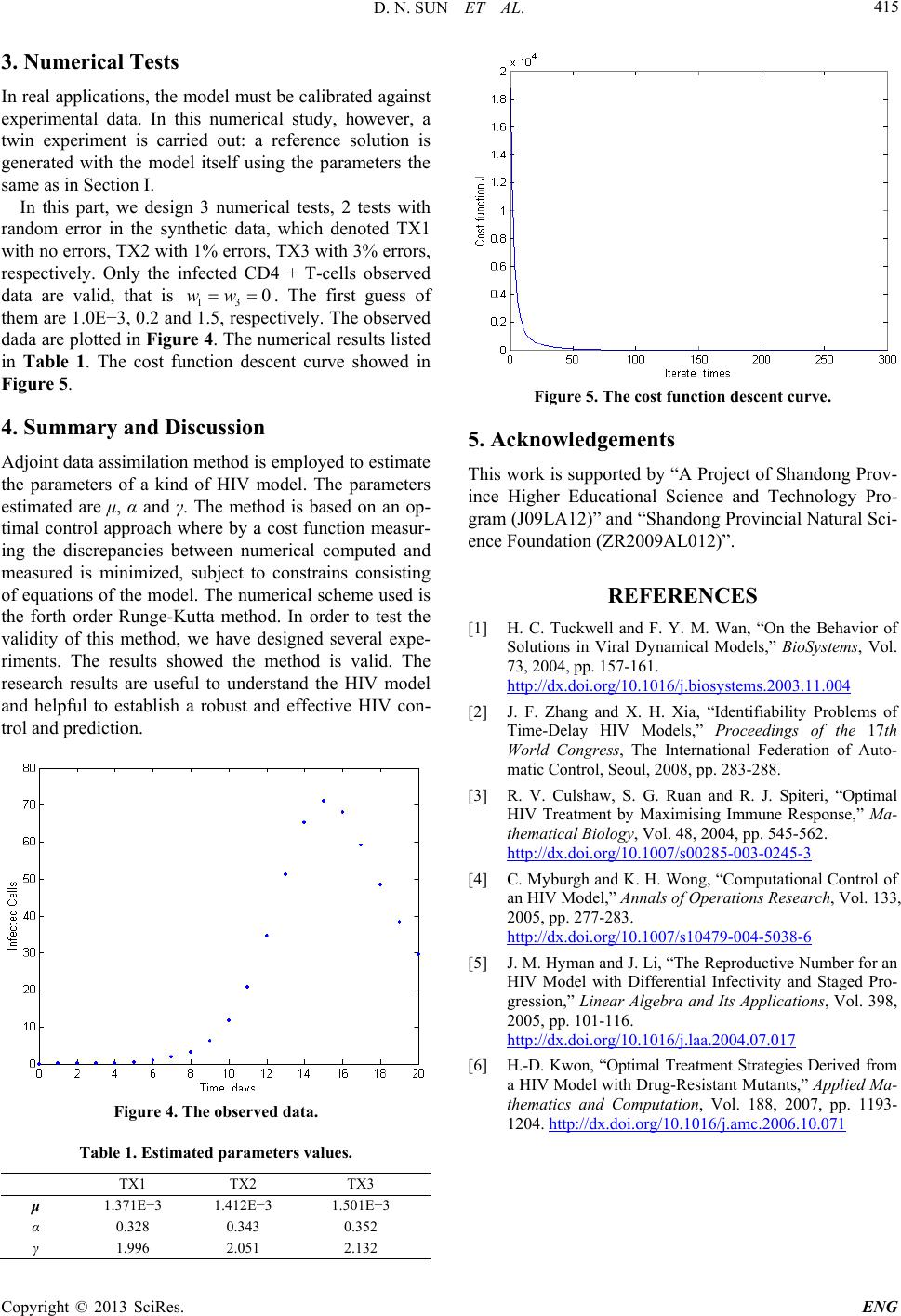
D. N. SUN ET AL.
Copyright © 2013 SciRes. ENG
415
3. Numerical Tests
In real applications, the model must be calibrated against
experimental data. In this numerical study, however, a
twin experiment is carried out: a reference solution is
generated with the model itself using the parameters the
same as in Section I.
In this part, we design 3 numerical tests, 2 tests with
random error in the synthetic data, which denoted TX1
with no errors, TX2 with 1% errors, TX3 with 3% errors,
respectively. Only the infected CD4 + T-cells observed
data are valid, that is
. The first guess of
them are 1.0E−3, 0.2 and 1.5, respectively. The observed
dada are plotted in Figure 4. Th e numerical resu lts listed
in Table 1. The cost function descent curve showed in
Figure 5.
4. Summary and Discussion
Adjoint data assimilation method is employed to estimate
the parameters of a kind of HIV model. The parameters
estimated are μ, α and γ. The method is based on an op-
timal control approach where by a cost function measur-
ing the discrepancies between numerical computed and
measured is minimized, subject to constrains consisting
of equations of the model. The numerical scheme used is
the forth order Runge-Kutta method. In order to test the
validity of this method, we have designed several expe-
riments. The results showed the method is valid. The
research results are useful to understand the HIV model
and helpful to establish a robust and effective HIV con-
trol and prediction.
Figure 4. The observed data.
Table 1. Estimated parameters values.
TX1 TX2 TX3
μ 1.371E−3 1.412E−3 1.501E−3
α 0.328 0.343 0.352
γ 1.996 2.051 2.132
Figure 5. The cost f unction desc ent curve.
5. Acknowledgements
This work is supported by “A Project of Shandong Prov-
ince Higher Educational Science and Technology Pro-
gram (J09LA12)” and “Shandong Provincial Natural Sci-
ence Foundation (ZR2009AL012)”.
REFERENCES
[1] H. C. Tuckwell and F. Y. M. Wan, “On the Behavior of
Solutions in Viral Dynamical Models,” BioSystems, Vol.
73, 2004, pp. 157-161.
http://dx.doi.org/10.1016/j.biosystems.2003.11.004
[2] J. F. Zhang and X. H. Xia, “Identifiability Problems of
Time-Delay HIV Models,” Proceedings of the 17th
World Congress, The International Federation of Auto-
matic Control, Seoul, 2008, pp. 283-288.
[3] R. V. Culshaw, S. G. Ruan and R. J. Spiteri, “Optimal
HIV Treatment by Maximising Immune Response,” Ma-
thematical Biology, Vol. 48, 2004, pp. 545-562.
http://dx.doi.org/10.1007/s00285-003-0245-3
[4] C. Myburgh and K. H. Wong, “Computational Control of
an HIV Model,” Annals of Operations Research, Vol. 133,
2005, pp. 277-283.
http://dx.doi.org/10.1007/s10479-004-5038-6
[5] J. M. Hy ma n and J. Li, “The Reproductive Number for an
HIV Model with Differential Infectivity and Staged Pro-
gression,” Linear Algebra and Its Applications, Vol. 398,
2005, pp. 101-116.
http://dx.doi.org/10.1016/j.laa.2004.07.017
[6] H.-D. Kwon, “Optimal Treatment Strategies Derived from
a HI V Model with Drug-Resistant Mutants,” Applie d Ma-
thematics and Computation, Vol. 188, 2007, pp. 1193-
1204. http://dx.doi.org/10.1016/j.amc.2006.10.071