1. Introduction
Urn models have been among the most popular probabilistic schemes and have received a lot of attention in the literature (see [1,2]). Let us describe the Pólya urn scheme briefly. In 1923, [3] proposed the following urn scheme to model processes such as the spread of infectious diseases. In this scheme, a single urn contains
white balls and
black balls. One ball is drawn at random and then replaced, together with
balls of the same color. The procedure is repeated n times. It is known that the sequence of the proportions of white balls is a martingale converging almost surely to a random variable having a beta distribution with parameters
and
. Since then, numerous generalizations and extentions of the Pólya urn have been studied : see [4-14]. In 1990, [6] generalized the Pólya urn model with the single change that the number of extra balls added in the urn is a function of time. One ball is drawn and is replaced in the urn along with F(n) balls of the same color. In his setup
can be any function. He showed that the proportions of white balls converge almost surely and the limit has no atom except possibly at 0 or 1.
In this paper, we propose a new class of discrete time stochastic processes generated by a two-color generalized Pólya urn that is reinforced every time. This is a generalization of the urn model considered in [10]. A single urn contains
white balls and
black balls: at discrete times
we draw
balls and note their colors, say
are black and
are white. We return the drawn balls to the urn. Moreover,
new white balls and
new black balls are added in the urn. The numbers
and
are random variables in
. We show that the proportions of white balls form a bounded martingale sequence which converges almost surely. Necessary and sufficient conditions for the limit to have no atoms at 0 or 1 are given.
This paper is organized as follows. In Section 2, we set the probabilistic model for a randomly reinforced urn. In Section 3, we show that the proportions of white balls form a bounded martingale sequence which converges almost surely. Necessary and sufficient conditions for the limit to concentrate on the set
are given. We conclude the paper by proving that interacting reinforced urn process are asymptotically exchangeable.
2. Model Description and Notation
On a rich enough probability space
, define two sequences
and
of positive, integervalued random variables and let
and
are fixed positive integers. A randomly reinforced urn generates the stochastic processes
. The sequences
and
respectively denote the number of black balls and white balls in the urn at time
. The dynamics of the processes
and
are governed by the following: set
and let
be a
random variable. Now we iterate this sampling scheme forever. Thus, at time
, given the sigma-field
generated by
, we consider that
is a Hypergeometric
random variable and we assume that
and
are independent conditionaly on
. Finally, we set
(2.1)
This is a generalization of the reinforced urn model considered in [10]. Assume that, at each time periode
a new firm appears on the market and have to choose operative systems among the systems A and B, for its
computers. The firm can choose
blocks of size
of computers having the operative system
. This choice depends on the number of computers having the operative system A on the market. The process
given in (2.1) is going to describe the evolution along time of the proportion of computers operating systems A.
3. Martingale Property
The process
is of primary interest for studying the stochastic processes generated by this generalization of Pólya’s urn. Here
represents the proportion of white balls in the urn at time
. We show that these proportions form a bounded martingale. This martingale converges almost surely. The next theorem is of fundamental importance, this is our main result.
Theorem 3.1. The sequence
is a bounded martingale with respect to the filtration
taking values in
Therefore, it converges almost surely to a random variable 
Proof.
Here
with
being the total number of balls in the urn at time
. Now let us show that
is a martingale. In fact

This shows that
is
-bounded martingale with respect to the filtration
. Hence, there exists a random variable
such that
on a set of probability one.
The exact distribution of
is unknown except in a few particular cases. The situation where
almost surely for all
and
almost surely for all n corresponds to the classical Pólya-Eggenberger contagious urn scheme. In this case
has a beta distribution with parameters
and
. Let us continue with the general case.
Theorem 3.2. The limit
is Bernoulli distributed if and only if
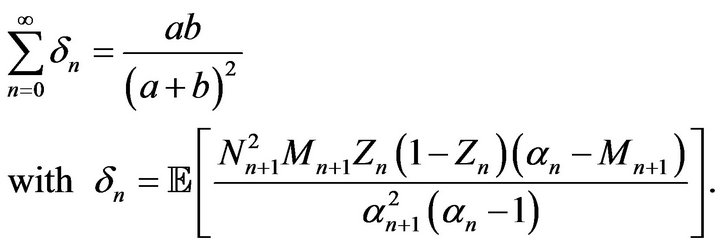
Proof. From (2.1) we have

