Tail Dependence Study of SSE Composite Index and SZSE Component Index Based on the Copula ()
1. Introduction
With the continuous development of the financial markets, the relationship of interior financial markets is more and more complex, it will promote the study of dependence between financial market structure. In the quantitative analysis of related financial market structure, dependence study is very important, a series of financial problems involve the dependence studies, such as risk management, portfolio selection, asset pricing and so on. Using multivariate distribution function to depict the dependence between each component is the most common method in mathematics; However traditional multivariate distribution function exists some problems in practical application, when the analysis formulas of multivariate distribution function contain many variables, it will be difficult to handle, and it requires the type of marginal distribution function to fit the type of multivariate distribution function. In financial analysis, marginal distribution often do not have the same type of distribution, this makes the traditional multivariate distribution functions can not be widely used in the dependence analysis of financial markets. By Sklar theorem [1], using the copula function to structure flexible multivariate distribution function, so as to grasp the real dependence between financial markets. Therefore, in this paper we use copula functions to study the tail dependence between SSE composite index and SZSE component index.
At present, using copula function to study the dependence of the financial market already had many achievements, but also in many domestic and foreign literatures, most of them used parameter estimation method to estimate parameters of copula functions. The innovation of this paper is to use a parameter estimation method for parameter estimation, to determine copula functions, and then to do the test of tail dependence.
2. Tail Dependence Based on Copula
Tail dependence can be used to represent extreme events of the interaction between the variables, for example, when the random variable X increased or decreased significantly, the probability of the random variable Y increased or decreased significantly [2]. We use the tail dependence coefficient of copula to research the relationship between SSE composite index and SZSE component index. Let X and Y be continuous random variables with distribution functions F and G respectively, and whose copula is C, and then, based on the copula function we define the upper tail dependence coefficient
and the lower tail dependence coefficient
as follows [3]:
(1)
(2)
where
, when
, we think the upper (lower) tail of X and Y be asymptotic dependence; when
, we think the upper (lower) tail of X and Y be asymptotic independence; where
and
are the t quantile functions of distribution functions
and
respectively [4].
3. Selecting Copulas and Estimating Parameters
There are many kinds of copula functions, Elliptic copulas and Archimedean copulas are used commonly, and each group has many specific copula functions. Different copula functions have different properties, in the actual application, choosing the right copula functions need to follow two principles: one is that the established model of copulas should be easy to operate and understand, to avoid the phenomenon of unknown parameters [5]; second is to choose the appropriate copula functions which are suitable for the sample datas. Elliptical copula functions are with a symmetrical tail dependence, this will be conflicted with fat-tailed distribution of financial datas. Archimedean copulas are the most widely used in the financial field, and it is easy to build and calculate them. The bivariate normal copula with symmetry and asymptotic tail independence is applied widely, but it is unable to capture the asymmetric dependence and tail dependence between the variables. Here we select copulas which have obvious tail features. Because the log return correlation is accorded with the Archimedean copula distribution, so we choose the Clayton copula with obvious lower tail features, the Gumbel copulas with obvious upper tail features, and the A12 copula with both upper and lower tail features from Archimedean copulas on the financial data fitting [6]. Moreover Archimedean copulas have a feature that Kendall’s tau is the analytic function of
. By Sklar theorem, when marginal distribution function is continuous, the copulas function will be uniquely identified.
For the parameter estimation of a specific copula function, parametric approach and nonparametric approach are common methods: 1) strict maximum likelihood approach (EML) and marginal distribution extrapolation approach (IFM) are more frequently used in the parameter method. Maximum likelihood approach estimates marginal distribution and parameters of copula function at the same time; Marginal distribution extrapolation approach divide estimation process into two steps, first to estimate the parameters of the marginal distribution function, and then estimate the parameters of copula function. 2) Genest and Rivest approachs are commonly used in the nonparametric approach [7]. As we know, for a copula function
, it is related to the Kendall’s tau as following:
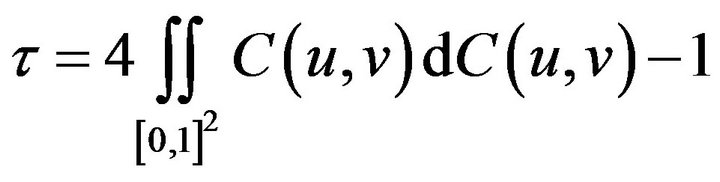
So for most of the single parameter Archimedean copula functions, because of its generating element
is a function of the parameter
and the relationship between
and the Kendall’s tau as follows:
(3)
By solving the formula above, we can get the estimate value of
. The deficiency of EML and IFM approaches lie in the parameter estimation of copula function is affected by the marginal distribution function, if the assumptive model of marginal distribution function were wrong, it will lead to a biased estimate to the copula function. Therefore, in this article we use nonparametric estimation method in view of the features of the Archimedean copulas, to calculate the estimate value of
by the estimate value of the Kendall’s tau, further to estimate the parameters of copula functions.
3.1. The Introduction of Three Types of Copulas
1) Gumbel copula
(4)
Gumbel copula function is very sensitive to the change of variables at the upper tail of the distribution, so it can quickly capture the changes of upper tail dependence, and it can be used to represent the relations between financial variables which have upper tail dependence. Its parameter
represent the degree of correlation, when
, the variables are independent; when
, the variables are completely related. By formula (1) and (4), we can get the upper tail dependence coefficient of Gumbel copula function, namely
, by its generating element
and formula (3), the Kendall’s tau is the analytic function of
:

