Characterization of Generalized Uniform Distribution through Expectation ()
1. Introduction
Normally the mass of a root has a uniform distribution. Plant develops into the reproductive phase of growth; a mat of smaller roots grows near the surface to a depth of approximately 1/6-th of maximum depth achieve (see G. Ooms and K. L. Moore [1] ). Dixit [2] studied the problem of efficient estimation of parameters of a uniform distribution in the presence of outliers. He assumed that a set of random variables
represents the masses of roots where out of n-random variables some of these roots (say k) have different masses; therefore, those masses have different uniform distributions with unknown parameters and these k observations are distributed with Generalize Uniform Distribution (GUD) with probability density function (pdf)
(1.1)
where
are known constants;
is positive absolutely continuous function and
is everywhere differentiable function. Since derivative of
being positive, range is truncated by
from right
.
Dixt [3] obtained Maximum Likelihood Estimator (MLE) and the Uniformly Minimum Variance (UMVU) estimator of reliability functional,
in the same setup and showed that the UMVUE is better than MLE when one parameter of GUD is known, where as both parameters of the GUD are unknown,
is estimated by using mixture estimate and is consistent.
In this paper the problem of characterization of GUD with pdf given in (1.1) has been studied and the characterization also holds for uniform distribution on interval
when
. Various approaches were used to characterize uniform distribution; few of them have used coefficient of correlation of smaller and the larger of a random sample of size two; Bartoszyn’ski [4] , Terreel [5] , Lopez-Bldzquez [6] as Kent [7] , have used independence of sample mean and variance; Lin [8] , Too [9] , Arnold [10] , Driscoll [11] , Shimizu [12] , and Abdelhamid [13] have used moment conditions, n-fold convolution modulo one and inequalities of Chernoff-type were also used (see Chow [14] and Sumrita [15] ).
In contrast to all above brief research background and application of characterization of member of Pearson family, this research does not provide unified approach to characterized generalized uniform.
The aim of the present research note is to give a path breaking new characterization for generalized uniform distribution through expectation of function of random variable,
using identity and equality of expectation of function of random variable. Characterization theorem was derived in Section 2 with method for characterization as remark and Section 3 devoted to applications for illustrative purpose including special case of uniform distribution.
2. Characterization
Theorem 2.1. Let
be a continuous random variable (rv) with distribution function
having pdf
. Assume that
is continuous on the interval
, where
. Let
be a differentiable functions of
on the interval
, where
and more over
be nonconstant. Then
is the pdf of Generalize Uniform Distribution (GUD) defined in (1.1) if and only if
(2.1)
Proof: Given
defined in (1.1), if
is such that
where
is differentiable function then
(2.2)
Differentiating with respect to
on both sides of (2.2) and replacing X for
after simplification one gets
(2.3)
which establishes necessity of (2.1). Conversely given (2.1), let
be the pdf of rv X such that
(2.4)
Since
, the following identity holds
(2.5)
Differentiating
with respect to x and simplifying after tacking
as one factor one gets (2.5) as
(2.6)
Substituting derivative of
in (2.6) it reduces to
(2.7)
where
is derived in (2.3) and by uniqueness theorem from (2.4) and (2.7)
(2.8)
Since
is decreasing function for
and
is satisfy only when range of X is truncated by
from right and integrating (2.8) on the interval
on both sides, one gets
derived in (2.8) as
(2.9)
and