thus we get
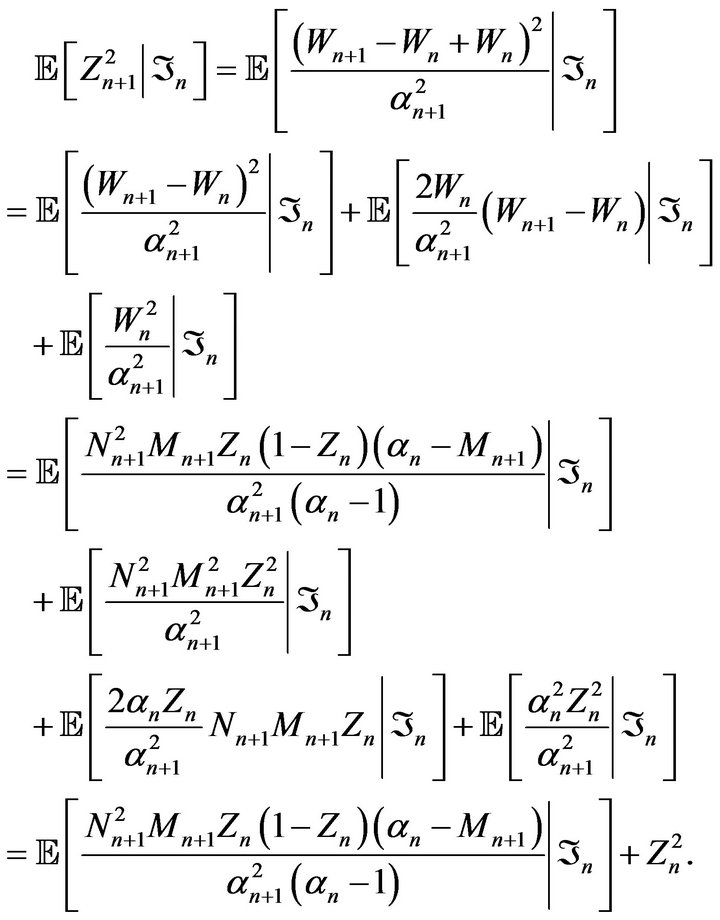
The transition between the second equality and the third equality relies on the fact that, conditionally on
are independent. As a result
, (3.1)
with 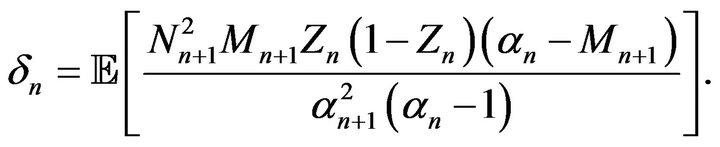
Now we set
, and we have from (3.1)

Consequently,
converges to
if and only if Vn converges to
, which happens whenever
. This is the desired result because a random variable
satisfies the condition
if and only if
is concentrated on the set
.
This theorem applies, for example, when
and
almost surely. In fact from (3.1), we remark that when
and
almost surely (
and
be any function) we have

and we deduce
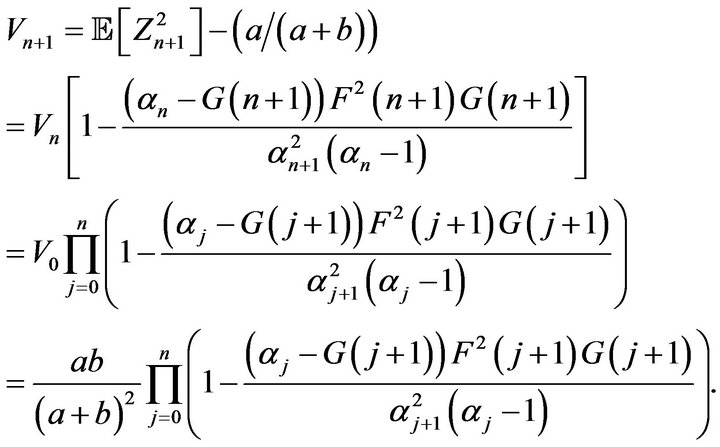
Consequently,
converges to
if and only if
converges to
, which happens whenever the product values
converges to
. This happens whenever
diverges.
Moreover, if
and
then

the general term of the series being proportional to
.
From Theorem 3.1 we deduce thatCorollary 3.3. Assume that the sequence
satisfies the following conditions

then
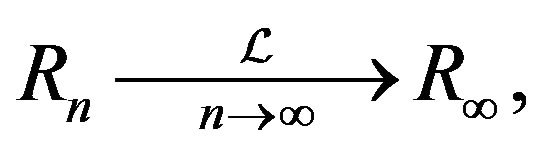
and

Proof. For every fixed
and for sufficiently large
,
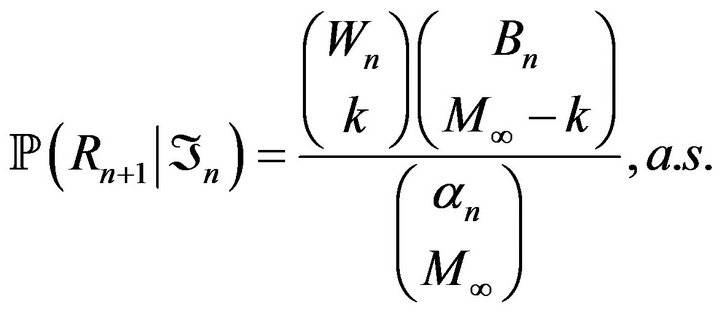
Then, using the well know convergence of the hypergeometric probabilities to the binomial, we have