Then,
, accordingly,
(5)
In addition, by the formula

We can get
under different levels of
.
2) Clayton copula
(6)
Clayton Copula function is very sensitive to the change of variables at the lower tail of the distribution, so it can quickly capture the changes of lower tail dependence, and it can be used to represent the relations between financial variables which have lower tail dependence. Its parameter
also represent the degree of correlation, when
, the variables are independent; when
, the variables are completely related. By formula (2) and (6), we can get the lower tail dependence coefficient of Clayton copula function, namely
, by its generating element
and formula (3), the Kendall’s tau is the analytic function of
:

Then,
, accordingly,
(7)
In addition, by the formula

We can get
under different levels of
.
3) A12 copula of Archimedean copulas
(8)
Gumbel copula function has only the feature of the upper tail dependence, and Clayton copula function has only the feature of the lower tail dependence, while the A12 copula of Archimedean copulas has both upper tail and lower tail dependence, by formula (1) and (8), the upper tail dependence coefficient can be expressed with the formula
, and by formula (2) and (8), the lower tail dependence coefficient can be expressed with the formula
, then by its generating element
and formula (3), the Kendall’s tau is the analytic function of
:

Then,
, accordingly,
(9)
3.2. Estimating
with Nonparametric Estimation
In order to estimate the parameters, firstly we need to know the estimated value of the Kendall’s tau, originally the Kendall’s tau was not defined by the copula function
, it was defined by the consistent probability of the random variable
minus the non-uniform probability of the random variable
, that is