Hence
derived in (2.9) reduces to
defined in (1.1) which establishes sufficiency of (2.1).
Remark 2.1. Using
derived in (2.3), the
given in (1.1) can be determined by
(2.10)
and pdf is given by
(2.11)
where
is increasing function for
with
such that it satisfies
(2.12)
Remark 2.2. If
characterization theorem 2.1 also holds for uniform distribution with pdf
(2.13)
3. Examples
Using method describe in remark 2.1 Generalize Uniform Distribution (GUD) through expectation of non-constant function of random variable such as mean,
raw moment,
,
,
quantile, distribution function, reliability function and hazard function is given to illustrate application and significant of unified approach of characterization result (2.1) of theorem 2.1.
Example 3.1. Characterization of Generalize Uniform Distribution (GUD) through hazard function
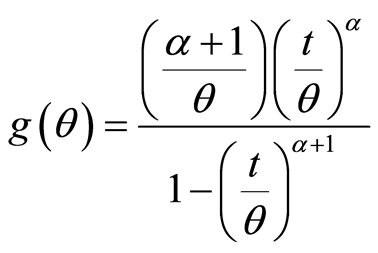
therefore
From (2.3) one gets
as
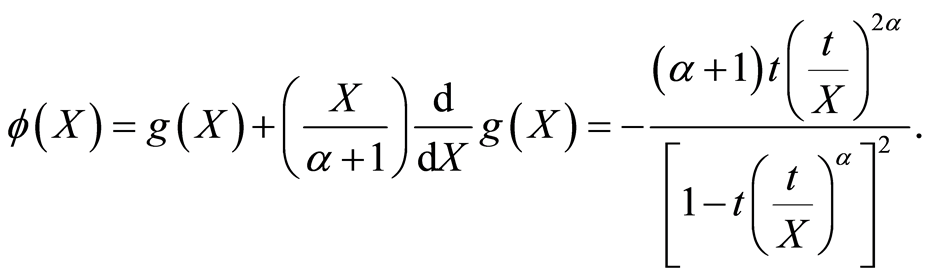
and using (2.10) of remark 2.1 the
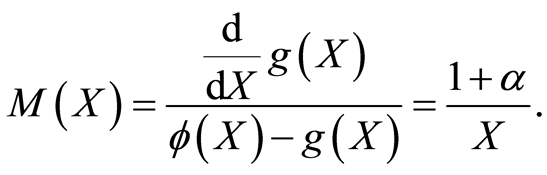
By characterization method describe in remark 2.1, if
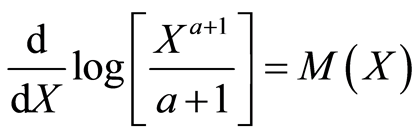
then

and substituting
as appeared in (2.12) for (2.11),

is characterized.
Example 3.2. The characterization of
defined in (2.1) through non constant function such as
(3.1)
and using
(3.2)
and defining
given in (2.10) and substituting
as appeared in (2.12) for (2.11),
is characterized.
Example 3.3. In context of remark 2.2 uniform distribution with pdf given in (2.13) characterized through
quantile

therefore
.
From (2.3) one gets
as

and using (2.10) of remark 2.1 the
.
By characterization method describe in remark 2.1, if