and the conclusion follows from the bounded convergence theorem.
As a consequence of Theorem (3.2), we have the following.
Corollary 3.4. Assume that the sequences
and
are bounded then 
Proof. Since the sequences
and
are bounded by some constant
and for all
we obtain from (3.1) that
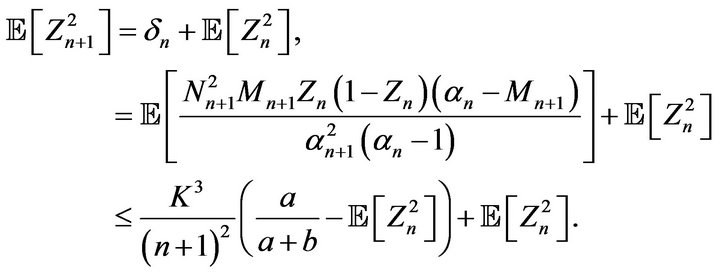
Let
be such that
. We obtain that

Now, since
, then
and 
so 
The final result in this section shows that the law of large numbers holds for interacting reinforced urn systems.
Theorem 3.5. We have
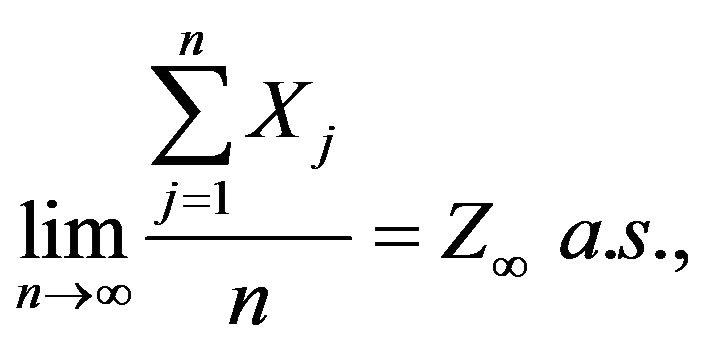
with 
Proof. Using Cesàro’s summability result we obtain that,

Let
. Since
then we have the following1)
, for all
.
2) For all
we have

This shows that the sequence
is orthogonal, with zero mean.
3) For
, we have

Therefore, the sequence
satisfies the conditions of Hall’s theorem (see [15], Theorem 2.8, p. 22), and we can conclude that the series
(3.2)
Finally, Kronecker’s lemma yields
(3.3)
This shows that
(3.4)
which is the announced result.
4. Asymptotic Exchangeability
According to [16] a sequence of random variables
is asymptotically exchangeable if the joint distribution of the sequence
converges as
to the distribution of some exchangeable sequence
. In particular, we have the following lemma due to [17].
Lemma 6. (Aldous)
Consider
be an infinite exchangeable sequence directed by
.
1) Let
be a regular conditional distribution for
given
.
Then
(4.1)
2) Let
be an infinite sequence. Let
be a regular conditinal distribution for
given
, and suppose that 
Then
(4.2)
Theorem 3.1 together with Lemma yield that the sequence of random variables
is asymptotically exchangeable. The main result of this section is the following.
Theorem 4.1. Under the conditions of Corollary 3.3, the sequence of random variables
is asymptotically exchangeable.
Proof. For all
, the conditional distribution of
given
, is such that

Hence, as in Corollary 3.3, we obtain that

The conclusion comes from Lemma 4.1, part (b).
5. Conclusions
Urn model have been widely studied and applied in both scientific and social science disciplines. In this paper, we have proposed a general class of discrete stochastic processes generated by a two-color generalized Pólya urn. This model generalizes a model previously studied by [10]. This paper also shows that the proportion of white balls form a bounded martingale sequence which converge almost surely. Asymptotic properties and asymptotic exchangeability are given. However, the complete characterization of the limit still remains to be resolved in the future and it would be interesting to explore the possibility extensions to more than two colors.
Another important application of this urn models is to randomize treatments to patients in a clinical trial (see [18]). Consider an urn containing balls of two type, representing two treatements. Patients normally arrive sequentially, and treatment assigned on the urn composition and previous treatment outcomes. For more details analysis on this application, we refer to [13].
6. Acknowledgements
The authors want to thank Professor Éric Marchand for his numerous advices and helpful discussions. The second author wants to thank NSERC for the financial support.
NOTES