where
and
are independent identically distributed samples of the random variable
.
If
are independent identically distributed samples which come from joint distribution function
of random variable
and its copula function is
are the ranks of these samples,
represent the rank of
;
represent the rank of
; then the Kendall’s tau for the sample is defined as
(10)
So we can work out
on the basis of
, and the copula function can be determined solely; then according to the next two formulas to calculate the estimated values of the upper tail dependence coefficient and the lower tail dependence coefficient.
(11)
(12)
4. Computing Steps and Results
4.1. Calculating the Parameter 
We select day’s closing price
, of SSE composite index and SZSE component index from January 4, 2007 to March 21, 2011. Logarithmic yield
.
We define
, and by formula (10), we can get
. Then by formula (5), formula (7) and formula (9) respectively; we get estimated values of parameters of different copulas in Table 1.
From Table 1, the parameters of three kinds of copulas are in their respective domains, this explain that there exist both upper tail dependence and lower tail dependence between SSE composite index and SZSE component index. Because the A12 copula of Archimedean copulas has both upper tail and lower tail dependence, we choose A12 copula to study for tail dependence, then we should do the test for the selected copula functions.
4.2. Testing for Copulas
In order to further illustrate A12 copula function can describe the tail dependence between SSE composite index and SZSE component index, we do test for the datas. In order to avoid the fault of hypothetical marginal distribution of copula function, we estimate parameters with the Kendall’s tau directly, so we don’t know the marginal distribution function. We use the empirical distribution and
to structure and test variables which obey uniform distribution on inspection. Firstly we use geometric method to test copula distribution, then use K-S statistics to do the test of goodness of fit.
Univariate distribution function
is defined as
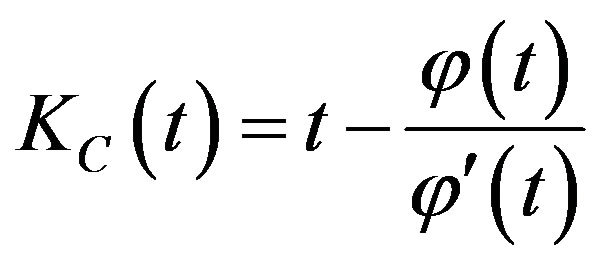
where,
is the generating element of copula functions [8],
obeys the standard uniform distribution. Setting variables X and Y to obey the empirical distribution, then we can wok out the
by means of
.
For A12 copula function,
(13)
Follow the steps to calculate1) To solve the distribution function
and
of logarithm yield
(both F and G are empirical distribution function);
2) To solve
by formula (8);
3) Putting the
which was calculated in step 2) into formula (13);
4)
, to do test with a Q-Q figure of the uniform distribution.
So we use these steps to get the Q-Q test which is shown in Figure 1.
In Figure 1, the
of A12 copula distribute uniformly on the oblique diagonal line and two sides, so it obey uniform distribution; Table 2 can also explain the
of A12 copula obeys uniform distribution. Therefore, we think that the A12 copula function is appropriate for describing the relationship between SSE composite index and SZSE component index, namely, A12 copula function is feasible to study the tail dependence of SSE composite index and SZSE component index.
4.3. Studying Tail Dependence
By formula (11) and formula (12), we can work out the upper tail dependence coefficient and the lower tail dependence coefficient, the results are shown in Table 3.

Figure 1. Q-Q figure of KC function of A12 copula.

Table 1. Parameter estimation of copulas.
The Table 3 shows that the upper tail dependence coefficient is 0.7343, the lower tail dependence coefficient is 0.7901. It indicates that there exists both upper tail dependence and lower tail dependence between SSE composite index and SZSE component index, what’s more, the upper tail dependence is less than the lower tail dependence. The tail dependence between logarithmic yield of SSE composite index and logarithmic yield of SZSE component index demonstrated the consistency of both two extremums; it also demonstrated that whether the price rise or fall, there would be different degrees of dependence between two indices.
5. Conclusion
We select Gumbel copula, Clayton copula and A12 copula from Archimedean copulas, all of which have features of tail dependence. We use the nonparametric estimation approach to estimate parameters of copula functions, and find that there exists both upper tail dependence and lower tail dependence between SSE composite index and SZSE component index, therefore, we select A12 copula to do the empirical analysis about the tail dependence between SSE composite index and SZSE component index. The results show that the upper tail dependence coefficient is less than the lower tail dependence coefficient between SSE composite index and SZSE component index, that is to say, the dependence between SSE composite index and SZSE component index during the downturn of the stock market is higher than the active period, which is consistent with the real financial market rule [9].
NOTES