then
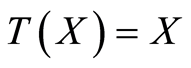

Example 3.3. The pdf
defined in (2.13) can be characterized through non-constant function such as
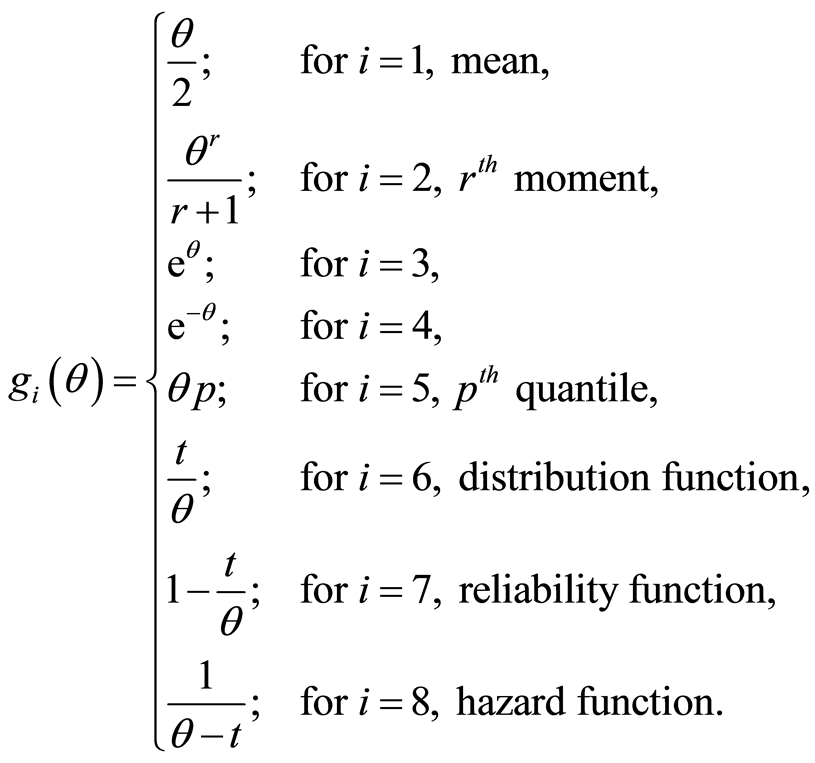
and using
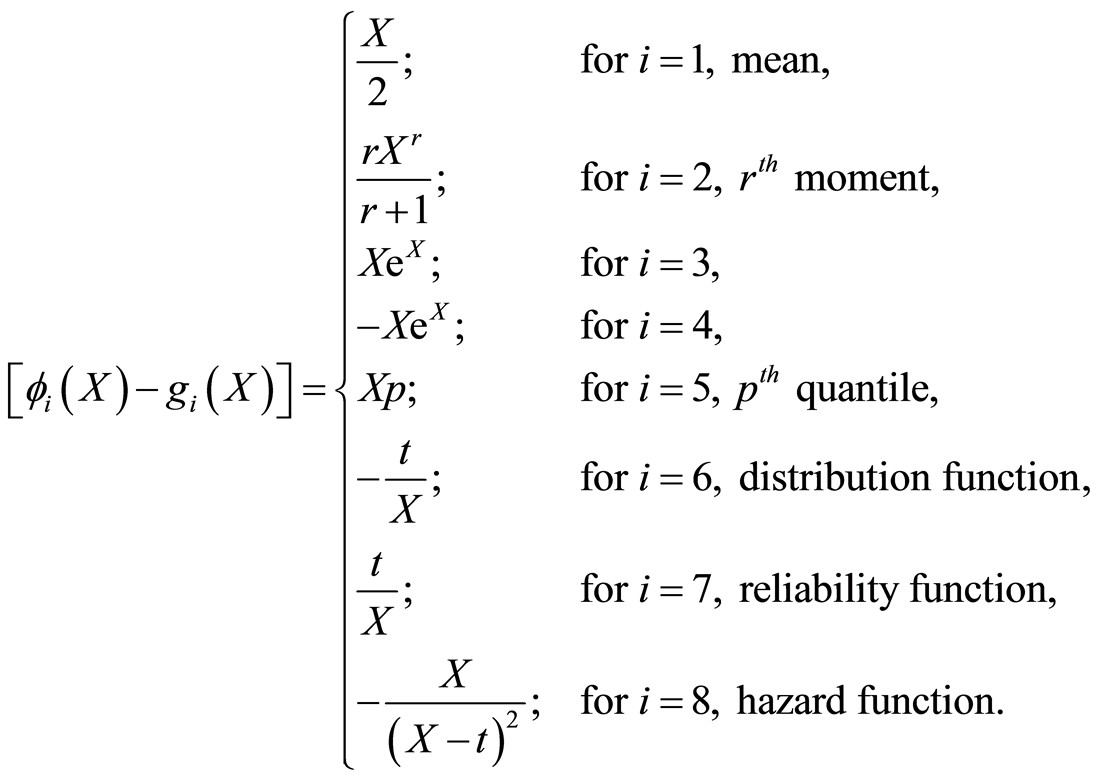
and defining
given in (2.10) and substituting
as appeared in (2.12) for (2.11),
is characterized.
4. Conclusion
To characterize pdf defined in (1.1) one needs any arbitrary non-constant function of X which should be differentiable and integrable only.
Acknowledgements
This work is supported by UGC Major Research Project No: F.No.42-39/2013 (SR), dated 12-3-2013.